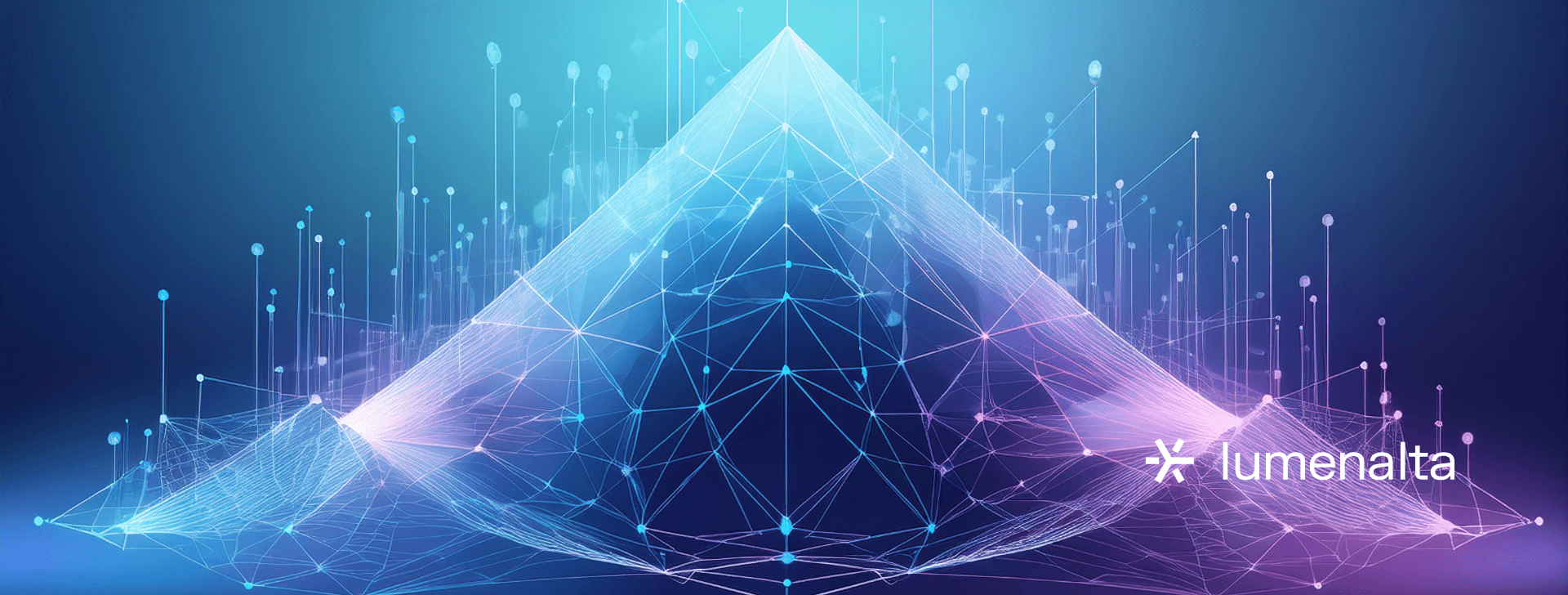
5 key big data analytics use cases in telecom
APR. 23, 2025
8 Min Read
Maximizing telecom efficiency takes more than routine upgrades or guesswork.
Providers tap into massive volumes of call records, billing data, and device interactions to enhance service quality and uncover new revenue paths. Big data analytics use cases in telecom deliver immediate operational improvements and a foundation for future expansions. Many organizations see measurable boosts in subscriber retention and cost savings when they embrace analytics across their entire ecosystem.
key-takeaways
- 1. Predictive analytics reduces churn by flagging dissatisfied customers early.
- 2. Fraud detection systems powered by machine learning minimize financial losses.
- 3. Targeted marketing campaigns boost engagement and drive immediate revenue.
- 4. Scalable data architectures allow quick responses to shifting traffic demands.
- 5. Aligned stakeholders and continuous training support lasting benefits from analytics investments.
