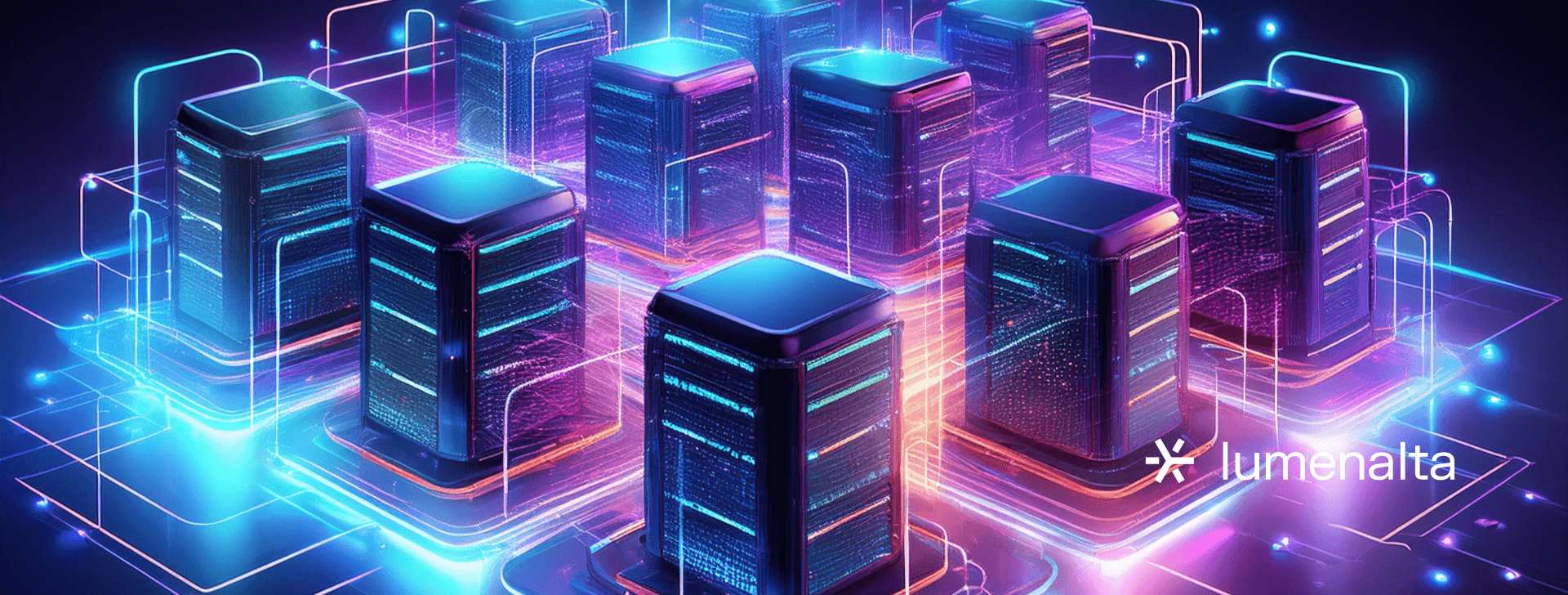
6 steps for building an AI data center
JAN. 19, 2025
6 Min Read
Artificial intelligence (AI) data centers are the foundation of modern innovation, powering everything from real-time analytics to advanced automation.
With artificial intelligence driving unprecedented necessity for high-performance infrastructure, the ability to build a cost-effective, scalable, and energy-efficient AI data center has become a critical priority for businesses. Investments like Microsoft’s recent $80 billion commitment to AI data center expansion highlight the strategic value of robust infrastructure in supporting large-scale machine learning models and improving operational workflows. AI data centers are no longer a luxury—they are necessary.
Key takeaways
- 1. AI data centers require specialized infrastructure, including high-performance processors, scalable storage, and low-latency networks, to meet the demands of AI workloads.
- 2. Advanced cooling systems and renewable energy options improve energy efficiency and reduce operational costs, making AI data centers more sustainable.
- 3. Selecting the right location is critical for optimizing costs, reducing latency, and supporting energy-efficient operations.
- 4. Scalability through modular designs and hybrid cloud strategies helps future-proof AI data centers for growth and shifting needs.
