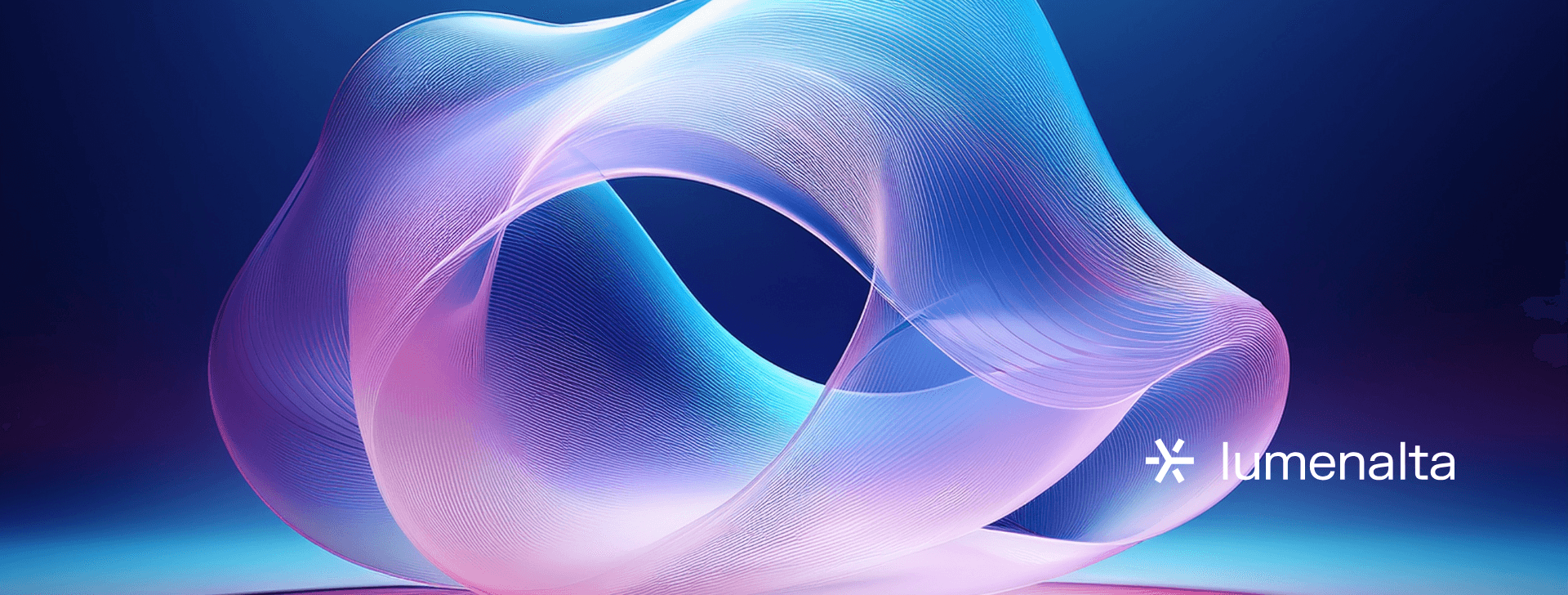
Understanding AI hyper-personalization in 2025
APR. 22, 2025
6 Min Read
AI hyper-personalization is shaping the future of customer experiences, operational workflows, and data-backed innovation.
Many enterprises view it as a powerful method for delivering individualized marketing, dynamic product offerings, and real-time decisions at scale. This shift is not just an add-on to existing solutions, but rather a key enabler of growth and profitability. Leaders in sectors like healthcare, logistics, and finance consider it an essential differentiator in crowded markets. Teams working on these initiatives often combine machine learning with advanced analytics to discover patterns and preferences that standard tools overlook. That level of insight supports new use cases, such as proactive risk assessment or predictive maintenance. Executives focus on speed to market and cost-effectiveness, making hyper-personalization with AI an attractive investment. Progress in this domain points to strong returns and meaningful advantages for organizations willing to adopt specialized data strategies.
key-takeaways
- 1. AI hyper personalization adapts user experiences in real time, offering more accurate predictions and recommendations.
