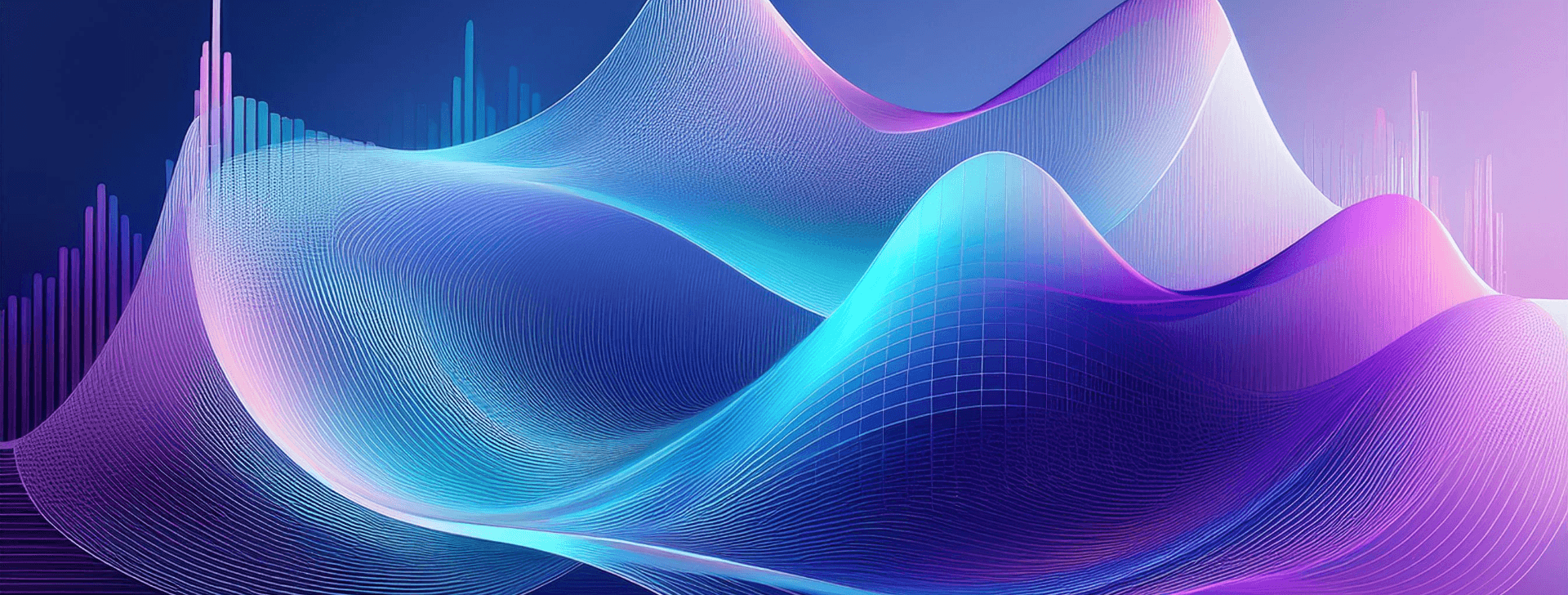
Data ingestion vs. data integration
NOV. 13, 2024
8 Min Read
With digital transformation, understanding the distinct roles of data ingestion and data integration is crucial for businesses.
Data ingestion and integration serve as foundational processes within data management, each playing a specific role in preparing, transferring, and combining data for analysis and application. Here, we’ll explore these concepts in depth, address their differences, and highlight best practices and future trends that will empower your business to make the most of its data resources.
Key takeaways
- 1. Data ingestion and data integration serve distinct but complementary roles within data management, with ingestion focused on gathering and storing data and integration dedicated to unifying and refining it.
- 2. Real-time and batch processing options for data ingestion allow organizations to address both immediate and periodic data requirements, supporting a wide range of applications.
- 3. Effective data integration combines data sources into a single, coherent view, supporting consistent, accurate analytics and data-driven decision-making across business functions.