- 5. AI data centers deliver measurable business impact by improving operational efficiency, supporting innovation, and enabling faster time to value.
Planning your AI data center
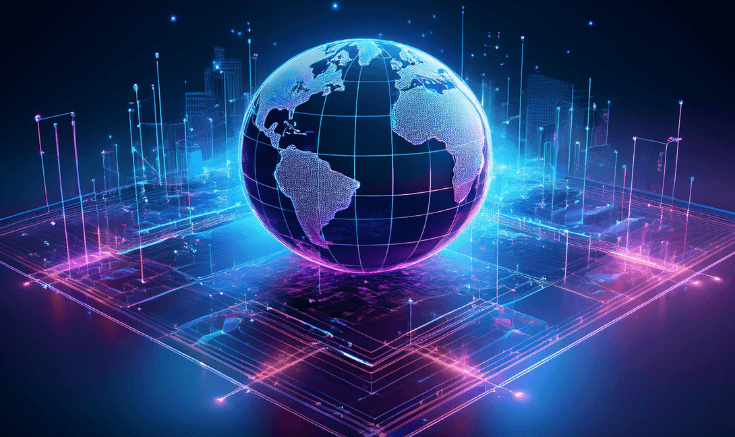
As artificial intelligence continues to reshape industries, building an AI data center has become a critical investment for businesses looking to optimize their operations, improve efficiency, and drive innovation. However, planning an AI data center requires a strategic, well-informed approach to ensure it meets the unique needs of AI workloads while remaining cost-effective and scalable.
A successful plan begins by understanding the purpose of the data center. AI workloads differ significantly from traditional computing tasks, requiring specialized hardware, immense processing power, and robust cooling systems to support machine learning models and high-performance computing (HPC). Organizations must also consider future scalability, ensuring the infrastructure can accommodate increasing requirements as AI adoption grows.
Key factors to include during the planning phase are power requirements, cooling strategies, and network architecture. Additionally, selecting the right location is essential, as proximity to reliable power sources and network connectivity can reduce latency and operational costs. A clear focus on these elements during the planning stage helps align the project with your business goals, making the AI data center a long-term asset.
"Every successful AI data center project begins with a clear understanding of its purpose, identifying specific applications, workloads, and business outcomes."
AI data center infrastructure requirements
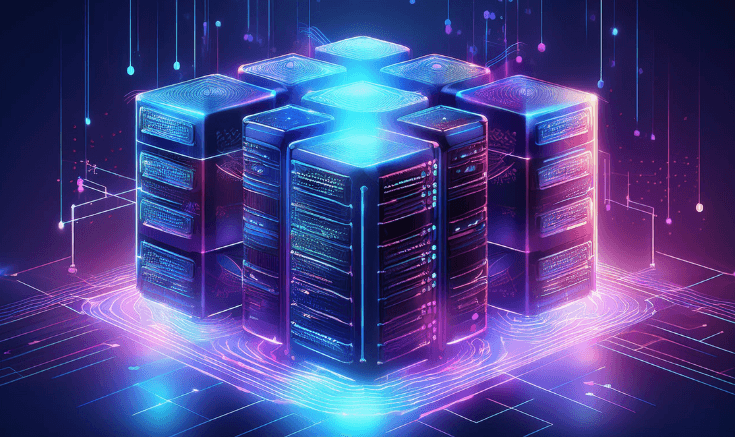
Building an AI data center involves creating a foundation that can support the demanding computational workloads of artificial intelligence. AI requires significantly more robust infrastructure than traditional data centers, as it processes massive datasets, handles complex algorithms, and runs high-intensity machine learning models. Without the right components, performance can suffer, costs rise, and scalability becomes challenging.
- High-performance computing systems: AI workloads calls for processing power optimized for parallel operations. GPUs (graphics processing units) and TPUs (tensor processing units) are widely used to accelerate model training and data analysis. These specialized processors reduce time to results, helping businesses get ahead.
- Scalable storage solutions: AI applications require efficient storage to handle large-scale datasets without bottlenecks. High-speed SSDs (solid-state drives) and NVMe (non-volatile memory express) storage deliver low-latency performance, keeping data readily accessible for processing tasks.
