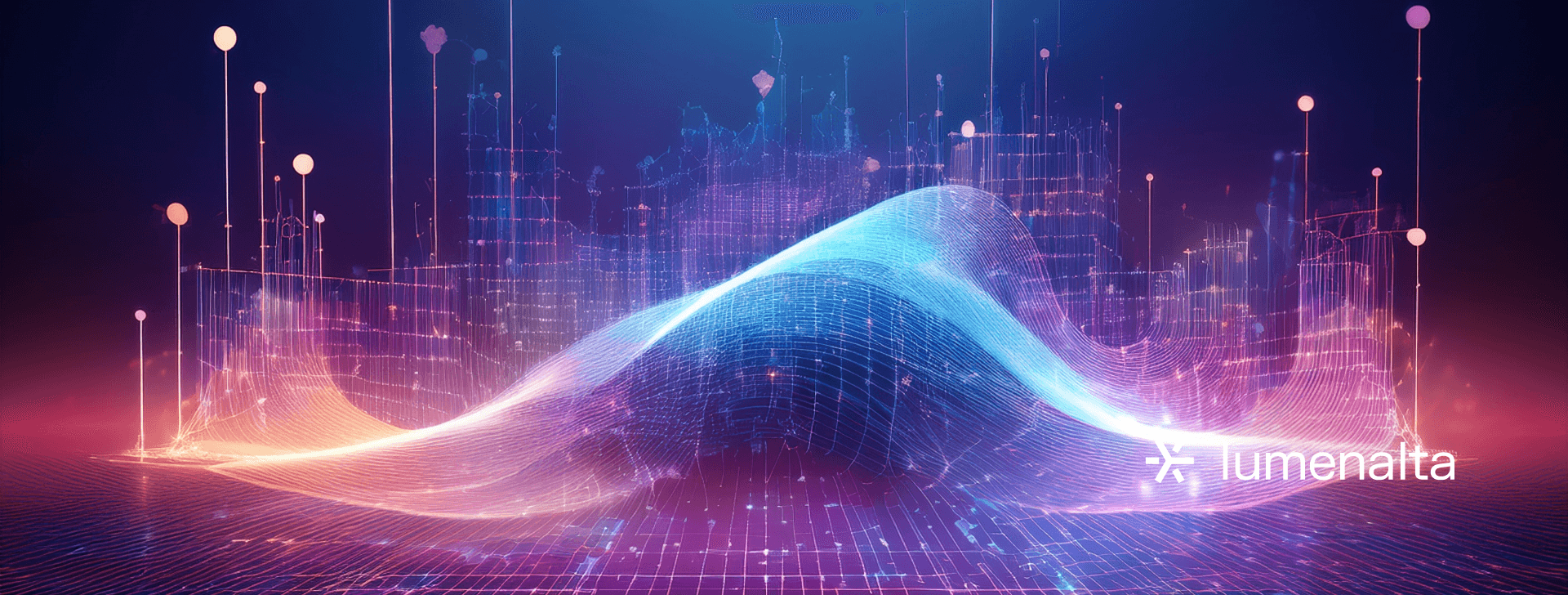
A guide to data analytics in the telecom industry
APR. 5, 2025
7 Min Read
Data analytics in the telecom industry amplifies revenue growth and fosters better user experiences for millions of subscribers.
Many service providers realize that old methods lack the precision needed to keep up with consumer needs. Shifting to robust data-based practices can pinpoint new revenue streams, reduce churn, and predict network usage with greater accuracy. This targeted approach often reveals fresh opportunities to boost ROI and satisfy shifting market trends. Machine learning systems and statistical models can sort through unstructured information to detect patterns, anomalies, and usage spikes. Telecom data analytics also drives efficiency by flagging potential issues before they escalate. Fewer disruptions mean fewer complaints and a positive reputation for the brand. Executives who embrace these insights often find better alignment among stakeholders and stronger investor confidence.
key-takeaways
- 1. Data analytics in the telecom industry helps detect revenue leakages and optimize network capacity.