- 2. Generative AI hyper personalization moves beyond classic segmentation by creating new, individualized content or products.
- 3. Executives often see significant cost savings and improved ROI when data pipelines and modeling are set up effectively.
- 4. Integration strategies include clear objectives, solid data foundations, and iterative development to ensure success.
- 5. Impact measurement involves tracking metrics such as churn reduction, user engagement, and overall profitability.
What is AI hyper-personalization?
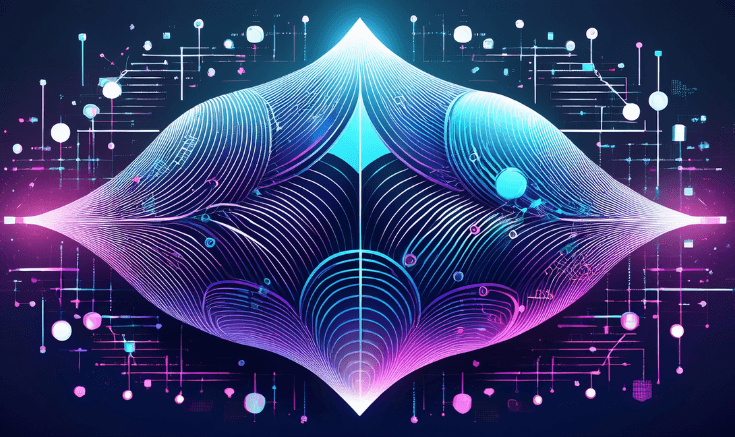
AI hyper-personalization involves customizing products, services, and interactions through advanced data analysis and machine learning. It goes beyond standard personalized recommendations by tailoring every aspect of a user's journey based on behavioral patterns, historical data, and predicted preferences. This approach requires advanced modeling and real-time analytics to accommodate even the smallest shifts in audience behavior. It often draws from aggregated information across multiple touchpoints to deliver experiences that adapt to each individual's expectations.
Organizations use AI hyper-personalization to create deeper connections with customers, stakeholders, and employees. The goal is to streamline experiences, reduce friction, and encourage loyalty through relevant touchpoints. This approach aims to reveal hidden patterns that lead to better product fit and improved overall satisfaction. Many industries treat it as an important strategy for sustainable growth, cost optimization, and data-led decision support.
"AI hyper-personalization involves customizing products, services, and interactions through advanced data analysis and machine learning."
AI personalization vs generative AI hyper-personalization
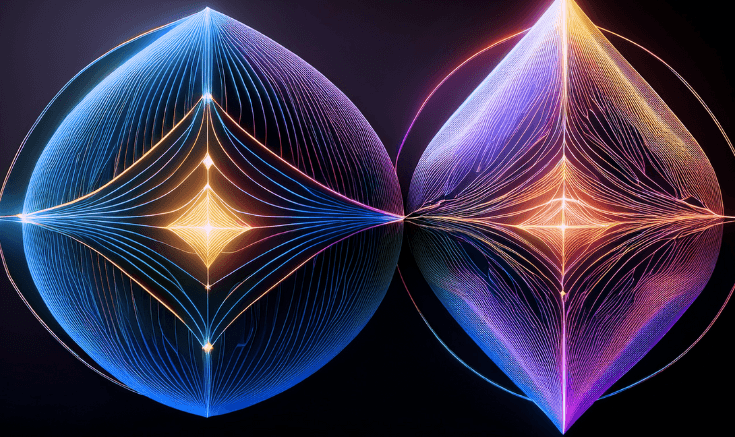
The main difference between AI personalization and generative AI hyper-personalization lies in the extent of tailored outputs. Traditional personalization solutions rely on algorithms that segment users into categories and deliver content or recommendations based on general patterns. Generative AI hyper-personalization takes this further by using generative models to create new content, product variants, or interactive elements that match each individual's profile. This approach relies on large language models and other generative technologies to produce outputs that feel unique at scale.
