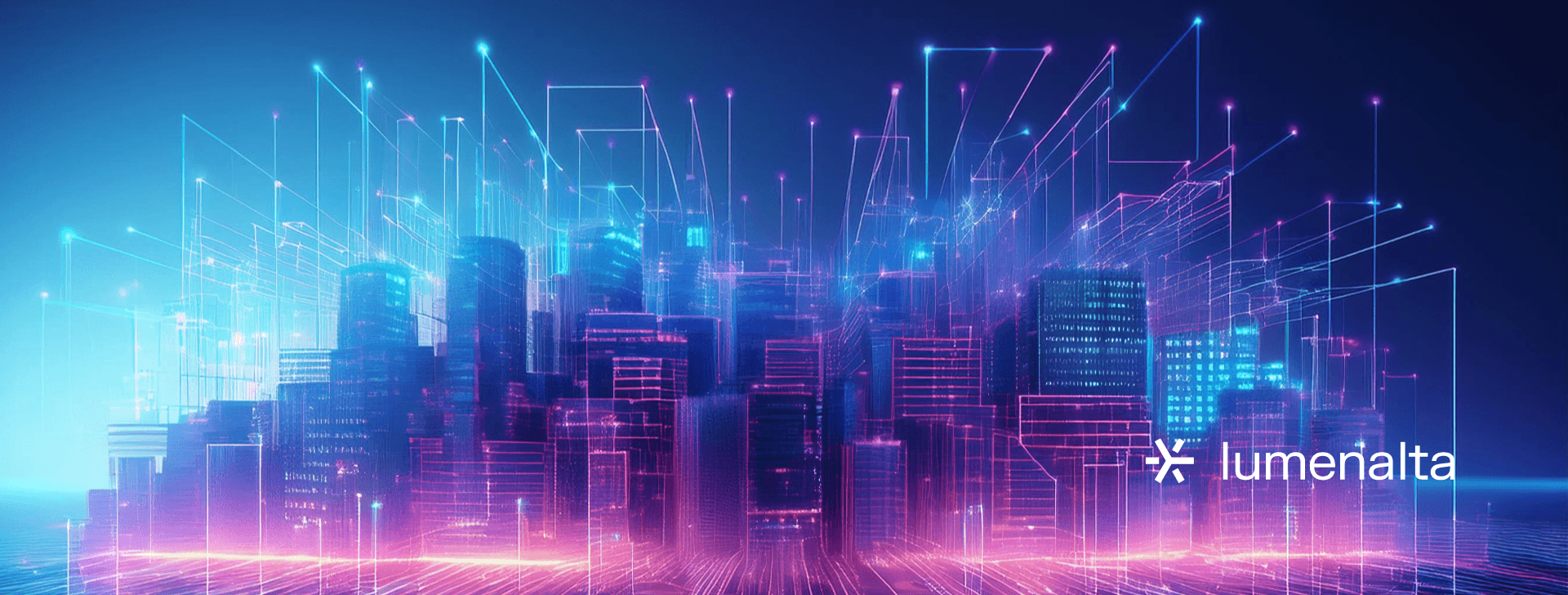
AI due diligence checklist (updated 2025)
FEB. 11, 2025
6 Min Read
AI is integral to modern initiatives geared toward cost efficiency, improved scalability, and new revenue streams.
Many organizations see it as a direct route to greater impact, yet the process of assessing algorithms, data pipelines, and compliance policies can feel overwhelming. A methodical AI due diligence checklist can turn that uncertainty into clarity, refining your path to market and revealing untapped business potential. Every angle counts, whether it involves verifying data quality or ensuring the technology aligns with ongoing objectives, giving you the confidence to move forward with concrete metrics in hand.
By establishing consistent evaluation steps, you can focus on what truly matters: generating measurable returns and reducing risk. An organized approach to AI assessments highlights exactly where to invest time and resources, trimming unnecessary overhead while strengthening accountability. The result is a more efficient pipeline, better stakeholder alignment, and less confusion surrounding responsibilities and performance benchmarks. Careful planning also helps you stay ahead of compliance hurdles, ensuring that AI solutions remain both secure and transparent at every phase.
