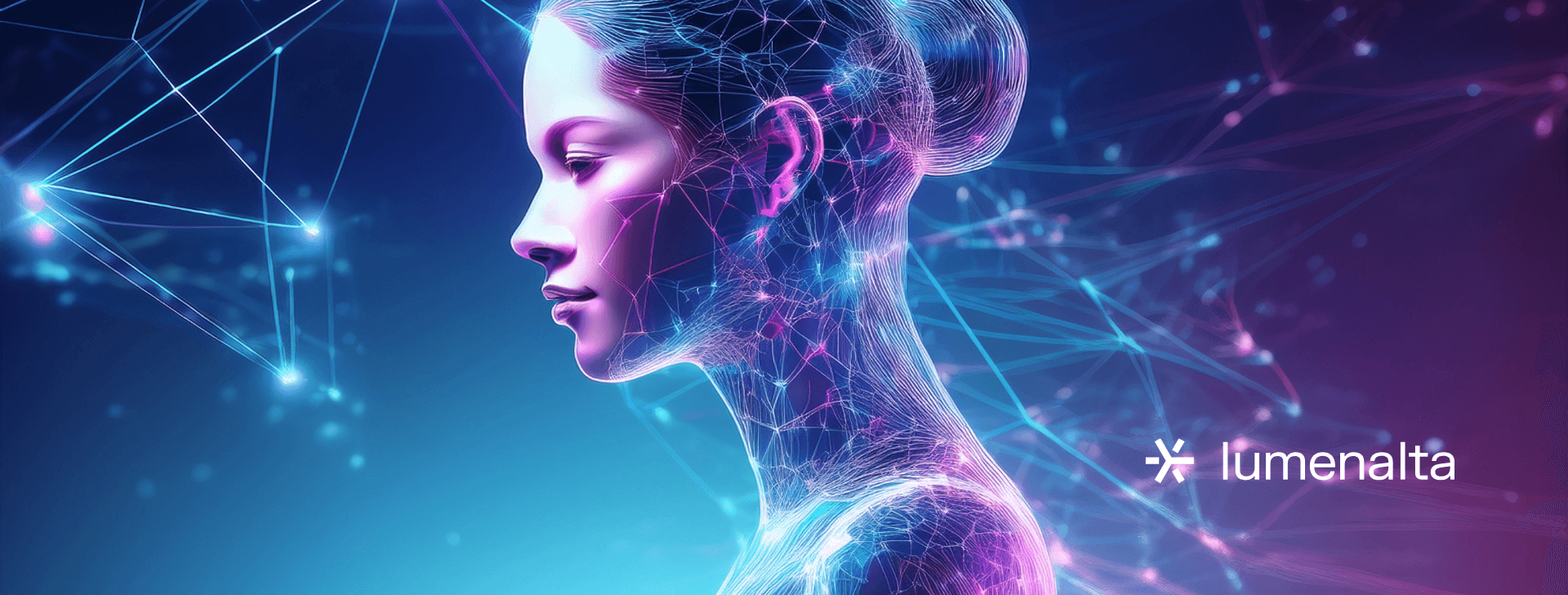
A guide to artificial intelligence and customer experience
APR. 5, 2025
6 Min Read
Delivering an AI-powered customer experience fosters loyalty, strengthens relationships, and supports stable expansion for organizations of all sizes.
Predictive analytics and real-time data processing transform every touchpoint into a personalized opportunity, offering immediate responses and specialized interactions. Cost-effective platforms built on artificial intelligence are shaping how businesses reduce overhead while maintaining high service standards. As demands grow in retail, financial services, and logistics, this approach connects organizations with new ways to boost revenue and foster trust. Consumers appreciate swift problem resolution, proactive updates, and consistency across channels, which is where AI in customer experience management becomes especially relevant. These automated solutions manage routine tasks, allowing teams to focus on more creative work and resolve exceptions promptly. Banks, for instance, have used chatbots for account inquiries and quick troubleshooting, while retailers apply computer vision for product recognition and customized marketing. Comprehensive integration of artificial intelligence in customer experience raises satisfaction levels, promotes brand loyalty, and encourages repeat engagements.
key-takeaways
- 1. AI customer experience strategies deliver real-time assistance, personalized interactions, and scalable operations to suit a broad range of sectors.
- 2. Planning with specific metrics in mind, such as reduced churn or shorter resolution times, sets a clear path for AI-enabled success.
- 3. Combining automation with a well-timed human touch protects the quality of engagement.
- 4. Robust governance of data and security safeguards future growth while maintaining compliance.
- 5. Forward-thinking teams experiment with advanced technologies like computer vision, robotic process automation, and predictive analytics.