- 2. Telecom data analytics supports actionable forecasts that reduce churn and enhance user satisfaction.
- 3. Diagnosing historical data reveals trends that guide future product launches.
- 4. Scalable infrastructures and robust governance can reduce technical obstacles.
- 5. Measuring ROI requires clear KPIs and consistent reporting to highlight tangible outcomes.
What is data analytics in the telecom industry?
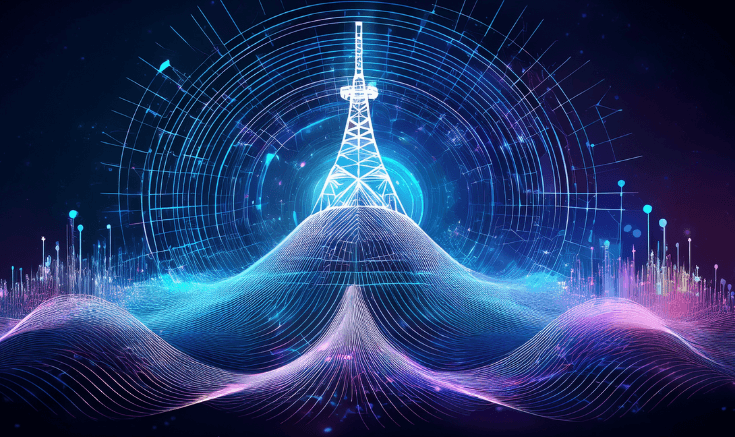
Data analytics in the telecom industry involves collecting, organizing, and interpreting large datasets from subscriber usage patterns. This approach focuses on structured and unstructured information such as call records, messaging traffic, data consumption trends, and more. Each insight helps service providers plan better packages that cater to user preferences. Many organizations value these findings because they inform important business strategies.
Traditional practices often relied on manual methods that offered partial visibility into overall performance. Shifting to automated data pipelines allows near real-time feedback and more agility in responding to market conditions. Telecom data analytics also addresses revenue leakages by spotting fraudulent activities sooner. It ensures more transparent operations, which can boost service quality and reduce operational costs.
"Shifting to robust data-based practices can pinpoint new revenue streams, reduce churn, and predict network usage with greater accuracy."
Benefits of implementing data analytics in the telecom industry
Many professionals seek better ways to improve service delivery and profitability. Data analytics in telecom introduces a data-backed mindset that addresses these objectives. Clear insights guide the launch of new offerings, ensuring a strategic allocation of resources. This process brings relevant intelligence that supports executive teams looking for cost-effective performance enhancements.
- Enhanced network efficiency: Data points from different nodes help determine peak usage times, guiding resource allocation to prevent bandwidth bottlenecks.
- Stronger customer retention: Telecom customer data analytics pinpoints the exact features and packages that increase loyalty and reduce churn rates.
- Fraud reduction: Identifying abnormal calling or messaging patterns in near real-time can limit fraudulent transactions and save revenue.
- Personalized marketing: Recommendations based on specific usage behaviors help match customers with plans that suit their lifestyles.
- Faster innovation cycles: Testing new services with targeted analytics reduces guesswork and focuses on tangible results that drive measurable gains.
Leaders often find that these benefits build a foundation for more effective communication across departments. Analysts and operations teams share insights that guide product development. Faster concept-to-market cycles emerge when everyone relies on consistent, data-backed metrics. These improvements pave the way for more advanced strategies that utilize analytics for broader objectives.
