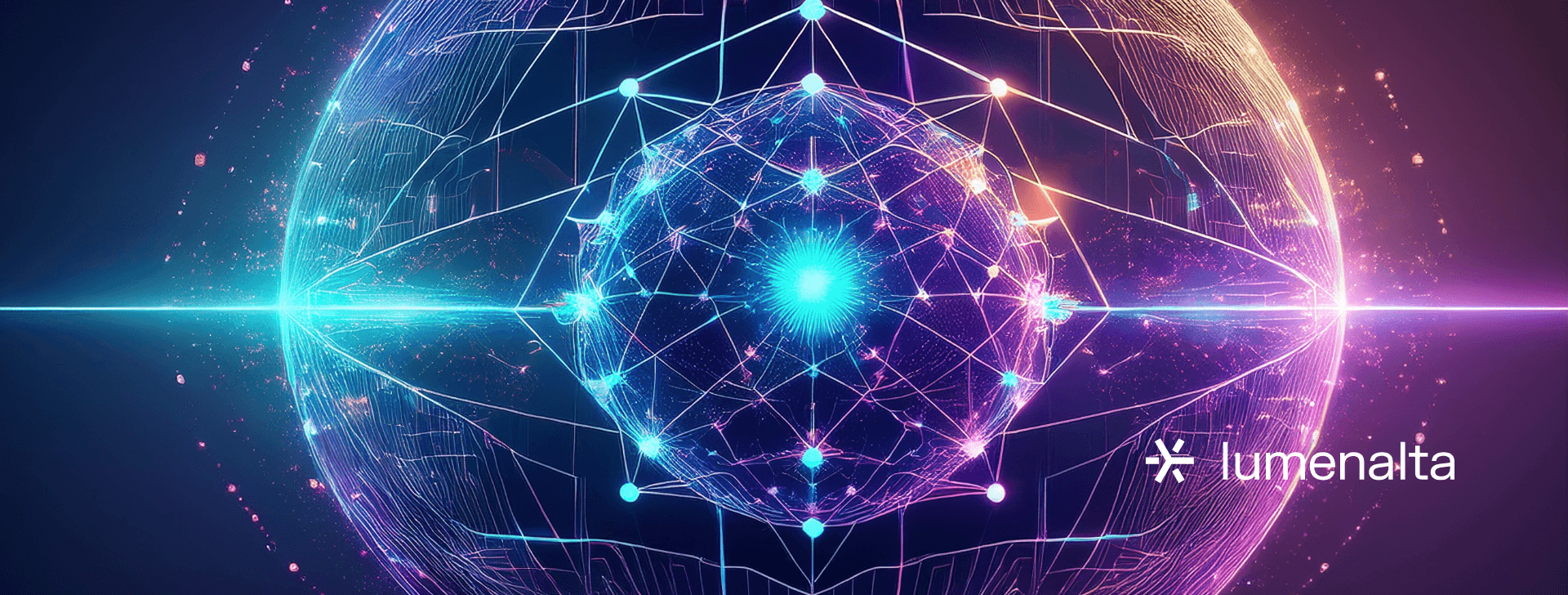
4 types of big data
MAR. 3, 2025
8 Min Read
Organizations handle unprecedented volumes of information that often arrive from multiple channels.
A powerful strategy is needed to extract insights from this influx and turn them into profitable outcomes. Many business leaders in logistics, financial services, and retail seek ways to refine these data-centered initiatives for better ROI. An effective place to start involves understanding the fundamental types of big data and how each category can shape results across the enterprise.
Resource allocation, cost-effectiveness, and fast deployment strategies are easier to optimize when you categorize data properly. Each type of data supports different analytics approaches and workflows, affecting how you plan projects and engage stakeholders. This classification also reveals new opportunities, such as monetizing information assets or modeling operational risks. Organizations that recognize these distinctions position themselves to expand faster and yield higher returns on their investments.
key-takeaways
- 1. Big data is defined by large, complex datasets that need specialized processing approaches.
- 2. Four categories—structured, semi-structured, quasi-structured, and unstructured—require unique strategies.