Generative AI hyper-personalization often leverages advanced data pipelines that integrate unstructured sources such as text, images, and behavioral trends. The objective is to craft experiences that resonate at a granular level, such as dynamic product designs, marketing messages, or user interfaces. This level of depth can help organizations reduce time to market and maintain agility in shifting consumer or stakeholder demands. It also brings new challenges in data governance, model maintenance, and ethical considerations, making it a sophisticated but powerful approach.
Benefits of AI hyper-personalization
Many professionals seek AI hyper-personalization to achieve immediate results and long-term improvements in customer and operational management. A structured approach can elevate data utilization, reduce costs, and refine overall strategy. Each advantage can drive measurable value for businesses aiming to align with evolving user expectations.
- Stronger customer loyalty: Tailored experiences often keep users engaged, supporting repeat interactions that influence higher retention.
- Efficient resource usage: Advanced models target specific needs, preventing wasted development time on features or services that users do not require.
- Real-time adaptation: Systems can refine responses on the fly, allowing businesses to adjust to patterns and trends faster than traditional tools.
- Predictive accuracy: Intelligent algorithms forecast user behavior with improved precision, driving actions that align with future demands.
- Personalized marketing: Campaigns resonate more effectively when messaging is tailored, leading to higher conversions with less spend.
- Scalable approaches: Automated processes adapt to user growth without requiring constant manual oversight.
These benefits can generate a lasting impact across a range of sectors. Decision-makers appreciate the efficiency gains and cost savings, while end-users enjoy experiences that feel relevant and purposeful. Each facet of AI hyper-personalization can contribute to a more agile framework for innovation. This technology creates opportunities to uncover untapped business potential and achieve measurable progress.
Applications of AI hyper-personalization across industries
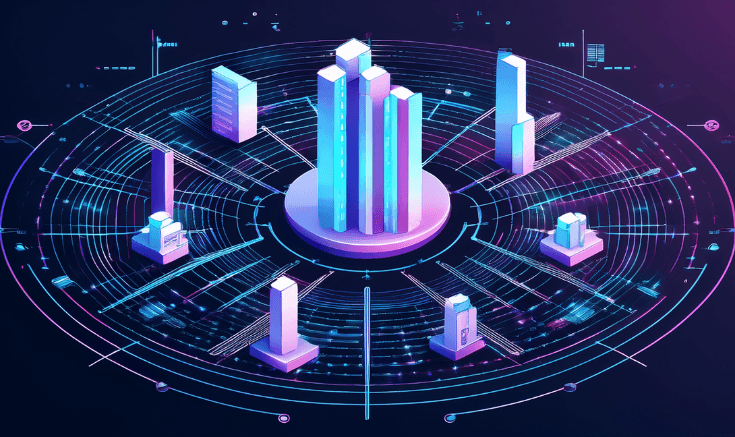
Many organizations incorporate AI hyper-personalization to strengthen user engagement, improve operational workflows, and reveal novel revenue streams. Different sectors adopt these approaches to address unique business challenges with tailored solutions. Practical applications range from marketing platforms to robust data-backed forecasting. The following areas highlight how various industries benefit when technology meets specific goals.
Retail and e-commerce
Retailers often rely on AI hyper-personalization to present product recommendations that match user preferences in real time. Data collected from browsing habits, purchase history, and social sentiments helps guide decisions about inventory and marketing. Some platforms also adjust pricing dynamically to capture maximum revenue based on live demand. This approach boosts profitability by delivering options that align with each shopper's profile.
Logistics and supply chain
Organizations that handle complex distribution networks benefit from AI hyper-personalization by customizing routes and inventory management around real-time signals. This method helps minimize delays, reduce operational costs, and speed up time to market for critical goods. It also supports more accurate demand forecasting, allowing management to scale resources effectively. Tailored delivery schedules and shipping methods promote efficiency across multiple stakeholders.
Financial services
Banks and insurance providers are turning to AI hyper-personalization for risk assessment and individualized product offerings. Payment solutions, investment portfolios, and customer support can all be refined with the right data-based insights. This approach shortens response times, lowers fraud risk, and aligns products with a client's financial goals. Many institutions achieve better client retention and revenue growth when implementing targeted, adaptive strategies.