- Advanced networking equipment: Low-latency, high-bandwidth connections are essential for seamless data transfers and uninterrupted AI operations. Many AI data centers implement 100Gbps or faster Ethernet networks to meet these needs.
- Cooling systems tailored for AI: Intensive workloads generate significant heat, making effective cooling systems a priority. Liquid and immersion cooling are cost-effective solutions supporting hardware longevity and performance.
- Reliable power supply systems: Stable power delivery is essential for running AI workloads smoothly. Redundant power systems and sustainable energy sources can reduce downtime, enhance operational efficiency, and lower energy costs.
- Specialized AI software: AI-optimized frameworks, such as TensorFlow, PyTorch, and Kubernetes, streamline tasks like model training, data processing, and deployment. These tools simplify workflows while improving operational efficiency.
- Robust security protocols: Protecting sensitive data is a top priority. AI data centers implement multi-layered cybersecurity measures, such as encryption, firewalls, and identity management systems, to mitigate risks while maintaining compliance with regulations like GDPR or HIPAA.
A well-architected AI data center provides the reliability, efficiency, and flexibility required to support business innovation. Organizations can achieve faster time to value, reduce operational complexity, and unlock new growth opportunities with a robust infrastructure. Each of these elements contributes to a future-ready data center designed to handle the shifting requirements of artificial intelligence.
6 steps for building an AI data center
Establishing an AI data center involves a well-structured approach to meet the unique requirements of artificial intelligence workloads. Unlike traditional data centers, AI-focused facilities must handle high-intensity computational tasks, process massive datasets, and provide scalability for future growth. A step-by-step process simplifies execution and ensures the infrastructure aligns with business objectives like faster time to value, cost efficiency, and measurable outcomes.
Step 1: Define your data center goals
The first step in building an AI data center is clarifying its purpose and objectives. Organizations must determine how the data center will support their AI initiatives, such as automating processes, improving product insights, or allowing large-scale model training. Clear goals create a foundation for evaluation throughout the project.
When goals are clearly defined, it becomes easier to prioritize infrastructure investments that deliver tangible business value. A company aiming to enhance processing speed for AI research may focus on cutting-edge processors, while one looking to minimize costs might prioritize energy efficiency. Aligning goals with measurable outcomes ensures every aspect of the data center contributes to long-term success.
Starting with well-defined objectives reduces the risk of misalignment, keeps stakeholders engaged, and sets the stage for building an AI data center that delivers value from the outset.
Step 2: Choose the right location
The physical location of an AI data center plays a vital role in its performance and operational costs. Proximity to robust network infrastructure and reliable power sources can significantly reduce latency and downtime. Organizations prioritizing energy efficiency often select regions with access to renewable energy options like wind or solar power.
Climate conditions are another critical consideration. Facilities located in cooler regions require less energy for cooling, which reduces operational costs over time. Tax incentives, regulatory compliance, and access to skilled labor are key factors when selecting a location.
Choosing the right location ensures the AI data center remains cost-effective, supports business objectives, and minimizes long-term operational challenges.
Step 3: Secure sufficient power capacity
AI workloads require significant energy resources, making power capacity a fundamental consideration during planning. Facilities must support high-performance computing systems and accommodate peak workloads without interruptions. Redundant systems, such as backup generators and uninterruptible power supplies, protect against power outages.
Renewable energy sources like solar or wind power can help organizations achieve sustainability targets while reducing energy costs. Many businesses also invest in energy management systems to optimize consumption and monitor power usage in real time.
Reliable power infrastructure supports operational stability and positions the data center as a sustainable and scalable resource for AI-backed initiatives.