- 3. Understanding these types of data in big data ensures correct storage, analytics, and governance choices.
- 4. Cross-functional collaboration and targeted pilot projects can accelerate positive outcomes.
- 5. A robust governance plan and careful technology selection help unlock value while managing compliance.
What is big data?
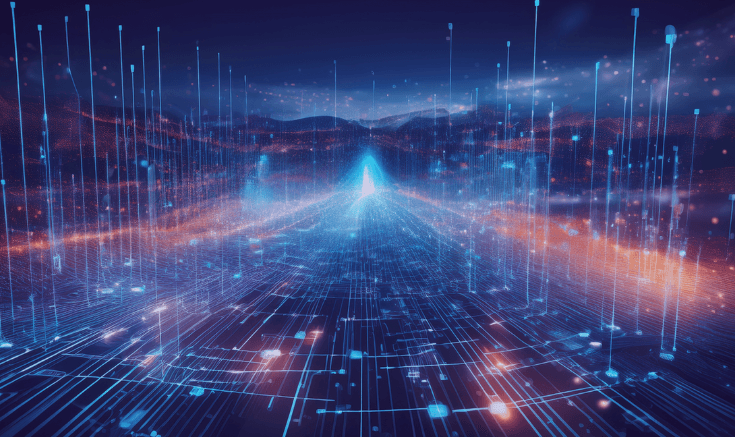
Big data refers to datasets that are too large or complex for conventional data processing methods. Examples range from social media interactions to sensor outputs from industrial equipment, all requiring specialized systems and expertise to process. These datasets move at high velocity, arrive in large volumes, and often lack consistency in structure, pushing teams to adopt modern frameworks for storage and analytics. When managed effectively, big data strengthens forecasts, accelerates product innovation, and reduces guesswork in strategic moves.
Enterprises often adopt specialized storage solutions, such as distributed file systems or data lakes, to handle these vast datasets. This infrastructure allows real-time processing or near-real-time analytics that keep pace with high-velocity data flow. Some organizations integrate big data with machine learning (ML) applications for personalized offers in retail or advanced risk modeling in financial services. The outcome is a more agile framework for turning complex streams of information into valuable insights that boost profitability.
"Resource allocation, cost-effectiveness, and fast deployment strategies are easier to optimize when you categorize data properly."
4 types of big data
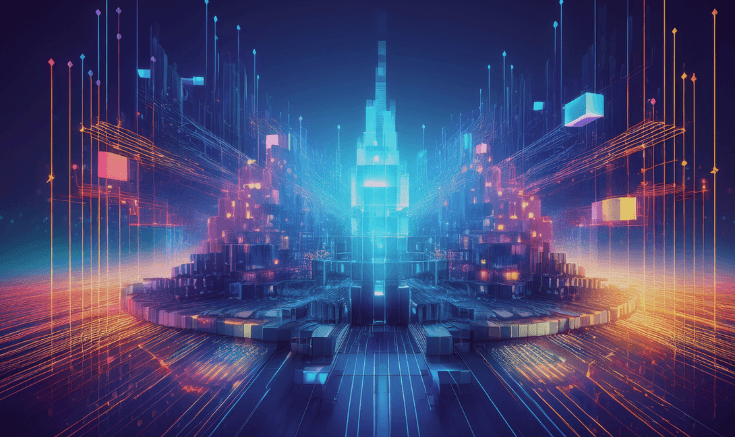
Organizations often categorize data based on format and how it can be processed. This practice is essential for designing the right data pipelines, and ensuring that speed, scalability, and security are not compromised. Each category has distinct needs for ingestion, storage, and retrieval, which impacts decisions around infrastructure and tooling. A closer look at the types of big data clarifies how each form can accelerate revenue growth and operational efficiency.
Defining categories is not just an academic exercise but a vital step in aligning analytics capabilities with real business outcomes. Structured data might serve compliance dashboards in financial services, while quasi-structured logs inform real-time user engagement tracking in e-commerce. Proper classification also simplifies data governance, as different security standards may apply to each type. This clarity paves the way for purposeful investments, eliminating guesswork and accelerating return on every data project.