Key takeaways
- 1. A structured evaluation of AI solutions highlights hidden risks and strengthens performance from the start.
- 2. Data quality, algorithm transparency, and stakeholder alignment are essential elements of any AI due diligence checklist.
- 3. Cost-effectiveness grows when security, scalability, and resource forecasting are addressed at every stage.
- 4. Transparent processes and pilot testing allow for early feedback and minimize project disruptions.
- 5. Continuous oversight builds confidence, reduces technical debt, and ensures machine learning projects maintain momentum over time.
Understanding AI due diligence
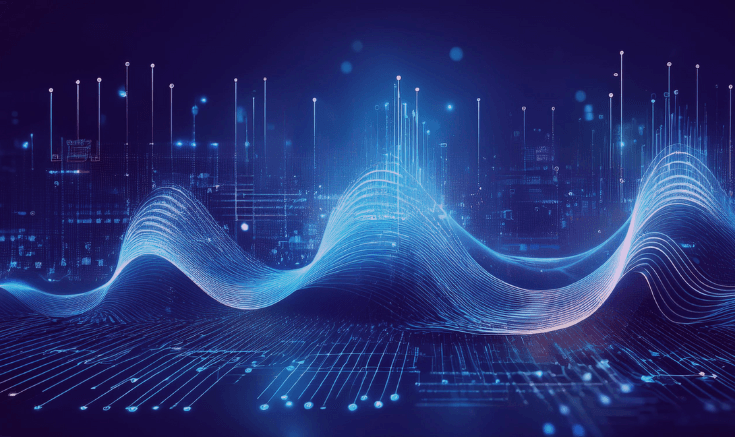
Enterprises that incorporate artificial intelligence solutions often face questions about reliability, risk, and potential ROI. AI due diligence focuses on a structured examination of algorithms, data pipelines, and compliance standards to ensure these solutions meet key performance benchmarks. This concept extends beyond verifying basic accuracy because it also considers scalability, transparency, and alignment with strategic objectives. A thorough evaluation can highlight potential pitfalls, leading to stronger governance and more efficient use of resources.
The process of assessing these systems has become a priority as data volumes and project complexities grow. A methodical AI due diligence checklist can assist your leadership teams in identifying issues early, reducing technical debt, and keeping time to value on track. This approach also reveals overlooked opportunities for optimization, especially if you aim to maximize returns or integrate new tools without delays. Some organizations treat this scrutiny as a continuous practice, refining methodologies over time and reinforcing confidence in AI investments.
“Enterprises that incorporate artificial intelligence solutions often face questions about reliability, risk, and potential ROI.”
Importance of AI due diligence
Enterprises rely on AI due diligence as a fundamental step for validating alignment with strategic targets, spotting potential cost savings, and ensuring accurate performance metrics. It helps you set realistic expectations regarding an AI project's scalability, security, and compliance requirements. This systematic approach uncovers hidden opportunities for process automation, advanced analytics, or improved governance. A thorough evaluation also gives you the confidence to move forward with implementation, knowing your investment stands on stable ground.
This practice clarifies the viability of new proposals and mitigates risk across cross-functional teams. A structured AI due diligence checklist, when introduced early, reduces the possibility of unwelcome surprises and project slowdowns. It shines a spotlight on overlooked data dependencies, integration blockers, and potential security oversights that might derail your initiatives. That level of clarity fosters stronger stakeholder engagement and a more reliable path to market.