5 key big data analytics use cases in telecom
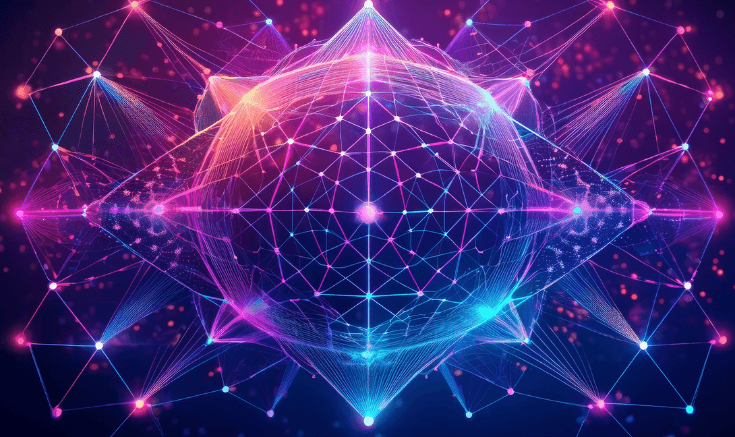
Telecom operators handle massive datasets every second as they manage voice calls, data traffic, and messaging services across countless devices. Big data analytics use cases in telecom include sophisticated strategies that merge technology and data science to elevate operations. Effective data-backed approaches enhance customer experiences, optimize costs, and reveal new revenue streams. Adopting advanced analytics can also reduce risks and protect infrastructure from malicious attacks. Industry players look for solutions that streamline processes while minimizing service disruptions. The most effective telecom data analytics use cases revolve around optimizing network performance, predicting customer needs, and refining pricing structures. Real-time insights from network equipment and user behavior shape important decisions around resource allocation.
1. Enhancing network optimization
Boosting the reliability of telecom infrastructures is an important target for most service providers. Large datasets from sensors, server logs, and connected devices can be analyzed to identify network bottlenecks or areas with excessive latency. Machine learning models help predict peak traffic intervals, allowing operators to allocate bandwidth more effectively. Proactive planning reduces downtime and ensures end users have consistent service across various coverage regions.
Predictive analytics also provides tangible gains by decreasing resource misallocation, which can save millions in operating costs. Many teams notice better customer satisfaction scores when services experience fewer outages or performance hiccups. Enhanced network optimization not only supports immediate user needs but also prepares companies for expansions into emerging service territories. A well-tuned infrastructure leads to stronger market positioning and contributes to higher investor confidence.
2. Predicting and reducing customer churn
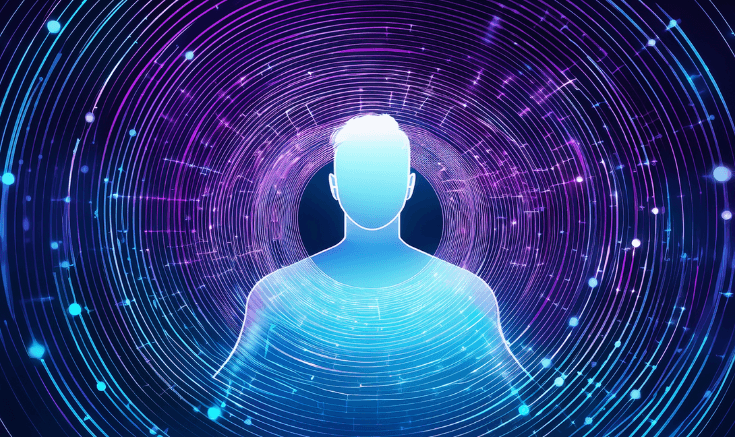
Retaining subscribers is a top priority that directly influences revenue and brand perception. Telecom operators sift through call records, billing histories, and support interactions to pinpoint recurring patterns that signal customer dissatisfaction. High churn rates often stem from coverage issues, slow data speeds, or billing complexities that push individuals to explore alternatives. Predictive analytics platforms score customers based on their likelihood to leave, prompting timely retention tactics.
Churn management workflows often include customized offers or improved plan structures that speak directly to user pain points. Some companies integrate real-time alerts when churn probability spikes, allowing immediate outreach with targeted solutions. Lower churn translates to stable revenue streams and long-term contract renewals, helping managers justify further investment in analytics capabilities. This approach cultivates ongoing loyalty and positions telecom brands as reliable partners for personal and business communication needs.