- 4. Modern data management tools, including serverless architectures and edge computing, enhance flexibility, scalability, and cost-efficiency, enabling organizations to handle complex data needs seamlessly.
- 5. Adopting best practices in both ingestion and integration—such as data quality checks, data mapping, and robust governance—ensures data remains valuable, consistent, and actionable.
Understanding data ingestion
Data ingestion is the critical first step in a data pipeline, acting as the gateway for all incoming data into an organization’s data ecosystem. This process involves transporting data from multiple disparate sources and loading it into a centralized repository or storage system, such as a data lake or data warehouse. Whether the data originates from structured databases, real-time IoT device feeds, or unstructured sources like documents and social media content, data ingestion ensures that all relevant information is collected and accessible in one location for future processing, analysis, or storage.
By centralizing data from diverse origins, ingestion lays the groundwork for any subsequent data operations, including integration, transformation, and analysis. It essentially prepares raw data, making it available for organizations to derive insights, optimize processes, and make data-informed decisions.
Core elements of data ingestion
- Source diversity: Data ingestion supports a range of data sources, including structured data (such as databases and spreadsheets), semi-structured data (like JSON logs and XML files), and unstructured data (emails, images, PDFs, etc.). This diversity allows organizations to gather a comprehensive dataset reflecting all aspects of their operations. Consolidating data from these varied origins is essential for organizations that need a holistic view of their processes, customers, and systems.
- Flexible data pipelines: Data pipelines form the backbone of ingestion, enabling automated data flows from source to destination. These pipelines ensure data handling is continuous and reliable, which is especially important in high-volume environments where even slight interruptions could lead to data loss or inaccuracies. Flexible pipelines are designed to adapt to growing data volumes and new sources, making it easy for organizations to scale as their data needs evolve without compromising on data speed or integrity.
- Real-time vs. batch ingestion: Data ingestion can operate in real time or in batches, each with unique advantages suited to specific applications. Real-time ingestion supports applications that require immediate data availability, such as IoT monitoring, fraud detection, or personalized customer experiences. Batch ingestion, on the other hand, groups large volumes of data and processes them at scheduled intervals, making it ideal for less time-sensitive tasks, such as compiling daily sales reports or monthly financial audits. Balancing real-time and batch ingestion allows organizations to address both immediate and periodic data needs efficiently.
- Data staging and storage: In some ingestion workflows, data staging serves as a temporary holding area where incoming data is validated, cleansed, and pre-processed before reaching its final destination. Staging ensures that only high-quality, validated data moves on to further processing stages, which reduces errors downstream and optimizes data consistency across systems. Staging is particularly beneficial for large organizations that rely on high data quality for regulatory compliance, reporting accuracy, and decision-making.
Establishing a robust data ingestion strategy enables businesses to maintain a steady, reliable flow of data into their systems, providing a solid foundation for effective data management. This approach not only enhances the quality and usability of data but also supports scalability, setting up organizations for long-term data success.
"Data ingestion is the critical first step in a data pipeline, acting as the gateway for all incoming data into an organization’s data ecosystem."
Understanding data integration
Data integration is a pivotal process in data management that combines, transforms, and enriches data from multiple sources to create a unified, coherent, and analysis-ready view. By consolidating data, integration ensures that all information across an organization is aligned, accessible, and consistent, allowing teams to rely on a single source of truth. This step is fundamental for enabling analytics, supporting data-driven operational decisions, and promoting a culture of informed decision-making across the organization. Through integration, businesses bridge data silos, eliminate redundancies, and enable seamless data flows across various applications and departments, allowing data to become an organization-wide asset.
Effective data integration transforms raw data from disparate sources into a well-organized, accurate resource, facilitating more sophisticated analytics, streamlined operations, and strategic insights. Whether for business intelligence, reporting, or real-time decision-making, integrated data empowers businesses to harness the full potential of their data assets.
Core elements of data integration
- Data transformation: Integration frequently involves data transformation processes that include cleaning, normalizing, and converting data into a consistent format. This transformation is crucial for eliminating discrepancies and aligning data across different sources, ensuring it is accurate and analysis-ready. By applying transformation rules, organizations can enhance data quality, reduce errors, and make it easier to interpret and analyze data across platforms, ensuring reliable outputs for reporting and analysis.