Evolution of AI due diligence practices
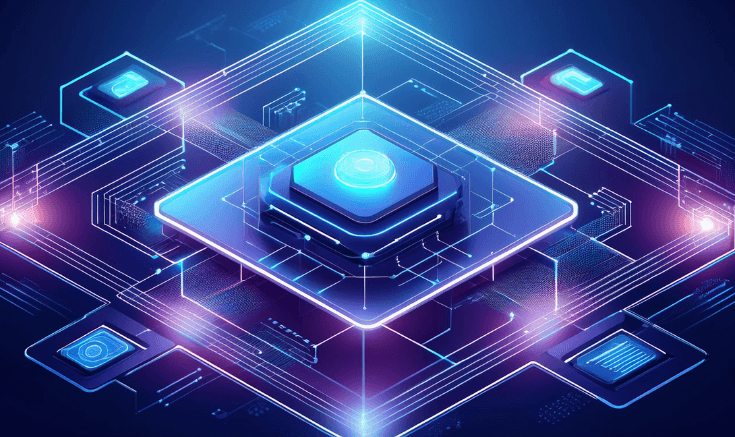
Organizations that explored machine learning a decade ago often had limited tools for assessing performance and compliance. Early guidelines centered on simple validation methods, but new frameworks demand thorough checks on data lineage, algorithm transparency, and security protocols. Analysts now incorporate advanced analytics to examine potential vulnerabilities and confirm alignment with business objectives. This expanded scope allows you to build more robust AI due diligence practices, ensuring that any identified gaps are addressed before major commitments are made. That evolution eventually led to the creation of an AI due diligence checklist, helping you evaluate factors like performance, accountability, and cost-effectiveness in a single framework.
- Early compliance checks to advanced governance frameworks: Technical evaluations once revolved around basic protocol adherence and data format compliance. Over time, organizations shifted to in-depth governance frameworks, examining everything from data provenance to privacy handling, thus laying the groundwork for more structured guidelines and tighter oversight.
- Ad hoc reviews to ongoing risk management: Early approaches often occurred on a project-by-project basis, with minimal follow-up or updates. Today’s practice emphasizes continuous oversight, where each component is reviewed at different intervals to identify new vulnerabilities, reinforce compliance, and guarantee smoother deployment cycles. This shift reduces unexpected interruptions and supports stable growth.
- Performance metrics to advanced analytics: Early AI audits placed a heavy focus on system accuracy without closely examining resource usage or hidden biases. Updated practices now incorporate advanced analytics to highlight patterns around data quality, bias mitigation, and computational overhead, ensuring your solutions remain both scalable and cost-effective.
- Reactive to proactive security checks: Previous evaluations addressed security concerns only after performance benchmarks were verified. Modern approaches prioritize security from the beginning, verifying data integrity and monitoring user access rights to reduce the possibility of compliance issues, legal liabilities, and costly remediation. This forward-thinking stance allows you to strengthen trust among stakeholders and prevent disruptions that could delay product rollouts.
- Single-discipline teams to cross-functional collaboration: Early AI reviews were often limited to data scientists, leaving gaps in legal, operational, and financial perspectives. Organizations now engage broader groups, ensuring that leadership, compliance officers, and finance teams contribute to discussions on risk tolerance, project feasibility, and cost management. That level of collaboration can accelerate deployment schedules and reduce friction between technical and business units.
- Minimal documentation to formalized reporting: Early AI evaluations rarely involved structured records, which complicated audits and slowed integration with enterprise systems. Present-day best practices emphasize thorough documentation, clarifying performance thresholds, data lineage, and error rates. This approach supports faster knowledge sharing and ensures that important information is retained for future reference. It also makes audits less disruptive, especially when seeking regulatory approval or external funding.
These shifts in methodology capture how AI due diligence practices have progressed into a more holistic framework. They lay the groundwork for a solid AI due diligence checklist that can meet the needs of varied stakeholders, align with specific business outcomes, and preserve confidence in system performance. Some organizations might aim to reduce overhead, enhance time to value, or seize untapped business potential, and these historical lessons provide helpful context. The next step involves identifying the key components of an AI due diligence checklist, focusing on practical steps that transform observations into tangible results.