3. Detecting and preventing fraud
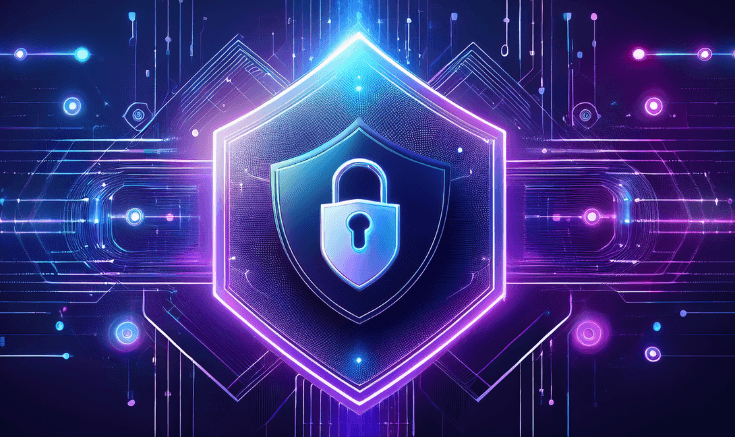
Fraudulent activities, including identity theft or billing scams, cost telecom operators significant resources every year. Detecting patterns in call durations, location changes, and account anomalies helps analysts spot suspicious behavior before it escalates. Big data analytics use cases in telecom rely on advanced algorithms that examine thousands of transactions per second, flagging anything unusual for further review. Immediate intervention and automated alerts minimize financial losses and protect users from unauthorized activities.
Implementing data-powered fraud detection safeguards brand reputation by maintaining a secure environment for both commercial and residential users. Machine learning refines these measures by adapting to new scam methods, eliminating outdated manual checks. Fraud prevention initiatives contribute to cost-effectiveness, preserve subscriber trust, and reduce the workload of legal teams. Strong security frameworks build credibility among investors and stakeholders seeking stable growth opportunities.
4. Optimizing pricing strategies
Telecom services range from pay-per-use plans to multi-device family packages, which makes pricing a delicate balancing act. Customer segments differ in their consumption patterns, whether it is heavy data usage or international roaming. Analytics solutions parse this variability by collecting extensive usage metrics and demographic information, highlighting where adjustments can boost profitability. Data visualization aids decision makers in testing different price points, promotional bundles, or service tiers without guesswork.
New plans can be rolled out faster using iterative modeling, which shortens time to market for promotional campaigns. Executives often see higher conversions and lower cancellation rates once packages align more closely with customer demand. A flexible approach to pricing benefits from continuous feedback loops that guide future updates, preventing stagnation in a dynamic sector. This proactive stance reinforces brand loyalty and fosters long-term revenue stability.
5. Personalizing marketing campaigns
Promotional outreach that speaks directly to individual preferences significantly raises engagement rates. Data points such as browsing activity, app usage, and purchase history form a holistic view of each subscriber, guiding highly relevant messaging. Marketing teams rely on big data analytics for telecom to segment audiences based on factors like age, location, or data consumption patterns. Tailored recommendations encourage users to upgrade plans, enroll in special offerings, or explore premium services.
Personalization strategies strengthen brand loyalty and reduce the likelihood of mass opt-outs, since customers see promotions that match their actual needs. Marketing departments quickly adapt their tactics based on performance metrics, improving return on ad spend. Leadership teams can trust that budgets are allocated wisely, increasing overall cost-effectiveness while achieving measurable results. Personalized experiences not only drive immediate revenue but also promote a culture of ongoing innovation in telecom data analytics use cases.
“Telecom operators handle massive datasets every second as they manage voice calls, data traffic, and messaging services across countless devices.”
Benefits of implementing big data analytics in telecom
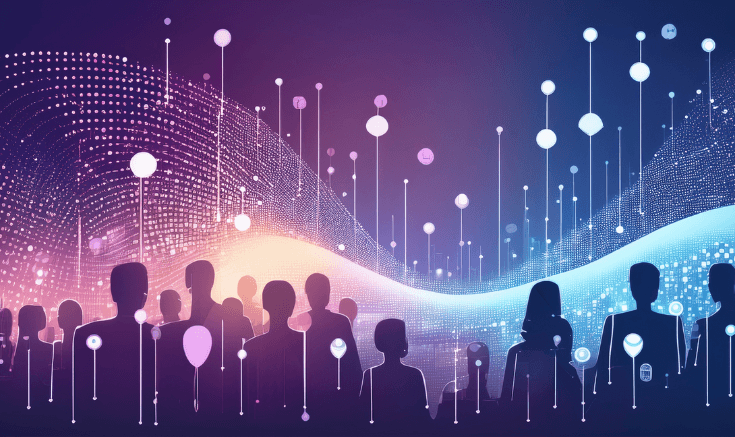
Telecom organizations find real-time analytics invaluable for maintaining quality service, protecting revenue, and aligning future investments. Many teams utilize data insights to guide technical upgrades or refine customer support protocols. Gains in operational efficiency often translate to more rapid expansions into new markets or service offerings.
- Immediate feedback on network performance, allowing timely improvements and seamless subscriber experiences.
- Enhanced fraud detection capabilities through continuous pattern analysis and machine learning models.
- Lower churn by identifying customer dissatisfaction triggers and addressing them before they escalate.