Healthcare and wellness
Healthcare practitioners explore AI hyper-personalization to improve patient engagement and treatment plans. Personalized health recommendations, medication tracking, and remote monitoring solutions often feed into improved patient outcomes. Providers can also optimize resource allocation by analyzing patterns in patient flows and predicting next steps. This helps drive better care while managing budgets more effectively.
Each industry adapts AI hyper-personalization in unique ways to meet specific demands. The potential for scalable growth, predictive power, and user satisfaction stands out as a unifying thread. Refined data pipelines and intelligent modeling support a wide range of workflows that extend beyond traditional personalization tactics. Many organizations set themselves apart by using these methods to drive bottom-line results and strategic innovation.
Challenges in implementing AI hyper-personalization
Despite its advantages, AI hyper-personalization can present hurdles that require thorough planning and alignment among teams. Complex data pipelines, evolving consumer expectations, and the need for advanced infrastructure can complicate adoption. Some organizations encounter limitations with data quality or legacy systems that slow progress. A holistic view of these obstacles helps leaders make informed decisions.
- Data privacy compliance: Detailed insights demand sensitive information, raising concerns about how data is stored, processed, and protected.
- Model complexity: Sophisticated algorithms need dedicated expertise and robust computing resources to function reliably.
- Integration hurdles: Legacy platforms might not support modern data flows, leading to additional costs for system upgrades or custom development.
- Ethical considerations: Personalized experiences must avoid bias and unfair outcomes, which can damage consumer trust.
- Limited talent pool: Skilled engineers, data scientists, and subject matter experts can be difficult to find or retain.
- Scalability: Large-scale deployments often require significant hardware, software, and maintenance investments.
These challenges add layers of complexity that decision-makers must factor into their strategic planning. A strong governance model can mitigate risks, and structured collaboration among stakeholders often leads to better project outcomes. Organizations that prioritize transparent communication and targeted investments have the best chance of success. Each hurdle can be addressed by clear objectives and a well-defined roadmap.
Strategies for effective AI hyper-personalization implementation
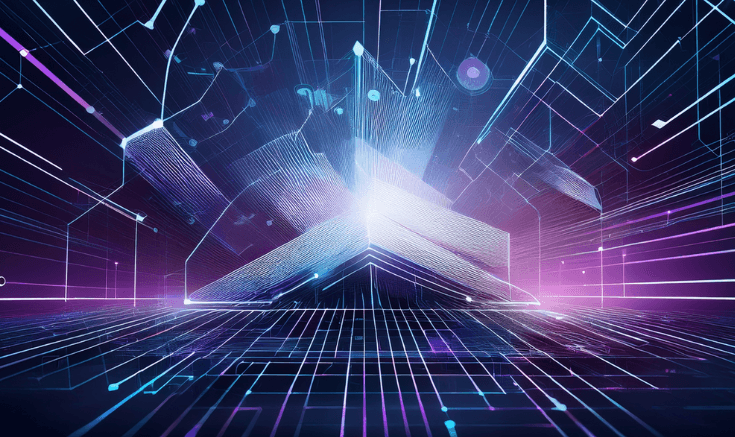
Many organizations find success with clear planning, well-defined budgets, and stakeholder engagement. A detailed roadmap ensures each phase of AI hyper-personalization is executed with measurable outcomes in mind. Integrating data from multiple channels is a high priority, along with selecting the right models to match business objectives.
Define objectives and scope
Leaders set precise targets around user engagement, cost efficiencies, or other KPIs before introducing AI hyper-personalization. This clarity helps teams focus on goals that deliver tangible returns. Narrowing the scope ensures quick wins and reduces complexity when scaling. Early alignment between technical and business units promotes effective resource allocation.
Invest in data quality and infrastructure
Consistent, accurate, and relevant data are critical for robust modeling. Storage solutions, processing tools, and networking must handle large data volumes with minimal latency. Cloud-based systems often support flexible scaling, especially when usage spikes are unpredictable. An optimized infrastructure shortens deployment cycles and lowers operational costs in the long run.