Structured data
Structured data fits neatly into predefined tables or relationships, making it simpler to query using relational databases. This format suits situations where consistent field definitions and data schemas are critical, such as transaction records or user profiles. Many businesses in financial services rely on structured data to track revenue trends, manage compliance, and forecast potential outcomes. Although structured data usually offers high accuracy, it can be time-consuming to scale if your organization depends solely on rigid database frameworks.
Semi-structured data
Semi-structured data has some level of organization, but it does not strictly adhere to a formal structure like rows and columns. Formats such as JSON, XML, or email logs fall under this category, offering enough metadata to interpret elements but maintaining flexibility for different attributes. Teams handling sensor-generated JSON logs in logistics can adapt more quickly to changes in device outputs because the format is not locked into a rigid schema. This adaptability gives organizations a cost-effective way to manage data variety without extensive re-engineering of existing systems.
Quasi-structured data
Quasi-structured data lands between semi-structured and unstructured, often containing irregular patterns that require additional parsing to extract meaningful insights. Clickstream data is a classic example, where web user interactions produce vast streams of events that may include timestamps, page views, and session details. Quasi-structured data requires specialized parsing and sometimes advanced analytics to interpret user behavior, opening the door to targeted marketing and personalized service offerings. This approach supports new revenue channels by revealing which touchpoints encourage higher spending or faster purchasing decisions.
Unstructured data
Unstructured data lacks any predefined format, making it challenging to store, search, and analyze with standard methods. Examples include social media posts, audio clips, video recordings, or free-text documents from call center interactions. Many large organizations rely on machine learning to parse these sources, deriving insights such as sentiment scores or topic clusters to plan resource allocation. Although unstructured data can be difficult to maintain, it often holds a wealth of market intelligence that remains untapped if left unmanaged.
"A closer look at the types of big data clarifies how each form can accelerate revenue growth and operational efficiency."
Structured vs unstructured vs semi-structured
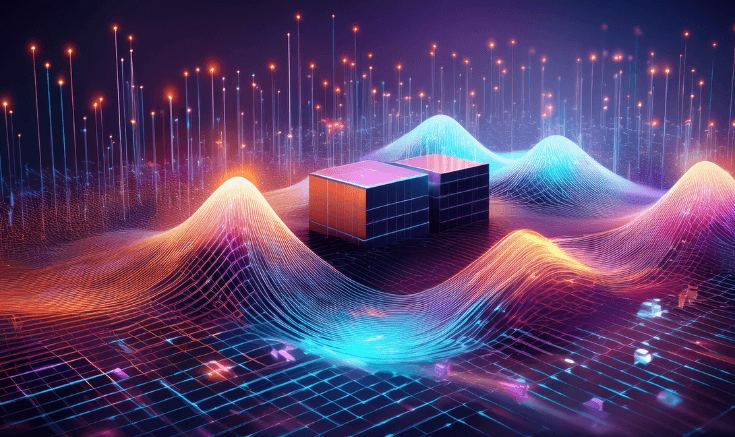
The main difference between structured, unstructured, and semi-structured data lies in their predefined format. Structured data fits into fixed fields, while unstructured data lacks any defined schema. Semi-structured data rests in the middle by offering some organizational tags but not a rigid tabular structure. Each type poses unique challenges for storage, indexing, and analysis, prompting different strategies and tools for organizations aiming to improve time to value.
Balancing these formats often requires a mix of on-premises and cloud-based solutions, tailored to your operational needs. For instance, structured data might sit in a relational warehouse for quick finance reports, whereas unstructured media files demand object storage solutions. Semi-structured data can occupy a flexible space in NoSQL databases, offering an adaptable schema that grows with new data points. The end goal is to match each data form with the best platform and analytics pipeline to maximize returns.