Big data analytics vs data analytics in the telecom industry
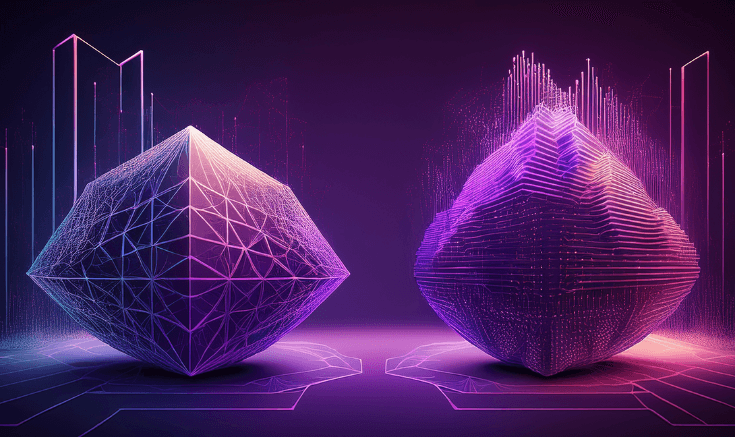
The main difference between big data analytics and data analytics in the telecom industry is the scale of information processed. Big data analytics addresses extremely large and complex datasets that originate from massive subscriber bases and interconnected systems. Data analytics focuses on extracting practical insights from these large volumes but may not always require specialized infrastructure for extremely high-velocity data streams. Both methodologies aim to enhance efficiency, revenue, and customer satisfaction.
Big data analytics often involves distributed computing platforms and specialized tools that handle parallel processing. Data analytics in telecom usually relies on more conventional methods to reveal patterns in usage statistics or marketing outcomes. Companies that handle smaller subscriber bases may not need advanced platforms but still value thorough analysis of call detail records or service usage metrics. Each approach focuses on elevating performance metrics and delivering better subscriber experiences.
Types of data analytics in telecom
There are multiple approaches that reveal different aspects of subscriber behavior and network health. Each method concentrates on specific objectives, such as identifying how past activities predict future patterns. Some focus on historical data to assess trends, while others look ahead with predictive models. It is often important to select the right mix of analytics types to guide tactical and strategic decisions.
- Descriptive analytics: Summarizes historical usage, churn, and revenue trends to provide a clear snapshot of past events.
- Diagnostic analytics: Investigates why certain events occurred, often using root cause analysis to find correlations or anomalies.
- Predictive analytics: Uses statistical models and machine learning to anticipate future usage, service demands, and churn risks.
- Prescriptive analytics: Suggests specific steps to address predicted outcomes, offering a more proactive approach to improving metrics.
- Real-time analytics: Monitors ongoing activities, such as call volumes and data traffic, offering near real-time alerts that guide swift operational decisions.
Executives often combine these types for a comprehensive approach. Diagnostic insights can lead directly to predictive forecasting, which then shapes prescriptive actions. Many telecom data analytics strategies also incorporate real-time monitoring for immediate adjustments in traffic management or customer care. This balanced framework supports agile decision processes that align with organizational goals.
Applications of data analytics in telecom operations
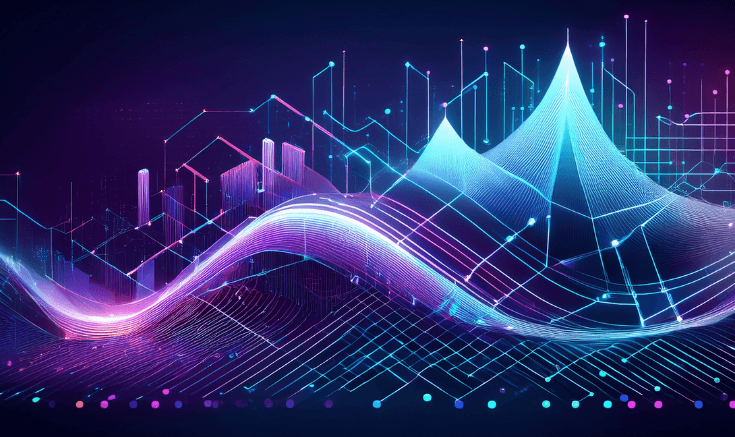
Many service providers use analytics to identify usage trends and plan network expansions. This approach can reveal previously overlooked business opportunities that improve average revenue per user. New data patterns often inspire fresh strategies for marketing, partner collaborations, or service bundling. Several core applications revolve around technical efficiency, fraud mitigation, and personalized offerings.