Key components of an AI due diligence checklist
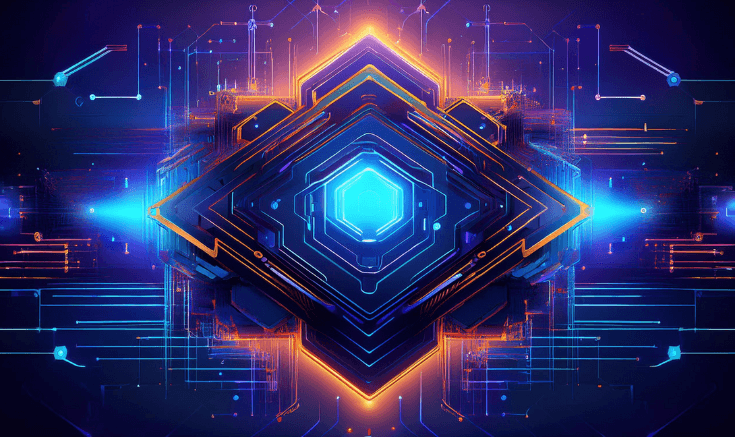
An effective AI due diligence checklist addresses a wide range of factors that collectively ensure reliable performance, regulatory compliance, and measurable results. This structured approach focuses on critical areas such as data quality, algorithm transparency, security protocols, and user adoption, offering a concrete way to manage risk. Every organization has unique strategic goals, yet certain recurring themes guide a thorough examination and promote consistency across different sectors. The list below highlights essential components that anchor your evaluations, helping you accelerate market entry and maximize returns.
- Data quality review: This element ensures that datasets are complete, up-to-date, and free from major inconsistencies or bias. Strong data hygiene can reduce error rates and operational inefficiencies, leading to more accurate predictions and quicker time to value. It also helps maintain compliance with privacy standards.
- Algorithm performance assessment: This step confirms that core models meet desired accuracy thresholds and can handle real-time processing workloads within your AI due diligence checklist. Steady monitoring and periodic testing also spot regression issues early, preventing costly mistakes. Frequent recalibration may be necessary as business objectives adjust.
- Security and compliance monitoring: This aspect emphasizes ongoing evaluations of data privacy regulations, encryption standards, and user access controls. Adhering to these guidelines protects intellectual property and safeguards customer trust while limiting legal liabilities. Proactive updates to security protocols can reduce disruptions down the line.
- Scalability and resource planning: This factor verifies the system’s ability to expand as project demands rise, particularly for organizations seeking broader market coverage. Careful capacity forecasting aligns with cost-effectiveness targets and spares you from sudden performance bottlenecks. Regular reviews also help refine infrastructure investments and play a vital role in any AI due diligence checklist that aims to reduce overhead.
- Ethical considerations: This area examines bias mitigation, transparency in model outputs, and respect for user data. Addressing these factors early fosters trust across different stakeholder groups and helps avoid brand-damaging controversies. Clear guidelines also set the stage for accountable AI usage.
- Governance and oversight: This point clarifies who is accountable for model updates, error handling, and strategic decisions involving AI adoption. Defined governance structures streamline communication and help you respond swiftly to shifting business requirements. Transparent leadership fosters consistency in how teams handle potential challenges.
- User adoption and stakeholder alignment: This section reviews training plans, support infrastructure, and user feedback loops to confirm that the technology meets real needs. Properly executed, it generates buy-in from leadership and frontline teams, driving higher overall impact. Encouraging open communication also boosts trust in the AI-based approach.
These essential elements shape an AI due diligence checklist that can adapt to different sectors, helping you identify risks early and capitalize on new growth potential. Reviewing each item in detail creates a cohesive AI strategy and secures stronger returns. A well-rounded assessment covers performance, compliance, and ethical parameters, minimizing hidden obstacles. After these core pillars are established, a step-by-step methodology offers deeper clarity, allowing you to allocate resources more intelligently while advancing your AI objectives.