- Creating unified views: One of the primary goals of data integration is to create a single, unified view of business data. This unified view allows organizations to achieve a holistic understanding of operations, customer interactions, financials, and other business activities. For instance, combining customer data from sales, support, and marketing channels creates a 360-degree view of customer behavior and preferences, enabling targeted and personalized marketing, improved customer service, and a stronger customer relationship management (CRM) system. Unified views not only improve decision-making but also eliminate duplicate data entry and errors that arise from multiple data versions.
- Enhancing interoperability: Data integration promotes interoperability by ensuring that data is compatible across various applications, systems, and teams. By aligning data formats, structures, and accessibility, integration allows data to flow smoothly between departments and systems, eliminating friction and bottlenecks. This interoperability supports faster transitions between processes, reduces operational delays, and helps maintain data consistency. For example, a company’s CRM and ERP (enterprise resource planning) systems can share data, ensuring that customer order information is immediately visible to both sales and production teams, enabling prompt fulfillment and customer satisfaction.
Through effective data integration, organizations can eliminate isolated data silos, streamline operational workflows, and enhance the reliability of their business insights. By combining data from across the enterprise into a single, actionable view, data integration maximizes the value of data and supports strategic initiatives, fostering a data-driven culture across the organization.
Key differences: Data ingestion vs. data integration
Data ingestion and data integration play complementary roles in an organization’s data ecosystem, each with distinct objectives that support a structured and meaningful data flow. Data ingestion is the initial step responsible for gathering raw data from multiple sources and centralizing it in a storage environment such as a data lake or data warehouse. This process prioritizes speed and completeness, ensuring that all relevant data is accessible, whether for immediate analysis or later processing. By establishing a robust pipeline, data ingestion allows businesses to capture and store valuable information in real-time or in batches, supporting various data-driven applications and historical data archiving.
In contrast, data integration focuses on transforming this raw data into a unified, structured format ready for analysis and decision-making. This step involves more complex processes such as cleansing, standardization, deduplication, and merging, ensuring that data from different sources aligns and maintains quality standards. By creating a consistent and reliable dataset, data integration supports critical business functions, including reporting, business intelligence, and operational insights. Together, data ingestion and integration create a streamlined flow from raw data collection to actionable intelligence, enabling organizations to harness data more effectively and make informed decisions.
Aspect | Data ingestion | Data integration |
---|---|---|
Purpose | Collecting and centralizing data from multiple sources for storage. | Transforming and unifying data to create a consistent and analysis-ready dataset. |
Process | Transfers raw data from origin to storage with minimal processing. | Involves cleansing, standardization, deduplication, and merging of data from multiple sources. |
Timeliness | Supports real-time or batch data processing, often used for immediate data availability. | Primarily operates post-ingestion to ensure data is fully processed and ready for analysis. |
Use cases | Data lakes, data warehouses, data hubs, and backup storage. | Business intelligence, analytics, reporting, operational insights, and cross-functional applications. |
Outcome | Provides raw, centralized data available for downstream processing or storage. | Generates consistent, deduplicated, and analysis-ready data, prepared for immediate use in applications. |
Data quality | May include basic validations to ensure format consistency. | Enforces strict data quality checks, standardizing and correcting errors to ensure reliability for decision-making. |
Complexity | Generally simpler, focusing on moving data with limited transformation. | Involves complex transformations, requiring advanced data mapping and alignment across systems. |
Frequency | Can be continuous or scheduled in batches depending on data source requirements. | Often scheduled periodically, with intervals based on the need for updated, unified datasets. |
The primary distinction lies in their focus: data ingestion is concerned with moving data into a centralized environment from various sources, while data integration focuses on refining and organizing this data to ensure consistency, accuracy, and accessibility. Ingestion supports a robust data pipeline by ensuring all relevant data is present, while integration builds upon this pipeline, transforming the raw data into structured, analysis-ready information.