What is AI in customer experience?
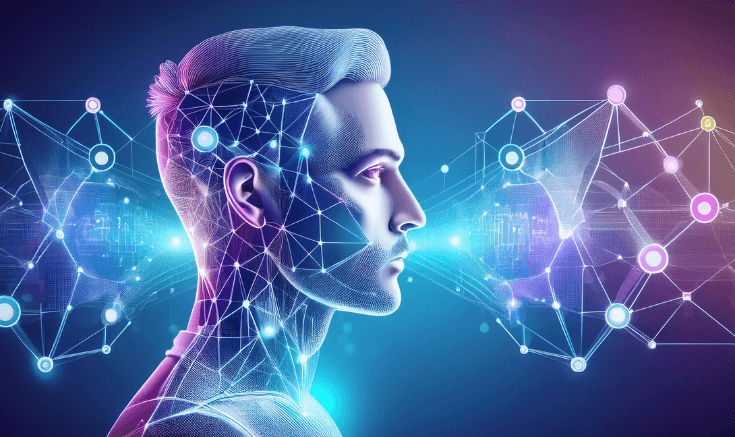
Artificial intelligence refers to algorithms and data-based models that automate tasks typically requiring human judgment. AI in customer experience centers on using these capabilities to personalize journeys, predict user preferences, and respond to inquiries without human intervention. This discipline involves predictive modeling, real-time analytics, and deep learning tools capable of refining their outputs based on historical insights. Many organizations are eager to adopt this technology to address rising expectations, reduce wait times, and provide consistent quality across platforms.
Many forms of AI for customer experience are delivered through chatbots, recommendation engines, and self-service portals that automate core services. Retailers often deploy advanced algorithms that examine browsing habits and purchase histories, creating tailored suggestions that resonate with target segments. Travel companies also build custom itineraries based on data patterns, saving time and supporting cost-effective efforts. These examples highlight how AI transforms conventional customer experiences into proactive, data-optimized workflows.
“Comprehensive integration of artificial intelligence in customer experience raises satisfaction levels, promotes brand loyalty, and encourages repeat engagements.”
Why AI in customer experience is important in 2025
Industries worldwide must streamline operations and accelerate speed to market, making artificial intelligence in customer experience vital for staying relevant. Automated systems reduce manual overhead and deliver immediate resolutions, which resonates with today’s expectations for efficient service. Data gathered across channels informs targeted outreach, allowing organizations to spot untapped business potential and design new revenue streams. This forward-thinking approach ensures that digital channels become profit centers rather than cost burdens.
Looking ahead to 2025, stakeholders value solutions that yield time to value and measurable business impact without heavy infrastructure costs. AI in customer experience achieves this by consolidating data, refining analytics, and facilitating faster approvals, ultimately boosting returns for businesses and investors. A well-planned rollout involves robust change management principles that align leaders, marketing teams, and IT departments toward shared objectives. The result is a sustainable framework that scales, supports advanced governance, and remains flexible for future updates.
Key benefits of integrating AI into customer experience
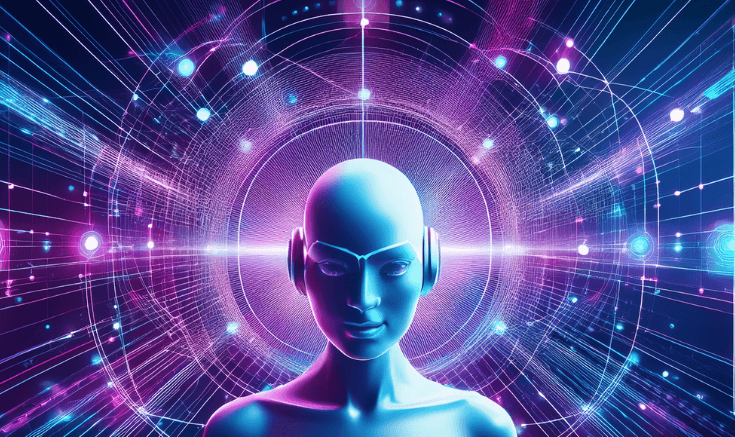
Organizations that incorporate artificial intelligence in customer experience frequently notice significant improvements in overall satisfaction and operational efficiency. Automated tasks, such as personalized messaging or claim processing, bring consistency to client interactions. This advantage leads to better resource allocation for complex challenges, reducing missed opportunities and inspiring new services.
- Personalization at scale: Automated engines analyze browsing history, transaction data, and user inputs to create offers that resonate with individual preferences.