Step 4: Implement advanced cooling systems
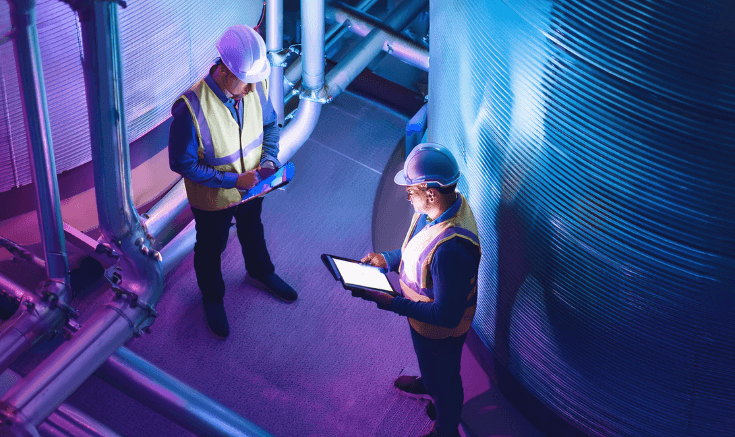
AI hardware generates significant heat, making efficient cooling systems essential for maintaining performance and preventing downtime. Traditional cooling solutions often fail to meet the needs of high-performance processors like GPUs and TPUs, leading organizations to adopt advanced technologies.
Liquid and immersion cooling systems have gained popularity due to their ability to manage heat and efficiently reduce energy consumption. Liquid cooling uses specially designed coolants to absorb and dissipate heat, while immersion cooling submerges components in thermally conductive fluids. These methods lower operational costs and improve the longevity of hardware systems.
Investing in innovative cooling solutions directly impacts energy efficiency and operational performance, making it a critical step in building an AI data center that aligns with business goals.
Step 5: Optimize network architecture
AI workloads rely on low-latency, high-bandwidth networks to handle the transfer of large datasets and enable seamless processing. A well-optimized network architecture eliminates bottlenecks and supports real-time operations for applications like predictive analytics or machine learning inference.
High-speed Ethernet connections, fiber-optic networks, and software-defined networking (SDN) enhance data flow and improve efficiency. Redundant connections within the network architecture provide additional reliability, ensuring uninterrupted operation during peak usage or maintenance periods.
A carefully designed network architecture accelerates processing times, reduces delays, and delivers the performance required for high-intensity AI workloads.
Step 6: Plan for scalability and future growth
Scalability is critical to an AI data center’s long-term success, particularly as pursuits for AI-led insights and automation grow. Modular designs, scalable hardware solutions, and cloud-native platforms allow facilities to adapt to needs without significant disruptions.
Incorporating hybrid cloud strategies or edge computing frameworks can also improve scalability. These approaches distribute workloads more efficiently, reduce latency, and support localized AI applications where needed.
Planning for growth allows businesses to remain flexible, identify new opportunities, and maximize returns on their data center investments. A scalable facility provides the agility to meet current and future AI requirements.
A structured approach to building an AI data center ensures it delivers measurable results and supports the organization's broader goals. Each step contributes to creating a cost-effective, high-performing resource and ready to unlock new business opportunities, from defining objectives to planning for future growth.
"AI workloads rely on low-latency, high-bandwidth networks to handle the transfer of large datasets and enable seamless processing."
Measuring business impact of AI data centers
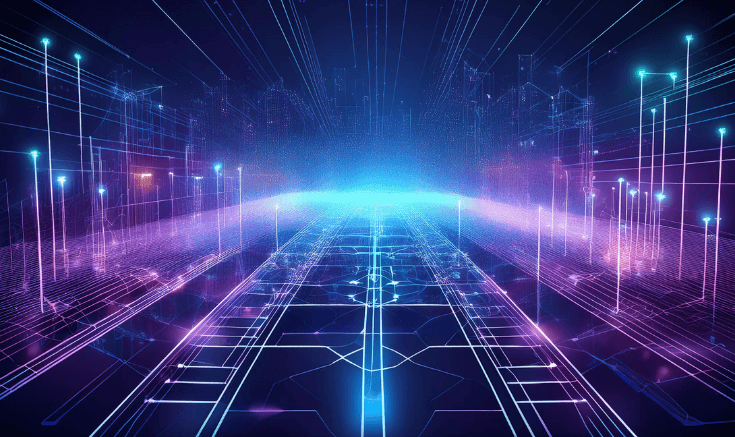
AI data centers are more than just infrastructure—they represent a strategic investment that can modify business operations and create measurable outcomes. Evaluating the business impact of these facilities requires aligning their capabilities with key organizational objectives, such as improving efficiency, accelerating innovation, and maximizing returns on investment.