Both processes also differ in timing and stages. Data ingestion encompasses obtaining and importing data for immediate use or storage. Integration, however, occurs after ingestion and involves processes like cleaning, deduplication, and transformation, ensuring data readiness for business applications.
Ultimately, data ingestion and data integration serve complementary purposes. Ingestion enables a steady flow of data into the ecosystem, while integration refines and structures it to become actionable information. Together, they are foundational to any data-driven strategy, allowing businesses to streamline data flow, enhance data visibility, and support informed decision-making. These processes ensure that data not only reaches the organization but also becomes meaningful, accurate, and valuable in guiding insights and strategic actions.
Common use cases for data ingestion and integration
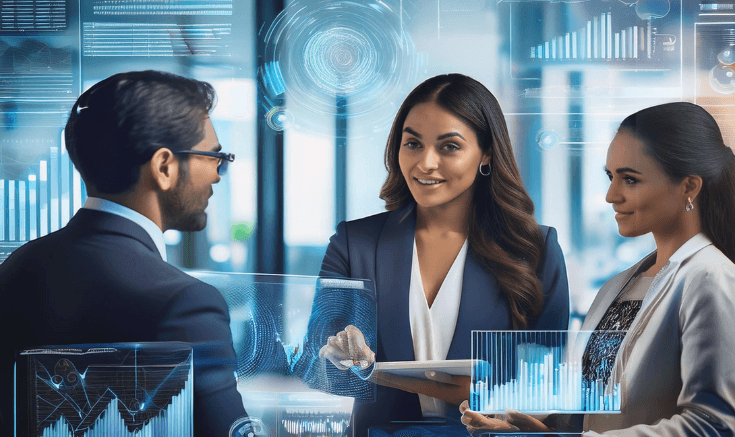
Data ingestion and integration are critical for driving operational efficiency, enhancing customer experiences, and generating actionable insights across various industries. Each use case leverages these processes to address unique data needs and maximize the impact of data-driven decisions, making them indispensable for organizations aiming to optimize and innovate.
Data ingestion use cases
Data ingestion pipelines are crucial for organizations to collect, process, and analyze data from various sources providing essential insights for operational monitoring, customer engagement, and strategic planning. By efficiently collecting data from various points, businesses gain a foundational layer of information to support timely decisions and preventative actions.
- Industrial equipment monitoring: IoT systems continuously capture data on equipment status, performance, and maintenance needs, allowing for predictive maintenance and reduced downtime.
- Customer data analysis: In retail and e-commerce, data ingestion gathers customer interactions from social media, websites, and support platforms to offer personalized experiences and targeted marketing.
- Financial markets analysis: Real-time data ingestion enables financial institutions to analyze market trends instantly, supporting trading decisions, risk assessment, and competitive positioning.
- Healthcare patient data collection: Hospitals and clinics use data ingestion to gather patient information from multiple devices and databases, centralizing it for faster diagnosis and treatment.
- Supply chain visibility: Manufacturers and logistics providers capture data from various nodes in the supply chain to track stock levels, demand fluctuations, and potential bottlenecks.
By supporting continuous data flow, data ingestion provides organizations with timely access to critical information across industries, allowing them to respond rapidly to changes, improve operational efficiency, and enhance customer engagement.
Data integration use cases
Data integration transforms raw, disparate datasets into cohesive, analysis-ready information, enabling businesses to unlock meaningful insights for decision-making and long-term strategic planning. Integration empowers organizations to combine data across systems, producing a unified view of operations and customer interactions.
- Customer 360 insights: Integrating data from sales, customer service, and social media gives businesses a comprehensive view of customer preferences and behavior, supporting personalized service and increased satisfaction.
- Healthcare interoperability: Combining patient records from various departments enables coordinated healthcare delivery, reduces diagnostic errors, and improves patient outcomes.
- Inventory and demand planning: Integrating data from inventory systems, sales channels, and supplier databases supports proactive inventory management, reducing stockouts or overstock.
- Employee performance analytics: HR departments integrate performance data from multiple tools to analyze workforce productivity, enhancing workforce planning and development.