- Faster support resolution: AI chatbots, virtual assistants, and intelligent IVR systems respond quickly to routine questions, reducing wait times and freeing up team members for advanced tasks.
- Improved forecasting: Systems track patterns and apply predictive analytics to project future trends, helping planners allocate resources more effectively.
- Consistent service quality: Automated processes standardize responses, making it less likely for human error to disrupt the experience across multiple touchpoints.
- Cost savings: Routine functions are handled digitally, cutting back on operational expenses and allowing staff to focus on higher-value activities.
Each of these benefits highlights how AI-enhanced customer experiences address diverse challenges without straining budgets. Large insurance firms, for example, have integrated these capabilities to expedite claims processing and reduce overhead. High-volume retail operations also depend on algorithm-based product recommendations to keep users engaged and returning for more. This approach leads to stronger relationships with minimal disruptions, enabling business leaders to direct capital toward growth opportunities.
Types of AI in customer experience
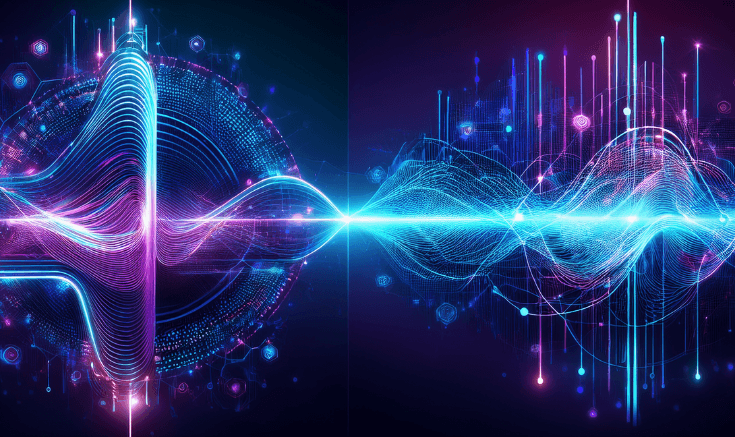
Many forms of AI help companies refine client touchpoints, from natural language understanding to robotic process automation. Each approach focuses on unique tasks, whether it’s analyzing conversations or detecting objects in images. Organizations often mix several techniques to design an all-encompassing customer experience and support high volumes of interactions. This variety accommodates different needs, from quick support calls to long-term loyalty programs.
Natural language processing
Natural language processing supports chatbots, speech assistants, and textual analytics that interpret user requests with minimal human oversight. This technology simulates conversation by mapping user input to structured data, reducing confusion and eliminating repetitive tasks. Businesses that embrace advanced language models lower operational costs while preserving quality. Many financial services teams use these solutions to expedite loan approvals and address routine questions in multiple languages.
Computer vision
Computer vision works by analyzing images or video content to recognize objects, identify patterns, or read text. Retailers rely on it for product inventory updates, self-checkout stations, and targeted advertising displays. In transportation and logistics, it can monitor package conditions and automate damage assessments, saving valuable time. This data-based strategy boosts reliability and nurtures trust among customers who expect transparency.
Predictive analytics
Predictive analytics processes historical data and real-time inputs to forecast user behavior, potential churn, and other performance indicators. This helps marketing teams craft personalized promotions, improving conversions and minimizing wasted resources. Instead of relying on guesswork, brands use these insights to plan staffing, manage supply chains, and shape future offerings. Enterprises also adopt it to spot anomalies in incoming client data, reducing fraud and reinforcing compliance.
Robotic process automation
Robotic process automation handles repetitive tasks, such as copying data between systems or verifying client information. These workflows run around the clock, ensuring consistent turnaround times and lowering human error rates. A major benefit involves bridging legacy platforms, as RPA bots can handle data entry in older interfaces without expensive overhauls. This approach helps maintain a seamless flow of operations, bolstering customer satisfaction.
Common challenges in implementing AI for customer experience
Introducing AI-powered customer experience may pose obstacles that go beyond technology. Concerns range from limited data quality to a lack of internal alignment on priorities. Leaders often face difficulties balancing quick wins with long-term governance.
- Poor data management: Incomplete or inaccurate records reduce the effectiveness of predictive models, leading to subpar results.
- Overreliance on automation: Failing to keep a human touch can cause frustration, especially for complex issues requiring empathy.
- Cultural resistance: Teams may be hesitant to accept new processes, making change management strategies essential for buy-in.
- Security vulnerabilities: AI systems require ongoing monitoring to handle data protection requirements and stay compliant with regulations.
- Budget constraints: Implementation costs, infrastructure upgrades, and maintenance can strain resources, limiting full-scale adoption.