Step-by-step AI due diligence checklist
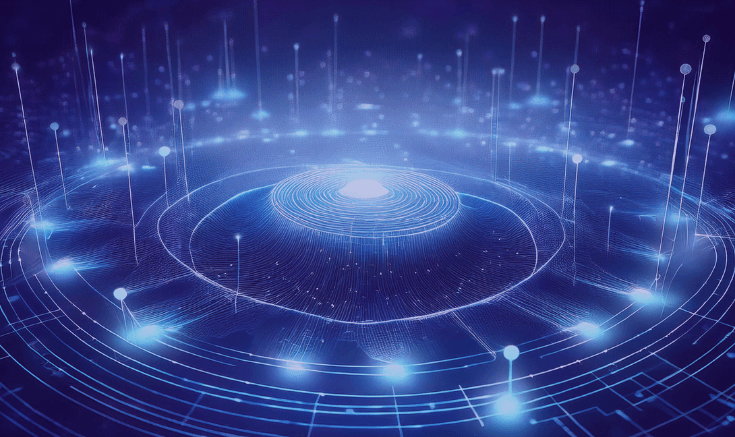
Projects involving machine learning can gain traction faster when guided by a clear plan. A structured approach provides momentum to identify risks, engage key stakeholders, and confirm that the technology fits with business goals. The objective is to map every stage, from defining a vision to tracking final outcomes, so you can minimize guesswork and strengthen implementation. This method adds clarity to your AI due diligence checklist, ensuring that each step aligns with your compliance obligations and performance targets.
- Identify the project scope: Confirm the expected functional areas, use cases, and high-level goals that the solution should address.
- Define performance criteria: Establish clear metrics for model accuracy, response times, and threshold tolerances based on your objectives.
- Examine data sources: Locate relevant datasets, noting any ownership issues, format inconsistencies, or potential gaps in coverage.
- Check data quality: Evaluate how well the datasets represent real conditions, and remove outliers or corrupted entries that could skew results.
- Assess privacy and compliance requirements: Confirm whether the data meets regulatory guidelines around consent, encryption standards, or access rights.
- Review algorithm suitability: Match the chosen models to your intended outcomes, and consider simpler approaches if they deliver better speed to market.
- Confirm resource scalability: Estimate compute needs, storage requirements, and associated costs to ensure cost-effectiveness as the solution grows.
- Document security measures: Outline authentication, data encryption, and system monitoring strategies that protect both the organization and its customers.
- Create a pilot testing plan: Start small with a controlled user group or dataset, gathering feedback before rolling out to broader populations.
- Evaluate integration complexity: Review existing infrastructure components, data pipelines, and governance protocols to pinpoint areas that need adjustments.
- Validate cost-benefit analysis: Compare the short-term investment against projected ROI and intangible benefits like improved stakeholder alignment.
- Plan a feedback loop: Establish processes that capture user comments, performance data, and error logs for continuous improvement across future versions.
A structured list ensures that each key milestone is tracked, making your AI due diligence checklist simpler to follow and adapt as your scope evolves. That level of organization leads to better communication between technical teams, management, and other stakeholders. The entire process also sets a stronger foundation for scaling machine learning, resulting in higher returns and a smoother path to market. This approach builds confidence in the final product, demonstrating that every factor—technical, financial, or compliance-related—has been properly considered before wide-scale adoption.
“A structured list ensures that each key milestone is tracked, making your AI due diligence checklist simpler to follow and adapt as your scope evolves.”
Best practices for AI due diligence
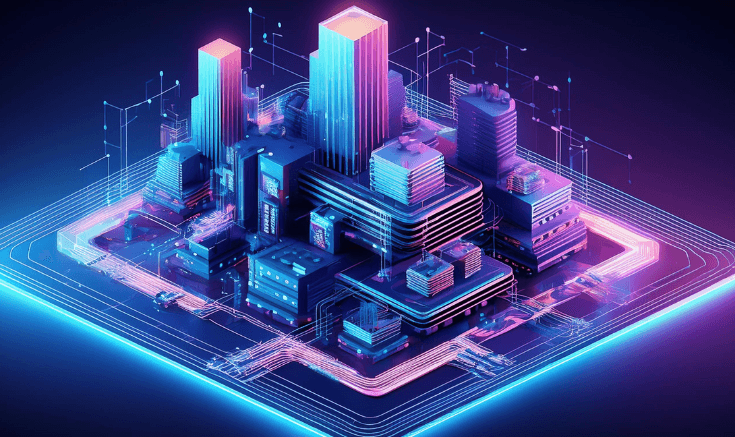
An organized set of best practices promotes consistent outcomes while building trust among your teams and investors. It brings clarity to governance structures, cost efficiencies, and project feasibility. A balanced approach ensures that machine learning solutions are tested, validated, and ready for production without hidden risks. Each action in this section supports better alignment with strategic objectives, controlling for scalability concerns and enhancing your AI due diligence checklist from concept to launch.