Adopt iterative development cycles
Small, phased rollouts let teams identify what works best and revise parts that require improvement. Feedback loops keep every stakeholder informed, maintaining agility as user needs shift. Consistent testing ensures the final system meets performance standards without wasting resources. This approach moves from concept to production with fewer setbacks.
Provide training and change management
Organizations that guide employees through the adoption process often see stronger ROI. Workforces benefit from regular workshops, hands-on practice, and access to knowledge-sharing networks. This preparation streamlines operations and fosters a culture of continuous improvement. Clear roles and responsibilities build momentum toward a sustainable AI hyper-personalization model.
Implementing these strategies can help organizations accelerate time to value. Thoughtful planning, solid data foundations, and effective collaboration form the backbone of any successful AI hyper-personalization endeavor. Leaders gain confidence when they see steady progress and measurable outcomes. Strategic execution paves the way for long-term benefits across multiple functions.
"A detailed roadmap ensures each phase of AI hyper-personalization is executed with measurable outcomes in mind."
Measuring the impact of AI hyper-personalization
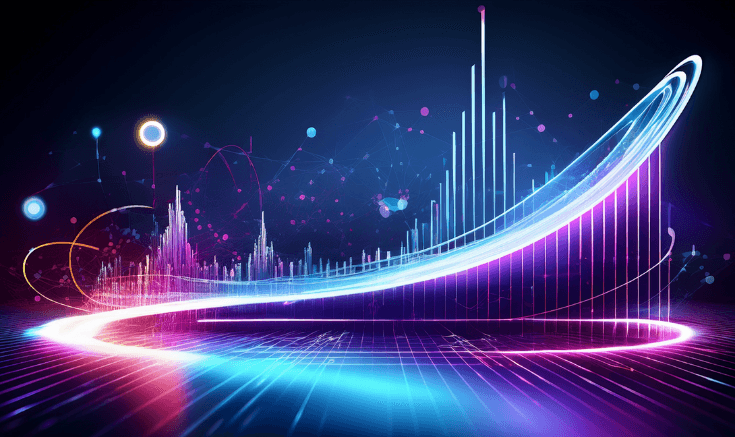
Organizations often track metrics like conversion rates, churn reduction, and net promoter scores to verify the effectiveness of AI hyper-personalization. These data points offer insight into real-time improvements in user satisfaction and operational efficiencies. Advanced dashboards allow leadership to compare outcomes against predetermined benchmarks and refine tactics where needed. Clear measurement frameworks highlight cost savings, improved margins, and overall growth potential.
Some leaders also incorporate qualitative feedback, such as user satisfaction surveys or support interactions, to complement quantitative results. A balanced view ensures that new initiatives address not only revenue or cost but also brand reputation. Reliable data collection methods help validate the return on investment, which resonates with stakeholders who want proof of meaningful progress. Consistent analysis drives continuous improvement, positioning AI hyper-personalization as a sustainable and value-powered capability.
AI hyper-personalization is not just an innovation—it’s a strategic way to refine products, services, and user journeys. Focus on optimization and adaptability leads to stronger engagement across multiple touchpoints. At Lumenalta, we specialize in implementing tailored data solutions that align with your strategic ambitions, ensuring each investment drives value. Let’s chart a brighter path.
table-of-contents
- What is AI hyper personalization?
- AI personalization vs generative AI hyper personalization
- Benefits of AI hyper personalization
- Applications of AI hyper personalization across industries
- Challenges in implementing AI hyper personalization
- Strategies for effective AI hyper personalization implementation
- Measuring the impact of AI hyper personalization
- Common questions about AI hyper personalization
Common questions about AI hyper personalization
How does AI hyper personalization improve customer loyalty?
What distinguishes generative AI hyper personalization from traditional methods?
Which metrics are important for measuring AI hyper-personalization success?
How can businesses handle data privacy concerns when implementing AI hyper-personalization?
Is AI hyper-personalization feasible for smaller organizations?
Want to learn how AI hyper personalization can bring more transparency and trust to your operations?