- Marketing attribution: Integrating data from advertising, social media, and website interactions allows businesses to understand which channels drive conversions, optimizing marketing spend and strategy.
Through these integration processes, organizations gain a unified, high-quality data layer that informs strategic actions, supports operational coherence, and drives insights across functions and customer journeys.
"Effective data integration transforms raw data from disparate sources into a well-organized, accurate resource, facilitating more sophisticated analytics, streamlined operations, and strategic insights."
Best practices for data ingestion
Implementing structured best practices for data ingestion is essential for maintaining smooth, accurate data flows and ensuring that raw data is prepared for meaningful use without interruptions. A robust ingestion framework minimizes errors, enhances data quality, and supports scalability as data volumes grow, creating a solid foundation for all downstream processes.
Clearly define data sources and formats
Start by identifying all data sources, their structures, and the expected data formats. This preparation enables teams to set up efficient ingestion pipelines tailored to different types of data, whether structured, semi-structured, or unstructured. Clearly defined source types and formats also reduce the risk of data mismatches, inconsistencies, and potential errors, ensuring a smoother ingestion process.
Establish real-time and batch ingestion strategies
Aligning data ingestion strategies with business needs helps maximize the value of data. Real-time ingestion is essential for time-sensitive applications, such as monitoring IoT devices or tracking live customer interactions, while batch ingestion is better suited for less urgent tasks that involve processing larger volumes, like daily sales reporting. Defining when and where to use each approach enables organizations to optimize resources and meet their specific data requirements.
Implement data quality checks
Data validation at the ingestion stage is crucial to prevent errors from propagating down the pipeline, which can compromise data accuracy and lead to flawed analyses. Quality checks might include verifying data completeness, accuracy, and format consistency, ensuring that only valid data proceeds through the pipeline. Data quality measures at the ingestion point reduce rework and protect data integrity across the system.
Monitor and optimize pipeline performance
Regular performance evaluations help maintain the stability of data ingestion pipelines. Monitoring metrics such as ingestion speed, latency, and error rates enables teams to identify bottlenecks or inefficiencies. Adjusting configurations and refining pipeline components as needed ensures that data is ingested quickly and reliably, even as data volumes increase.
Ensure compliance with data governance
Data governance is especially important when handling sensitive information, such as personal health data or financial transactions. Ensuring compliance with regulations like GDPR, HIPAA, or CCPA involves embedding privacy and security standards within the ingestion process. This practice not only minimizes compliance risks but also builds trust with users and stakeholders, as data is managed responsibly from the outset.
A well-managed ingestion pipeline is scalable, reliable, and adaptable, enabling businesses to accommodate growing data volumes without sacrificing quality or performance. By following these best practices, organizations can establish a robust ingestion framework that delivers high-quality, ready-to-use data, setting the stage for effective data integration and analytics.
Best practices for data integration
Successful data integration establishes consistency and cohesion across various data sources, enabling organizations to perform effective analysis and derive actionable insights. By following structured integration practices, businesses can reduce data discrepancies, enhance reliability, and maintain high data quality across their systems.
Data mapping and standardization
Defining data relationships and mapping fields in advance is essential for creating a structured integration framework. This involves identifying how data fields from different sources correspond with each other and establishing standard formats for dates, units, and other values. Standardization reduces inconsistencies across datasets and simplifies data merging, making it easier to interpret and analyze integrated data. It also ensures that data remains compatible across applications, supporting accurate and efficient analytics.
Automate transformation processes
Automating repetitive tasks, such as data cleansing, deduplication, and normalization, improves efficiency and reduces the risk of human error. Automation can be applied to detect and correct common issues, such as formatting errors or duplicate entries, saving time and enhancing data quality. This approach allows data teams to focus on higher-value activities, such as strategic analysis, while ensuring that integrated data remains accurate and consistent.
Prioritize data quality checks
Maintaining data accuracy and completeness throughout the integration process is crucial for ensuring reliable analysis and trustworthy insights. Implementing regular quality checks, such as validation rules for accuracy and consistency, helps detect errors early in the process, reducing the risk of compromised data. Quality checks also ensure that integrated data aligns with business needs, building confidence in data outputs and supporting informed decision-making across the organization.