Tackling these complications early helps organizations focus on time to value and scalable growth. Proactive governance frameworks define key roles, responsibilities, and escalation paths so that operations remain consistent across regions. Companies that balance automation with personalized care often gain loyalty and positive word-of-mouth. These best practices lay a strong groundwork for more advanced deployments down the line.
AI vs machine learning in customer experience
The main difference between AI and machine learning stems from their scope and application. AI covers the broader category of intelligent technologies, including image recognition, speech processing, and advanced analytics. Machine learning, in contrast, focuses on algorithms that learn from data and refine their predictions over time. Many industries use both approaches, bringing advanced features like text-based chatbots and recommendation engines into daily operations.
Organizations seeking AI customer experience solutions often consider whether advanced machine learning can deliver deeper personalization or more dynamic interactions. Predictive models that self-adjust based on trends often yield better outcomes and cut operational costs. Teams that adopt these tools can address growing client demands while discovering innovative revenue pathways. Selecting the right approach depends on evaluating existing systems, data maturity, and future strategic goals.
Strategies for optimizing AI-backed customer experience
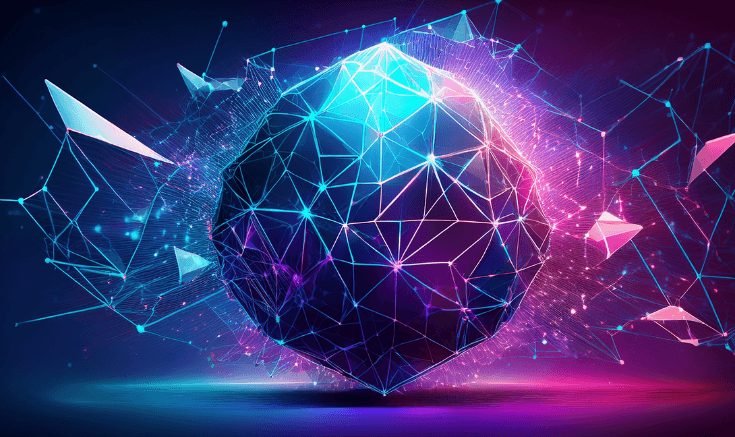
Organizations that aim to refine AI-backed customer experience often start with clear objectives around user satisfaction and operational gains. Setting defined metrics, such as response times or churn reduction, provides a baseline for progress tracking. Piloting solutions on a smaller scale reveals insights for broader rollouts, ensuring the final model fits real-time demands. A robust feedback loop from customers and frontline employees ensures continuous improvement.
Each strategy should align with existing business processes, marketing efforts, and compliance protocols. Stakeholder alignment is crucial to avoid miscommunication that leads to wasted resources or duplicated tasks. That unity also helps attract the funding required to build stable platforms, ensuring that the final product stays cost-effective.
Adopt automation tools
Selecting the right automation suite reduces manual tasks, giving teams extra capacity for strategic insights. This approach replaces tedious data entry or routing tasks that once demanded significant staff time. Enhanced workflows deliver results faster, leading to a better first-contact resolution rate and elevated user confidence. Gradual integration of these tools into existing platforms avoids disruptions and respects existing team processes.
Refine data governance
Structured data policies guarantee that each department uses consistent formats, definitions, and protocols. High-quality inputs translate into more accurate predictions, enabling stable operations that scale. Teams should categorize data sets properly to maintain privacy safeguards and comply with regulatory standards. Reliable data flows also encourage better analytics, fueling precision at every level of engagement.
Balance human touch
Automation excels in routine tasks, but personal interaction remains valuable for sensitive or high-impact issues. Creating a handoff from chatbot to agent at key friction points ensures empathy and clarity. This practice cultivates trust, demonstrating that organizations prioritize genuine connection when it counts most. A well-balanced strategy offers the best of AI technology while preserving critical human expertise.
Focus on agile iteration
Short development cycles accelerate time to value and minimize risk, since updates can be tested and refined in smaller increments. Frequent communication with sponsors clarifies requirements and helps teams adjust rapidly. Early identification of issues prevents costly rework later in the project, enhancing overall stability. This adaptable framework allows businesses to respond quickly to market changes while controlling budgets effectively.
“Stakeholder alignment is crucial to avoid miscommunication that leads to wasted resources or duplicated tasks.”
Measuring the impact of artificial intelligence on customer experience
Meaningful metrics like average resolution times, conversion rates, and customer loyalty scores show whether an AI-based customer experience delivers the expected results. These data points reveal progress, highlight gaps, and steer future improvements. Many organizations run A/B tests to determine which features drive the greatest lift in user satisfaction and revenue growth. Teams also analyze historical data to spot trends and confirm that the AI system continues to perform as intended.
