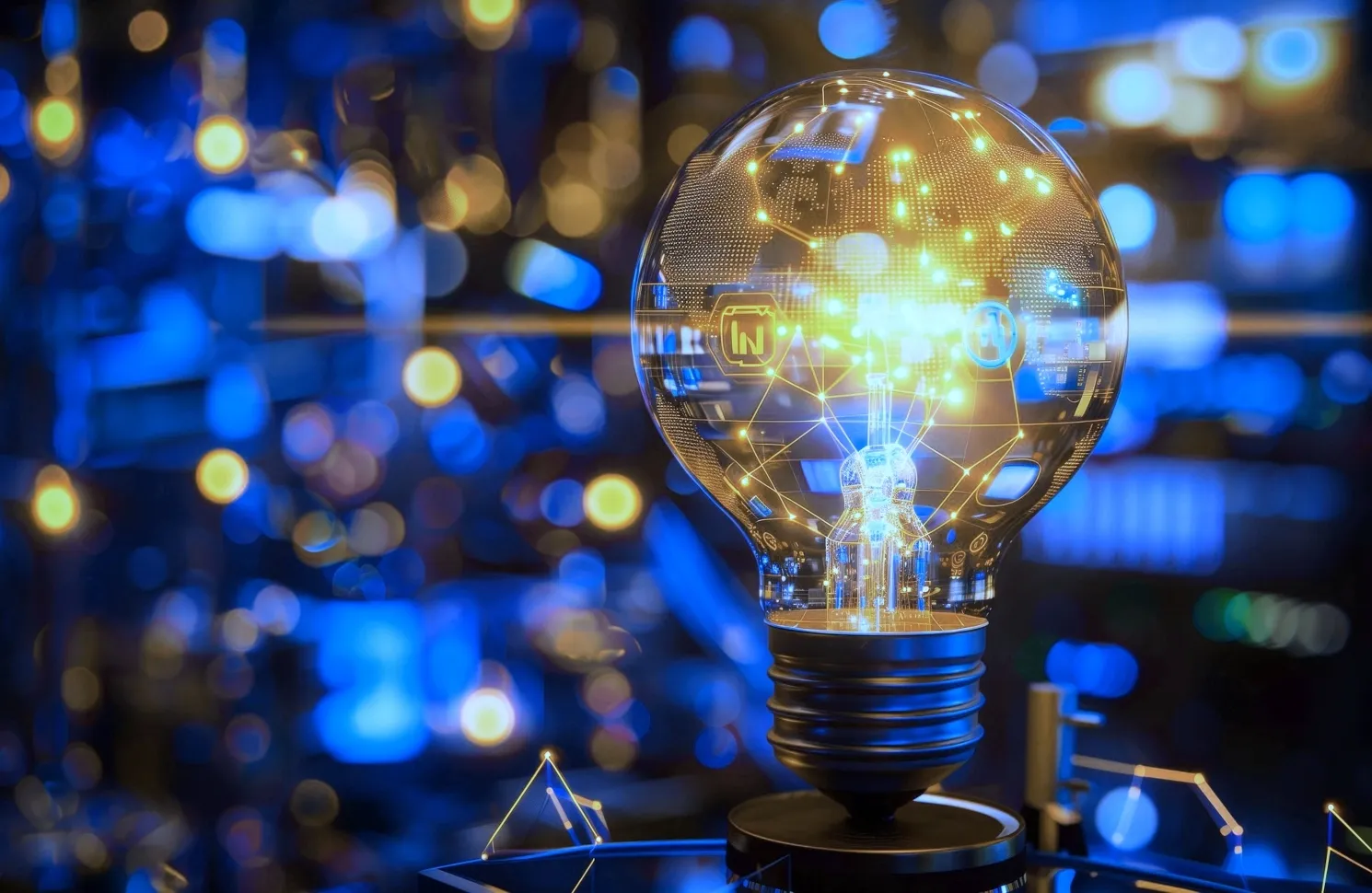
How legacy data can accelerate your progress with AI
AUG. 9, 2024
2 Min Read
Your legacy data is your secret weapon. Here’s how to use it.
In a stunning display of technological collaboration, Elon Musk has announced the activation of the ‘Memphis Supercluster,’ a powerful AI training cluster. Developed by teams from xAI, X, Nvidia, and supporting companies, this facility boasts 100,000 liquid-cooled H100s on a single RDMA fabric. Musk claims it’s ’the most powerful AI training cluster in the world.’
This breakthrough isn’t just a headline; it’s a wake-up call for businesses across the spectrum. You might be thinking, “That’s impressive, but what does it mean for my organization?” The answer may surprise you: You’re likely sitting on an untapped goldmine that could propel your AI initiatives forward faster than you ever imagined.
