5 best practices for effective data product management
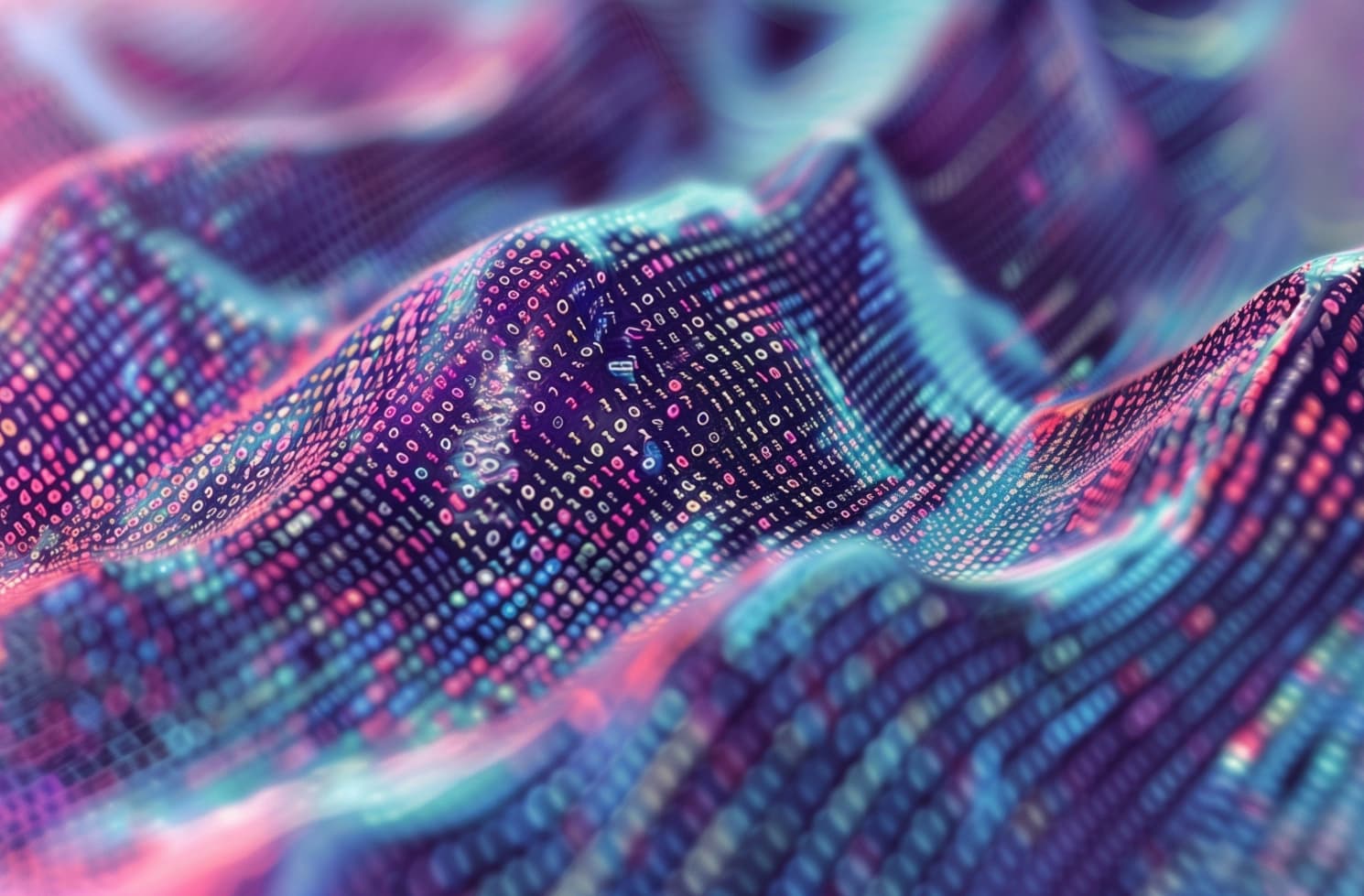
APR. 25, 2024
An optimized data product management ensures strategic data initiatives are executed successfully with stakeholders of all technical backgrounds.
Unlike some digital product initiatives, data product management involves intricate layers of data governance, integration, and analysis. From the product side, it requires a nuanced approach to planning, execution, and progress visualization.
At Lumenalta, product analysts play a pivotal role in ensuring transparency, accuracy, and progress across a diverse stakeholder group of technical and non-technical stakeholders.
From aligning stakeholder interests to showcasing the advancement of the technology in weekly alignment sessions, product managers face a myriad of hurdles in steering to success.
Here are five best practices to help guide you towards optimized product management in this domain. When executed with these in mind, strategic data initiatives can be executed successfully in engagements of small to large sizes and with stakeholders of all technical backgrounds.
1. Communicate early with technologists and engage with stakeholders to identify priority business insights.
In most cases, there is a lot of data that can be collected and looked at in a multitude of ways. It’s important to have the conversations as early as possible to understand what kind of business insights you want to gain from the data. Then, prioritize the ones that will be of the biggest impact/gain and set to work on those first.