Cost-benefit analysis, informed judgments, and feedback loops ensure that each enhancement meets financial goals and stakeholder interests. Regularly reviewing these key performance indicators helps maintain alignment on ROI. Some initiatives focus on reducing overhead and staff workload, while others target growth in brand reach. Tracking outcomes thoroughly keeps leadership informed and supports refined strategies.
Future trends in AI and customer experience
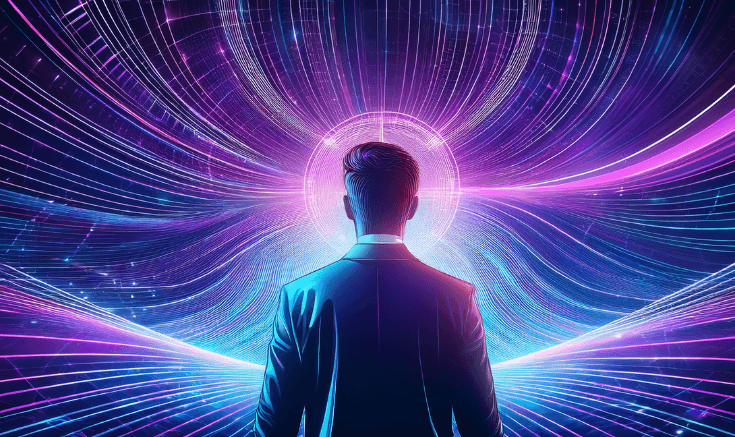
Organizations are investigating new ways to infuse artificial intelligence and customer experience with hyper-personalization and real-time engagement. These capabilities rely on sophisticated models that learn from every interaction, adapting offerings based on immediate signals. As more industries adopt cloud computing in healthcare, finance, and beyond, the infrastructure to support advanced AI strategies grows. Leaders are tapping into these solutions to uncover new market segments and expedite product development. Artificial intelligence for customer experience is evolving to address cross-channel interactions and advanced customization. Chat interfaces, voice recognition, and recommendation systems continue to expand, removing barriers once present across different platforms.
Personalization at scale
Large enterprises use AI to customize everything from discount offers to product bundles, ensuring that each consumer feels recognized. Algorithms refine suggestions based on new data, leading to continuously relevant recommendations. This approach keeps customers engaged and can boost revenue significantly. Smaller firms also apply these methods, proving that personalization is not restricted to major brands.
Predictive engagement
Systems identify triggers that suggest a user is about to exit or abandon their cart, then deliver tailored messages to recapture interest. This data-backed approach extends to service inquiries, anticipating issues before they escalate. Many telecom providers already employ these capabilities to minimize churn, resulting in more consistent income. As markets become more saturated, this proactive outreach can differentiate a brand.
Cross-channel interaction
AI now unifies messaging apps, email, social media, and phone calls, giving clients a consistent experience across every touchpoint. A single interface records each conversation, so no time is wasted repeating details. Tracking these channels also reveals preferred engagement styles, making outreach more relevant. This seamless transition eliminates confusion and amplifies user satisfaction.
Voice-powered platforms
Voice recognition and speech synthesis solutions interpret requests in seconds, assisting users who prefer hands-free commands. Home devices and virtual assistants extend these solutions into living spaces, opening new opportunities for brand engagement. Some financial institutions have introduced voice-activated payment features that give clients quicker ways to handle everyday needs. Additional enhancements in language processing promise even more effortless interactions.
AI in customer experience extends beyond refining user journeys. It propels businesses toward sustainable expansion and measurable ROI. Across sectors like logistics, retail, and financial services, artificial intelligence offers an unmatched opportunity to scale efficiently. At Lumenalta, we focus on building customized AI-based solutions that align with business objectives while fostering confidence. Let’s chart a brighter path forward.
table-of-contents
- What is AI in customer experience?
- Why AI in customer experience is important in 2025
- Key benefits of integrating AI into customer experience
- Types of AI in customer experience
- Common challenges in implementing AI for customer experience
- AI vs machine learning in customer experience
- Strategies for optimizing AI-backed customer experience
- Measuring the impact of artificial intelligence on customer experience
- Future trends in AI and customer experience
- Common questions about AI customer experience
Common questions about AI customer experience
How can AI for customer experience reduce operating costs?
Is AI in customer experience management suitable for smaller organizations?
Which industries gain the most from AI-enabled customer experience?
What data is needed to power an AI-powered customer experience?
How does artificial intelligence customer experience impact user loyalty?
Want to learn how artificial intelligence can bring more transparency and trust to your operations?