Incorporate metadata for tracking and traceability
Metadata provides essential context to integrated data, helping users understand data origins, transformation steps, and usage history. By including metadata, organizations improve data governance, enabling users to trace data lineage and verify its accuracy and relevance. Metadata also enhances data management transparency, facilitating compliance with regulatory requirements and building trust in data quality across departments.
Implement robust data governance
Establishing clear data governance policies and procedures safeguards data quality, privacy, and regulatory compliance. These policies define roles and responsibilities, data access controls, and data handling practices, ensuring data integrity and security throughout the integration process. Governance measures also include regular audits and updates to keep policies relevant as data volumes and regulatory landscapes evolve. A strong data governance framework promotes consistency and accountability, ensuring that data remains valuable and compliant across the organization.
These best practices create a cohesive integration framework, ensuring that data remains accessible, reliable, and actionable across business functions. By standardizing processes, prioritizing quality, and maintaining robust governance, organizations can optimize their data integration practices and build a trusted data foundation that supports analytics, decision-making, and operational efficiency.
The role of ingestion and integration in the data lifecycle
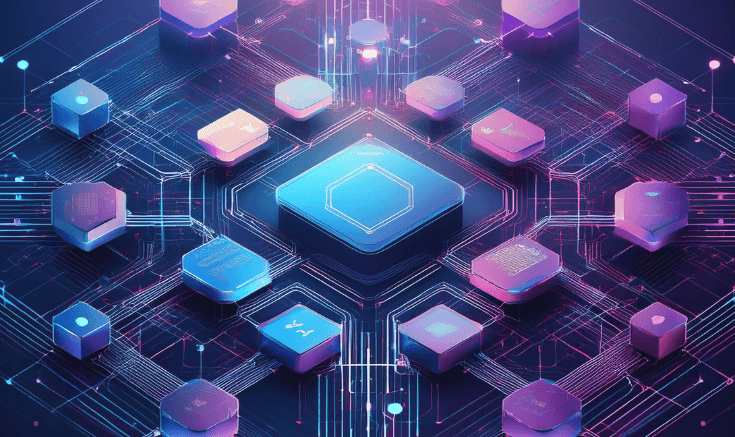
Data ingestion and integration are fundamental stages within the data lifecycle, working together to ensure that data is accessible, usable, and valuable from its initial capture to long-term storage. Beginning with data collection and ingestion, data flows into the system, centralizing information from diverse sources. This stage organizes data for immediate processing or storage, setting the foundation for consistent and reliable data handling across the organization.
Following ingestion, transformation and integration processes standardize, cleanse, and merge data, creating a cohesive dataset that is ready for analysis. Integration removes inconsistencies and combines data into a single view, ensuring it aligns with business standards and goals.
The prepared data then enters the analytics and insight generation stage, where it supports various analytics initiatives. At this point, data feeds into dashboards, predictive models, and reporting tools, providing the insights that drive strategic decision-making. Accurate, integrated data at this stage empowers organizations to make informed choices, optimize operations, and respond proactively to emerging trends.
Finally, data reaches the storage and archiving stage, where it is saved or archived to ensure availability for future use. This process maintains data integrity across systems, preserving valuable information for historical analysis, regulatory compliance, or future reference.
Each stage in the lifecycle maximizes the value of data, transforming raw information into actionable insights and enabling data-driven decision-making. Together, ingestion and integration ensure that data not only enters the system but also flows seamlessly through the organization, supporting operational efficiency and strategic growth.
"These processes ensure that data not only enters the system but also flows seamlessly through the organization, supporting operational efficiency and strategic growth."