One of the most immediate impacts of an AI data center is the ability to process large datasets at unparalleled speeds. This capability directly translates to faster product development cycles, more precise insights, and improved automation across various functions. These efficiencies result in reduced time to market, lower operational costs, and the potential to capitalize on untapped opportunities.
AI data centers also contribute to long-term value by permitting scalable solutions that adapt to future business needs. Their flexibility allows companies to deploy advanced AI models without frequent infrastructure overhauls, reducing costs while supporting growth. Additionally, organizations gain an advantage by leveraging AI-led insights for strategic initiatives, improving customer engagement, and refining operational processes.
The measurable business impact of AI data centers is seen in operational efficiency, cost savings, and improved scalability. These facilities provide the foundation for companies to innovate, streamline processes, and create value for stakeholders in a way that traditional infrastructure cannot match.
AI data center sustainability and scalability in 2025
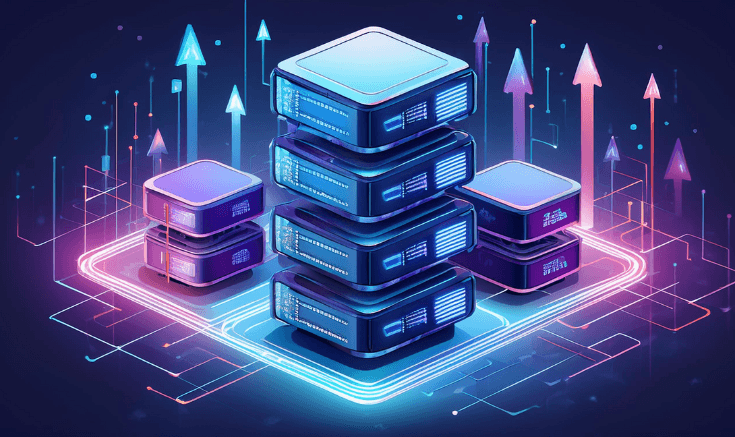
Sustainability and scalability are at the forefront of AI data center design in 2025 as businesses prioritize efficiency, cost management, and environmental responsibility. Modern AI data centers are expected to handle the growing needs of artificial intelligence and meet stringent energy and sustainability standards to minimize their ecological footprint.
Energy efficiency is a key focus area. AI data centers are adopting renewable energy sources, such as solar, wind, and hydroelectric power, to offset their substantial energy requirements. Advanced cooling technologies, such as immersion and liquid cooling, further contribute to sustainability by reducing the energy consumption typically associated with traditional cooling methods. These innovations lower operational costs and improve compliance with sustainability goals, making data centers more cost-effective in the long term.
Scalability has become equally critical as AI adoption grows across industries. Modular data center designs are becoming standard, allowing organizations to scale their infrastructure without significant disruptions. Edge computing is another emerging trend, equipping localized processing closer to users while reducing latency and improving network efficiency. This hybrid approach to scalability supports varied workloads, from small-scale AI applications to enterprise-level machine learning models.
In 2025, AI data centers are no longer just facilities but strategic assets designed to support sustainable operations and future-ready growth. This dual emphasis on sustainability and scalability equips businesses to maximize efficiency while aligning with regulatory and environmental standards.
AI data centers are transformative assets that unlock new efficiency, scalability, and innovation levels. Investing in the proper infrastructure and strategies enables businesses to build facilities that deliver measurable results and long-term value. At Lumenalta, we partner with organizations to design and implement tailored solutions for AI data centers, helping you achieve your goals while staying ahead of industry needs. Let’s build a brighter future together.
table-of-contents
- Planning your AI data center
- AI data center infrastructure requirements
- 6 steps for building an AI data center
- Step 1: Define your data center goals
- Step 2: Choose the right location
- Step 3: Ensure sufficient power capacity
- Step 4: Implement advanced cooling systems
- Step 5: Optimize network architecture
- Step 6: Plan for scalability and future growth
- Measuring business impact of AI data centers
- AI data center sustainability and scalability in 2025
- Common questions about AI data center
Common questions about AI data centers
What is the purpose of an AI data center?
How does an AI data center differ from a traditional one?
What factors should be considered when selecting an AI data center location?
How do advanced cooling systems benefit AI data centers?
Why is scalability important for AI data centers?
Want to learn how artificial intelligence can bring more transparency and trust to your operations?