Network optimization
Operators need consistent visibility into traffic loads across multiple regions. Data analytics in telecom supports more precise resource distribution, leading to reduced congestion and improved speed for subscribers. Infrastructure upgrades often rely on analytics to ensure that every new project meets specific usage criteria. The result is a more resilient network that supports stable connectivity and higher customer satisfaction.
Personalizing marketing campaigns
Analyzing subscriber segments reveals which promotions have the highest impact on different user groups. These insights also show which channels drive positive responses, such as SMS blasts or social media ads. Targeted marketing reduces wasted ad spend and focuses on customers who appreciate tailored plans. Most importantly, personalization can encourage longer relationships with fewer reasons to switch carriers.
Fraud mitigation
Criminal activities such as illegal call routing can cause serious financial losses. Data analytics identifies suspicious usage patterns by tracking call durations, unusual destinations, or excessive messaging spikes. Quick detection reduces the window of opportunity for illegal operations. Organizations that monitor usage in near real-time often see a decline in fraud-related revenue leaks.
Strategies for optimizing data analytics in telecom
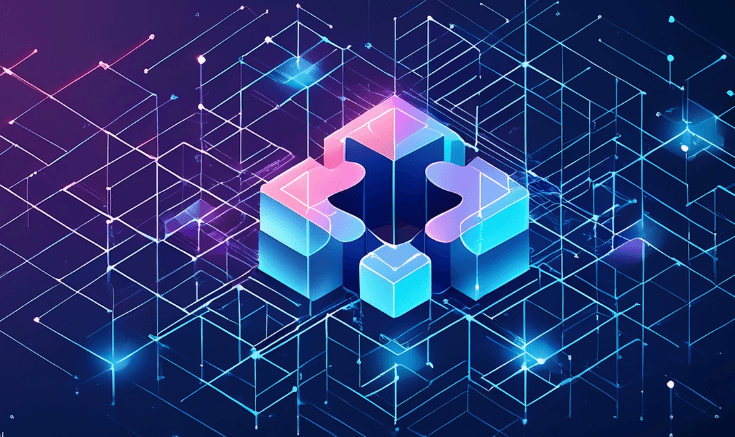
Modernizing processes involves more than adopting new tools. Thoughtful planning ensures that teams know how to integrate these technologies into their existing workflows. Many executives focus on building solid foundations that include quality data management practices and reliable governance. Sustained optimization often requires ongoing evaluations to address potential issues or scale up as subscriber bases grow.
Emphasize data governance
Clear protocols define how information is stored, protected, and accessed. This helps maintain consistency across multiple departments and fosters trust in the outcomes of analysis. A robust governance framework can also address compliance requirements associated with sensitive subscriber data. Well-established rules often speed up the process of turning raw information into meaningful insights.
Invest in scalable infrastructure
Upgrading hardware and software solutions can support larger data volumes without performance bottlenecks. Some telecoms adopt cloud-based platforms to quickly adjust storage and processing capacity based on usage spikes. This approach often lowers costs by reducing idle infrastructure. It also promotes agility when rolling out new data analytics initiatives that require additional resources.
Champion cross-functional teamwork
Coordinating efforts between data scientists, engineers, marketing teams, and executives leads to better outcomes. Everyone benefits when analytics strategies align with revenue targets and user experience goals. Collaboration also speeds decision cycles because each group contributes specialized knowledge. A unified approach helps maintain synergy as companies expand analytics capabilities.
"A robust governance framework can also address compliance requirements associated with sensitive subscriber data."