Data category | Format | Storage solutions | Typical use cases |
---|---|---|---|
Structured | Rigid schema (tables, columns) | Relational databases, data warehouses | Financial transactions, customer relationship management (CRM) systems |
Semi-structured | Partially defined structure (JSON, XML) | NoSQL databases, data lakes | Sensor logs, API messages |
Unstructured | No predefined schema (text, audio, video) | Distributed file systems, data lakes | Social media posts, emails |
Implementing big data for your business
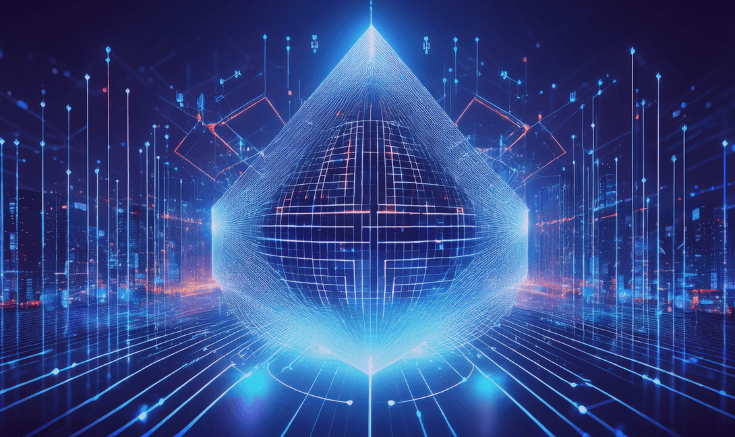
Many organizations begin with smaller pilot projects to demonstrate tangible results and secure buy-in from key stakeholders. This approach clarifies which data types in big data provide the highest value for specific use cases, from structured transaction analytics to unstructured call center transcripts. Cloud-based data lakes offer a scalable foundation that adapts to fluctuations in data volume and variety, reducing upfront investments in hardware. Early successes provide momentum for broadening big data strategies that align with cost-saving goals and accelerated market entry.
Cross-functional collaboration is another success factor, ensuring that technical experts, frontline employees, and executives understand each data category’s potential. Identifying the right types of data in big data helps unify departmental objectives, giving finance teams a clear view of revenue patterns while marketing professionals refine campaign metrics. Structured datasets often meet compliance requirements, semi-structured logs power rapid operational insights, quasi-structured clickstreams inform marketing, and unstructured text fuels sentiment analysis. Adopting the right frameworks and skill sets prevents misalignment between business objectives and data outputs. Each new expansion of big data integration paves the way for deeper analytics, stronger compliance, and targeted growth strategies.
Selecting the best data formats for your objectives requires clarity on how each category strengthens different areas of your organization. From analyzing structured transaction data to interpreting audio-based customer feedback, the right approach translates into streamlined processes and meaningful results. Collaborations across finance, marketing, and operations teams reveal fresh ways to monetize information, leading to measurable impact. Focusing on the precise types of data in big data sets the stage for consistent growth, solid compliance, and more precise forecasting.
Beyond immediate productivity gains, a robust data governance strategy ensures that your organizational knowledge remains accurate and accessible. This level of oversight helps you adapt to emerging regulations and customer demands without compromising speed or agility. Teams that prioritize data cleanliness, relevant metrics, and real-time analytics have fewer blind spots when aiming for new markets or refining existing offerings. Building a data-centered culture positions you to act swiftly on trends, minimize guesswork, and exceed stakeholder expectations.
Forward-thinking organizations treat data as a strategic asset rather than a technical afterthought. Executives plan budgets and timelines around data readiness, ensuring that growth initiatives have the support they need to succeed. Meanwhile, operational teams adopt best practices in data collection, curation, and distribution to maintain consistent quality. These principles can be part of everyday processes, helping you outpace competitors in delivering solutions that resonate with customers and stakeholders.
Big data is more than a buzzword—it's an engine for smarter, faster, and more profitable operations. It delivers flexibility and scale that free your teams to focus on real impact. At Lumenalta, we provide tailored data solutions that align with your strategic vision, ensuring you have the right architecture to succeed. Let’s chart a brighter path forward.
Common questions about big data
How do organizations pick the best types of big data to start with?
Are cloud solutions suitable for every data category?
What security measures are important for big data deployments?
Does big data analytics always involve machine learning?
How quickly can a company see ROI from big data initiatives?
Want to learn how big data can bring more transparency and trust to your operations?