By identifying your business data key performance indicators at the start and prioritizing the foundational business data, you lay the groundwork for your data strategy solution.
Ultimately, this approach enables you to implement additional data collection more rapidly and opens up the ability to build in parallel without the risk of reworking the solution.
Stakeholder management
In addition to identifying and prioritizing foundational business data, effective stakeholder management is crucial for the success of your data strategy. Early communication with stakeholders about the required business data fosters alignment and ensures that their needs and objectives are fully understood.
With their key insight on intended business decisions to be informed by the data, you can prioritize data collection efforts accordingly.
This proactive approach not only lays the groundwork for the data strategy but also enhances stakeholder buy-in and support throughout the implementation process.
Actively involving stakeholders from the outset, increases your potential to streamline decision-making processes, mitigate potential risks, and accelerate the execution of your data strategy initiatives.
2. Build milestones around each data source, and identify the decision makers for each.
To ensure that the data solution remains true to the strategic vision, it’s important to create visibility with stakeholders even without a tangible product to show.
For each data source, identify the key milestones to get the work to release. With these milestones, your team can align on a structured framework for managing the progression of each data source within the data project, ensuring transparency, accountability, and alignment with strategic goals.
Here’s what a potential data product roadmap could include:
Data source identification
Data retrieval
Data transformation
Data integration
Quality assurance
Stakeholder review and approval
Each project will likely have a different set of stakeholders who are critical in understanding the specific data source and how that data needs to be retrieved, transformed, and sent to the organization for the business to use.
Approach this efficiently by customizing your strategy for the stakeholders you are working with from the beginning.
3. Introduce product visualization with creative demos.
Data projects do not have an obvious visual user interface (UI) for the stakeholders to see and compare to designs, making it difficult to measure progress. For stakeholders to still feel the impact your team is constantly making, think outside the box and find ways to visualize the work — whether in diagrams, infographics, or a presentation.
In one of my client projects, we developed an architecture diagram of all the data sources and services and how they would connect. With this approach, we could highlight key points in the diagram each week during demos.
To support strong product visualization, consider creating a presentation with the real data being ingested: demonstrate how the new work being completed enables the creation of a specific data output that directly ties to a specific business goal.
Data projects will take a little more foundational creative thought and preparation to demo to the stakeholders, but it’s important to take the time and invest upfront to showcase the progress being made.
4. Communicate to stakeholders the backend work.
There is so much ‘unseeable’ work that goes into a data strategy project. Arguably, it’s 90% of the work.
There are no designs to review with stakeholders and no clear user flows for the product. While the required functionality is mostly the same, the data that needs to be included is coming from many different sources.
Knowing this, from an early stage in the project, you need to leverage planning tools (e.g., business priorities, roadmaps, key milestones, etc.). Support your organization by layering a transparent communication strategy to stakeholders with complete documentation and feedback loops to highlight the background work.
Introducing daily or biweekly standup meetings can go a long way in highlighting the level of effort behind the data, as well as address questions and seek clarifications early on before risking rework down the road.
See the backend work for this Minibar Delivery project.
5. Include easy-to-understand monitoring and UI tools where possible for end-users.
Even though data projects are complex, there is, of course, still an end-user that needs to understand how to use the final product. Knowing who the end-user is at the business and their needs is critical to the project’s success.
Include work that provides the end-user with the monitoring and UI tools they need to do their job simply and effectively — whether that is a health status indicator for a data ingestion point or a tool to allow them to query specific data and pull it into visual charts and graphs.
Here are four UI tools to create transparency and efficiency in data projects:
1. Amazon Quicksight
Enhance the effectiveness of a data project by democratizing data access and enabling stakeholders to derive actionable insights from their data. Quicksight is made to be small and quick even for the largest datasets.
2. Microsoft Power BI
This tool enables users to connect to various data sources, transform raw data into meaningful insights, and create interactive visualizations and reports due to its versatility and customizability. Its advanced analytics capabilities, such as machine learning and natural language processing, enable deeper insights and predictive analytics.
3. Salesforce Tableau
Use Salesforce Tableau to aggregate and analyze data from Salesforce CRM and other sources, create dynamic dashboards and reports, and share business insights with stakeholders across the organization. Its predictive modeling capabilities further enhance its utility in empowering data-driven decision-making and optimizing business processes.
4. Google Looker
Enhance visualization by providing users with intuitive features and ad-hoc data exploration. Looker can help organizations centralize and analyze data from various sources to uncover valuable insights, de-siloing business approaches throughout data models.
No matter what the user aims to achieve with this solution, it’s crucial to include data product management in the initial strategic planning and implementation phases of the project.
By doing so, you ensure the final product delivers a meaningful impact. Investing the effort upfront, during the early stages of the data project, unlocks its full potential for the organization.