The secret? Your legacy data.
The AI revolution is here, and it’s moving at breakneck speed. But with the right approach, your organization can not only keep pace but potentially lead the charge. Let’s explore how you can turn your legacy data into a powerful catalyst for AI innovation.
The rapid pace of AI advancement
The xAI supercomputer example isn’t just flashy — it’s a testament to the speed at which AI technology evolves. Here’s what this means for mid-market and enterprise businesses:
- Unprecedented processing power: While exact performance metrics aren’t confirmed, the sheer scale of 100,000 liquid-cooled H100s represents a significant leap in processing capability.
- Efficient data movement: The single RDMA fabric highlights the importance of optimized data transfer in large AI systems.
- Democratization of AI: As technology trickles down, mid-market companies will find increasingly powerful AI tools within reach.
- Competitive pressure: Companies delaying AI initiatives risk falling behind rapidly.
- New possibilities: This level of computing power opens up new avenues for businesses across all sectors.
For businesses, the message is clear: AI implementation is an immediate opportunity, not a far-off consideration. However, this rapid pace presents challenges.
How can companies keep up without overextending their resources? The key lies in smartly leveraging existing assets — particularly your data.
Leveraging legacy data for AI advancement
Mid-market and enterprise businesses have a powerful, often overlooked asset: legacy data. This treasure trove of historical information can catalyze rapid AI progress within your organization.
Donovan Crewe, Sr. Software Architect at Lumenalta, emphasizes the importance of legacy data: “Legacy data often requires some form of preprocessing or normalization in order for it to be usable by models or for training. With more advanced models being released, the ease of ’cleaning up’ legacy data becomes a much easier task.”
Legacy data provides a wealth of real-world, company-specific information for training AI models. These datasets often contain years of valuable insights about customers, operations, and market trends. By leveraging this data, you can create AI models tailored to your specific business context from day one.
AI excels at identifying patterns that humans might miss, potentially revealing new business opportunities or operational efficiencies.
Historical data is the foundation of predictive analytics. By training AI models on your legacy data, you can create powerful predictive tools that forecast future trends based on your company’s unique historical patterns. This can lead to more accurate demand forecasting, risk assessment, and strategic planning.
AI models trained on your legacy data can hit the ground running, significantly reducing the time-to-value for your AI initiatives.
Utilizing existing data is often more cost-effective than acquiring new data or building expansive new datasets. It allows you to maximize the value of resources you already possess. Moreover, your legacy data already exists within your company’s compliance and security frameworks, simplifying the process of implementing AI solutions.
Case study: Financial services
Consider a mid-sized financial institution that used its legacy transaction data to develop an AI-powered fraud detection system. By training the AI on years of historical transaction data, including known instances of fraud, the institution created a highly accurate model that could identify potential fraud in real time. This improved security, enhanced customer trust, and reduced operational costs.
To implement this strategy:
- Conduct a thorough audit of your legacy data.
- Invest in tools and processes to clean and standardize your data.
- Start with a pilot project using a subset of your legacy data.
- Ensure your data infrastructure can handle increased demands as you expand.
- Implement systems for continuous learning and improvement.
By strategically leveraging your legacy data, you can accelerate your AI initiatives, gaining a competitive edge without massive upfront investments in new data collection or cutting-edge hardware.
The importance of data infrastructure
While leveraging legacy data can jumpstart your AI initiatives, a robust data infrastructure is crucial to enabling AI success and long-term digital transformation.
Data infrastructure encompasses the technologies, systems, and practices that allow your organization to collect, store, process, and analyze data effectively. In the context of AI, this infrastructure needs to be agile, scalable, and capable of handling the complex demands of modern machine learning algorithms.
Many organizations face common challenges with their existing data infrastructure:
- Siloed legacy systems
- Data quality issues
- Inconsistent formats and lack of standardization
- Outdated storage and processing capabilities
Improving and modernizing your data infrastructure is critical. Key components include:
- Implementing a centralized data lake or data warehouse
- Adopting cloud-based solutions for scalability and flexibility
- Establishing clear data governance policies
- Implementing real-time data processing capabilities
- Utilizing metadata management and data cataloging tools
It’s important to note that modernizing your data infrastructure doesn’t necessarily mean discarding all legacy systems. Often, the goal is to create a hybrid environment where legacy systems are integrated with modern data platforms.
Let’s use the case of a mid-sized manufacturing company that invested in modernizing its data infrastructure before launching AI initiatives for example. By integrating data from its production lines, supply chain, and customer feedback systems into a unified data platform, the company developed AI models that optimized production schedules, predicted maintenance needs, and improved product quality.
The initial investment in infrastructure paid dividends in increased efficiency, reduced downtime, and improved customer satisfaction.