Future trends in data ingestion and integration
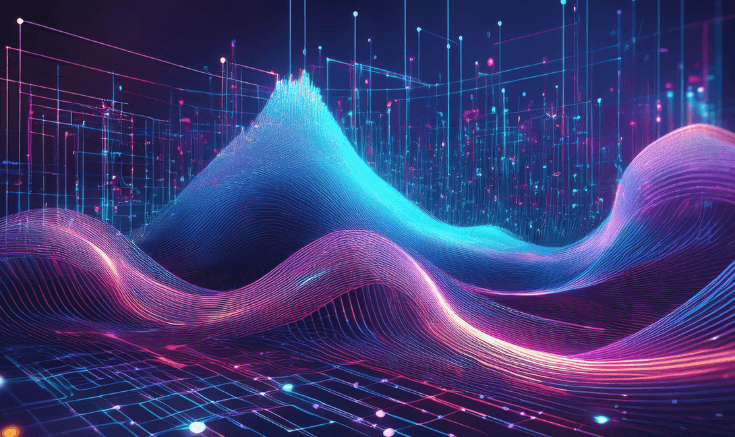
The future of data ingestion and integration is rapidly evolving as new technologies and methods emerge to meet the increasing demands of data-driven organizations. Here are five key trends shaping this landscape:
- AI-powered automation: Machine learning and artificial intelligence are transforming data workflows, automating processes in both ingestion and integration. By leveraging algorithms to handle repetitive tasks, organizations improve data accuracy, reduce manual effort, and minimize errors, resulting in faster, more reliable data flows.
- Edge computing and decentralized processing: As IoT devices proliferate, more data processing is happening closer to the data source. This decentralized approach, known as edge computing, reduces latency and allows for real-time responses. Processing data at the edge is crucial in applications like predictive maintenance or real-time monitoring, where immediate insights are essential.
- Data observability and monitoring: Maintaining data quality and visibility has become a priority as data pipelines grow in complexity. Data observability enables organizations to monitor pipeline health, track data lineage, and identify any potential issues early on. By enhancing transparency and control, observability strengthens data trustworthiness and supports compliance with regulatory standards.
- Serverless architecture: Serverless solutions for data ingestion and integration are gaining popularity due to their scalability and cost efficiency. With a serverless approach, organizations can handle fluctuating data volumes without the burden of infrastructure management, allowing for flexible scaling and budget optimization. This architecture is particularly attractive for businesses with variable data workloads.
- Real-time data fusion: Merging real-time and historical data enables organizations to create comprehensive, timely insights that enhance decision-making. This trend is especially relevant for industries like finance, healthcare, and retail, where immediate access to data insights is crucial. Real-time data fusion empowers businesses to act quickly on current data while leveraging historical patterns to make informed decisions.
These trends represent a shift toward more agile, intelligent, and resilient data processes, equipping organizations to better manage their data and remain competitive. Embracing these advancements will allow businesses to future-proof their data strategies, improving both the quality and speed of their data insights.
In understanding data ingestion vs. data integration, it's clear that both processes are vital components of a robust data strategy. Each serves unique functions and benefits across the data lifecycle, from ingestion's role in capturing raw data to integration's focus on transforming and unifying data for analysis. Leveraging best practices, appropriate tools, and future-forward trends in both areas will empower businesses to stay competitive and drive meaningful insights.
For businesses seeking a partner who combines technical expertise with a commitment to tailored outcomes, Lumenalta offers a unique approach. As a trusted tech operative, we work alongside clients to develop transformative data solutions through radical engagement, ensuring each step—from ingestion to integration—aligns seamlessly with your strategic goals.
Table of contents
- Understanding data ingestion
- Understanding data integration
- Key differences: Data ingestion vs. data integration
- Common use cases for data ingestion and integration
- Best practices for data ingestion
- Best practices for data integration
- The role of ingestion and integration in the data lifecycle
- Future trends in data ingestion and integration
- Common questions about data ingestion vs. data integration
Common questions about data ingestion vs. data integration
What is the main difference between data ingestion and data integration?
How do real-time and batch processing work in data ingestion?
Why is data quality important in data ingestion and integration?
What are the benefits of serverless architecture for data ingestion and integration?
How does data observability enhance data ingestion and integration?
Want to learn how data integration can bring more transparency and trust to your operations?