Challenges faced in telecom data analytics adoption
Many organizations encounter technical and operational obstacles during deployment. These hurdles can slow progress or result in fragmented data, limiting the scope of analysis. Some struggles arise from incompatible systems or a lack of skilled personnel to interpret findings.
- Data silos: Isolated storage methods make it difficult to combine multiple data sources into a unified view.
- Poor data quality: Inaccurate or incomplete records can distort results and lead to inefficient decisions.
- Security concerns: Handling subscriber data requires rigorous protocols to prevent unauthorized access and breaches.
- Cost pressures: Building robust analytics frameworks may involve significant investments in hardware, software, and talent.
- Limited analytics talent: Finding professionals who understand data science and telecom operations can be challenging.
Addressing these challenges involves structured planning, collaboration, and adequate resourcing. Many telecoms allocate budgets to training existing staff or hiring external expertise. Others work on technology upgrades that simplify data sharing across departments. Each improvement makes it easier to adopt analytics at scale.
Measuring the ROI of data analytics initiatives in telecom
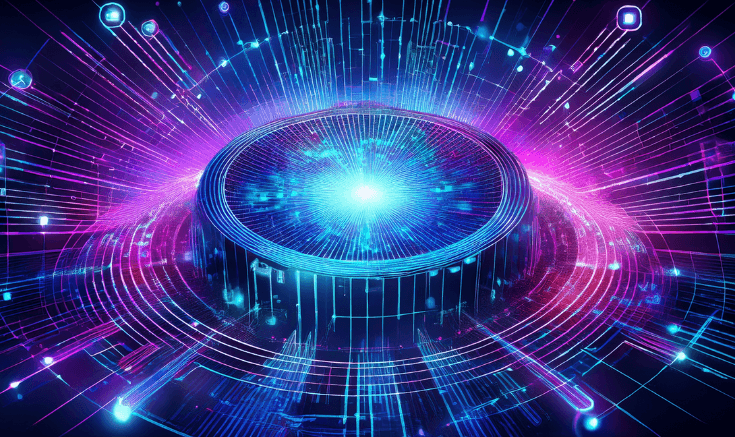
Leaders often assess financial returns by comparing initial investments with tangible results like reduced churn or higher revenue per user. The process usually starts with setting clear key performance indicators (KPIs) that track cost savings, subscriber growth, and satisfaction improvements. Detailed reporting helps highlight which projects yield the best outcomes. This level of visibility sets the stage for greater confidence in analytics-based strategies.
Additional measurements often include operational metrics such as the time it takes to roll out new services. Many executives also measure the rate of fraud incidents before and after implementing advanced analytics. A noticeable decline in fraudulent activity can translate to significant cost savings and higher trust among subscribers. Consistent success in these areas usually justifies further investments in data analytics in telecom.
Data analytics is not just a technical upgrade—it’s a direct route to meaningful insights and better outcomes. Unlocking precise intelligence across subscriber touchpoints helps organizations stay agile and focus on measurable progress. At Lumenalta, we develop customized analytics frameworks aligned with your strategic priorities, ensuring every data point leads to tangible benefits. Let’s chart a brighter path forward.
table-of-contents
- What is data analytics in the telecom industry?
- Benefits of implementing data analytics in telecom industry
- Big data analytics vs data analytics in the telecom industry
- Types of data analytics in telecom
- Applications of data analytics in telecom operations
- Strategies for optimizing data analytics in telecom
- Challenges faced in telecom data analytics adoption
- Measuring the ROI of data analytics initiatives in telecom
- Common questions about data analytics in telecom industry
Common questions about data analytics in telecom industry
How does data analytics in telecom support better product development?
What is the difference between telecom data analytics and general analytics methods?
How do providers use telecom customer data analytics to improve loyalty?
How often should telecom operators update data analytics models?
Does data analytics in telecom help reduce operating costs?
Want to learn how data analytics can bring more transparency and trust to your operations?