- Emphasize stakeholder involvement: Early engagement with leadership, compliance experts, and end users guarantees clear expectations and fewer conflicts during implementation. It also helps identify where extra resources or guidance might be needed. This open conversation paves the way for confident decision-making and fosters a shared sense of responsibility. It encourages stronger collaboration across different teams.
- Maintain thorough documentation: Written records of data sources, performance targets, and model assumptions streamline updates or audits down the line. They also equip your organization with a quick reference tool for troubleshooting and user training. Strong documentation helps preserve institutional knowledge and speeds up future expansions or pivots. It also serves as a living reference point that can be updated if any changes arise.
- Set regular review cycles: Scheduled checkpoints detect changes in data quality, performance degradation, or emerging compliance obligations before they escalate. These systematic evaluations confirm that current outputs match business goals and user needs. They also help refine resource allocation and foster agile responses to any issues. This approach strengthens accountability across various teams.
- Combine automated and manual testing: Automation saves time and flags errors rapidly, but human oversight still provides context-sensitive insights. That blend improves your ability to capture subtle anomalies that automated systems might miss. A multi-layered testing framework amplifies model performance and reduces risk across diverse deployment scenarios. It also reveals areas where manual intervention adds maximum value.
- Encourage transparency in model outputs: Explaining how your machine learning systems make recommendations builds stakeholder trust and can highlight areas for improvement. This approach facilitates constructive feedback and clarifies the business logic behind complex processes. High visibility supports ethical data usage and avoids misunderstandings over model decisions. It further streamlines the acceptance of AI-driven insights across teams.
- Conduct periodic security audits: These checks go beyond encryption and access controls, probing for potential vulnerabilities or outdated policies. Frequent security assessments keep you aligned with regulations and maintain user confidence. A firm handle on security also prevents cost overruns related to emergency fixes. It protects your valuable assets, including sensitive data and intellectual property.
- Leverage pilot programs: Testing new AI capabilities in smaller, controlled settings helps you observe practical value without risking a broader rollout. It offers early insights into cost-effectiveness, user acceptance, and data accuracy under real conditions. Timely feedback from pilot groups leads to targeted improvements that strengthen your final deployment. This strategy can highlight gaps before resources are committed on a large scale.
Embedding these steps into your AI due diligence checklist provides a strategic way to measure and refine AI initiatives. It keeps you focused on maximizing returns, expediting releases, and managing stakeholder expectations more effectively. Familiar best practices guide your teams in addressing immediate hurdles and clarifying any gray areas in model governance. A thoughtful approach ensures your AI deployments stand on stable ground, minimizing disruptions while preserving long-term adaptability.
AI is not just a new approach—it’s a gateway to more efficient, data-centered business strategies. Organizations unlock deeper insights that let them focus on actionable priorities. At Lumenalta, we specialize in designing tailored AI deployments that align with your goals, ensuring you’re ready to lead. We chart a more productive path forward by uniting technology with sustainable outcomes.
Common questions about AI due diligence
What is an AI due diligence checklist and why does it matter?
How does an AI due diligence checklist optimize time to market?
Which teams should participate in the AI due diligence checklist process?
How often should I revisit my AI due diligence checklist?
What if I already have AI systems in place—does the checklist still apply?
Want to learn how AI solutions can bring more transparency and trust to your operations?