Balancing AI progress with data management
Sustainable AI success requires a delicate balance between pushing the boundaries of AI capabilities and maintaining robust data management practices. This balance ensures that AI initiatives are built on a solid foundation that can support long-term growth and adaptation.
Strategies for balancing AI progress and data management:
- Adopt an iterative process where AI projects and data management initiatives inform and enhance each other.
- Prioritize data governance alongside AI development.
- Foster collaboration between data management teams and AI development teams.
- Implement ethical AI practices addressing bias, privacy, and transparency.
- Invest in tools and technologies that support both AI development and data management.
Neglecting data management in favor of rapid AI progress can lead to significant risks:
- Biased or inaccurate AI models
- Flawed decision-making
- Damaged customer trust
- Data security breaches
- Compliance violations
- Difficulty scaling AI initiatives
A balanced approach offers numerous long-term benefits:
- More accurate and reliable AI models
- Greater agility in responding to new opportunities or challenges
- Unlocking new AI use cases through improved data accessibility
Take, for example, a retail company that balanced its AI initiatives with data management improvements. As they developed an AI-powered personalized recommendation system, they simultaneously invested in enhancing their customer data platform.
This dual approach resulted in more accurate recommendations and enabled the company to quickly adapt the system for other applications, such as inventory management and marketing campaign optimization.
Related: AI in retail and ecommerce
Expert insight: Latest AI technology releases
Staying informed about the latest technological advancements is crucial for business leaders. One of the most significant recent developments is the release of new large language models, such as the Llama 3.1 models from AI at Meta.
The Llama 3.1 405B model, in particular, stands out as one of the largest publicly available foundation models, setting a new standard for AI in enterprise applications and research and development. Its capabilities extend beyond basic language tasks to include advanced functions such as synthetic data generation and model distillation.
Crewe highlights the significance of these new releases: “The release of models like Llama 3.1 405b really eases the integration of AI into businesses that may have been blocked by language or context limitations. The generation of synthetic data is a great addition especially to assist businesses who have scarce, high quality training data.”
For businesses, the ability to customize and optimize these models for specific use cases is a game-changer. It allows companies to tailor AI solutions to their unique needs without starting from scratch, potentially saving significant time and resources in AI development.
The availability of these advanced models on cloud platforms makes them more accessible to a wider range of businesses. This democratization of AI technology means that even mid-market companies can now leverage state-of-the-art AI capabilities that were previously only available to tech giants.
Crewe notes, “I think the adoption rate of models depends on how easily accessible a cloud version is. OpenAI has proven this by making ChatGPT available to the average person, which has led to a surge in AI’s popularity.”
While these advancements are exciting, businesses need to approach them strategically. Not every company needs the most advanced AI model for their applications. The key is to align the AI technology with specific business goals and use cases.
Actionable steps for executives
- Assess your current state: Conduct a thorough assessment of your organization’s AI readiness and data capabilities.
- Develop a data-centric AI strategy: Create a comprehensive strategy that aligns your AI goals with your overall business objectives.
- Invest in data infrastructure: Allocate resources to modernize your data infrastructure.
- Start with pilot projects: Identify specific business problems that AI could address and start with small-scale pilot projects.
- Foster cross-functional collaboration: Break down silos between your data management, IT, and business teams.
- Prioritize continuous learning: Invest in ongoing training and development to keep your team updated on the latest AI technologies and best practices.
- Implement ethical AI practices: Establish clear guidelines for the ethical use of AI in your organization.
- Leverage cloud platforms and pre-trained models: Explore how you can use cloud-based AI services and pre-trained models to accelerate your AI initiatives.
- Monitor and iterate: Implement systems to continuously monitor the performance of your AI models and data quality.
- Plan for scale: Develop a roadmap for scaling successful AI solutions across your organization.
Crewe offers this advice to executives: “Start now. The sooner you start integrating AI in your organization, the easier it will be to benefit from the optimization and insights it has to offer.”
The AI revolution is not a distant future — it’s happening now and it’s moving faster than many anticipated. However, the message is clear: you don’t need to build a supercomputer or hire an army of AI experts to start benefiting from AI. The key lies in leveraging your existing assets, particularly your legacy data, while simultaneously strengthening your data management practices.
“Embracing AI will benefit companies by optimizing support, providing future insights based on past data, and delivering products to market faster. This will offer a competitive advantage over companies that avoid AI. I recently read that AI is the electricity of our age. There were people who feared electricity and those who embraced it. I recommend embracing it,” says Crewe.
By following these actionable steps, you can position your organization to take advantage of AI’s transformative potential.
Remember, the goal is not just to implement AI for its own sake, but to use it as a tool to drive real business value, whether that’s through improved efficiency, enhanced customer experiences, or new product innovations.