- More agile pricing approaches based on data-based insights into usage trends and consumer preferences.
- Reliable forecasting tools that enable improved resource allocation across diverse geographical regions.
- Personalized engagement tactics that resonate with individuals, strengthening brand perception and fueling growth.
These advantages reflect how thorough data analysis can elevate performance and nurture subscriber loyalty. Leaders who invest in advanced analytics often see significant returns that extend beyond short-term gains. Results become more predictable, facilitating better capital planning and resource management. Teams that adopt analytics-led workflows frequently find themselves positioned for sustainable success in an evolving telecom sector.
“Telecom organizations find real-time analytics invaluable for maintaining quality service, protecting revenue, and aligning future investments.”
Strategies for successfully adopting big data analytics in telecom
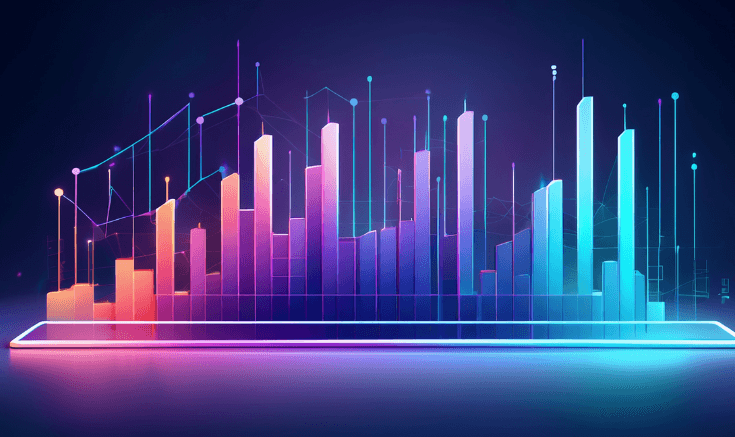
Secure stakeholder alignment
Leaders often begin with transparent communication about goals, budget requirements, and success metrics to gain broad backing. Collaborative workshops can clarify how analytics initiatives support cost efficiency, subscriber retention, and better regulatory compliance. Stakeholders from operations, finance, and IT teams should have a clear role in data governance policies, ensuring everyone is accountable for outcomes. This inclusive approach fosters a sense of shared responsibility, minimizing disruptions when new processes or tools are introduced.
Focus on scalable architecture
Infrastructure that grows with organizational demands is essential for real-time data ingestion and processing. Cloud-based solutions and container orchestration platforms help manage surges in data volume while keeping overhead manageable. Leaders allocate resources in a way that matches current requirements without committing to unnecessary capital expenses. Scaling smartly also sets the stage for expansions into new services, such as 5G or emerging IoT applications.
Implement targeted training
Technical proficiency among employees leads to efficient workflows and faster adoption cycles. Training programs should address both the fundamentals of analytics and the day-to-day usage of relevant tools. Guidance from data scientists and senior engineers helps operational teams incorporate insights into standard procedures, promoting a data-first mindset. A well-trained workforce drives user satisfaction and fosters consistent innovation throughout the organization.
Adopting big data analytics use cases in telecom position service providers to minimize risks while maximizing returns. Proactive planning, robust architecture, and continuous learning anchor the most successful deployments, making advanced analytics a key driver of growth. Customer-centric initiatives emerge naturally once teams embed data insights into their daily routines. Thoughtful implementation often reduces operational complexity and paves the way for sustainable gains.
Big data analytics is not just a technical hurdle—it’s an avenue for sustainable growth and strategic differentiation. The right data insights accelerate product launches, empower cost-effective scaling, and amplify subscriber trust. At Lumenalta, we specialize in designing tailored solutions that align analytics initiatives with business goals, ensuring you stay prepared for emerging opportunities. Let’s chart a brighter path together.
table-of-contents
- 5 key big data analytics use cases in telecom
- 1. Enhancing network optimization
- 2. Predicting and reducing customer churn
- 3. Detecting and preventing fraud
- 4. Optimizing pricing strategies
- 5. Personalizing marketing campaigns
- Benefits of implementing big data analytics in telecom
- Strategies for successfully adopting big data analytics in telecom
- Common questions about big data analytics use cases in telecom
Common questions about big data analytics use cases in telecom
How do telecom operators begin with big data analytics?
What are common data points used for telecom analytics?
Why is cost-effectiveness a central focus for big data analytics use cases in telecom?
How do fraud detection systems stay current with new threats?
Which adoption strategy works best for telecom data analytics use cases?
Want to learn how big data analytics can bring more transparency and trust to your operations?