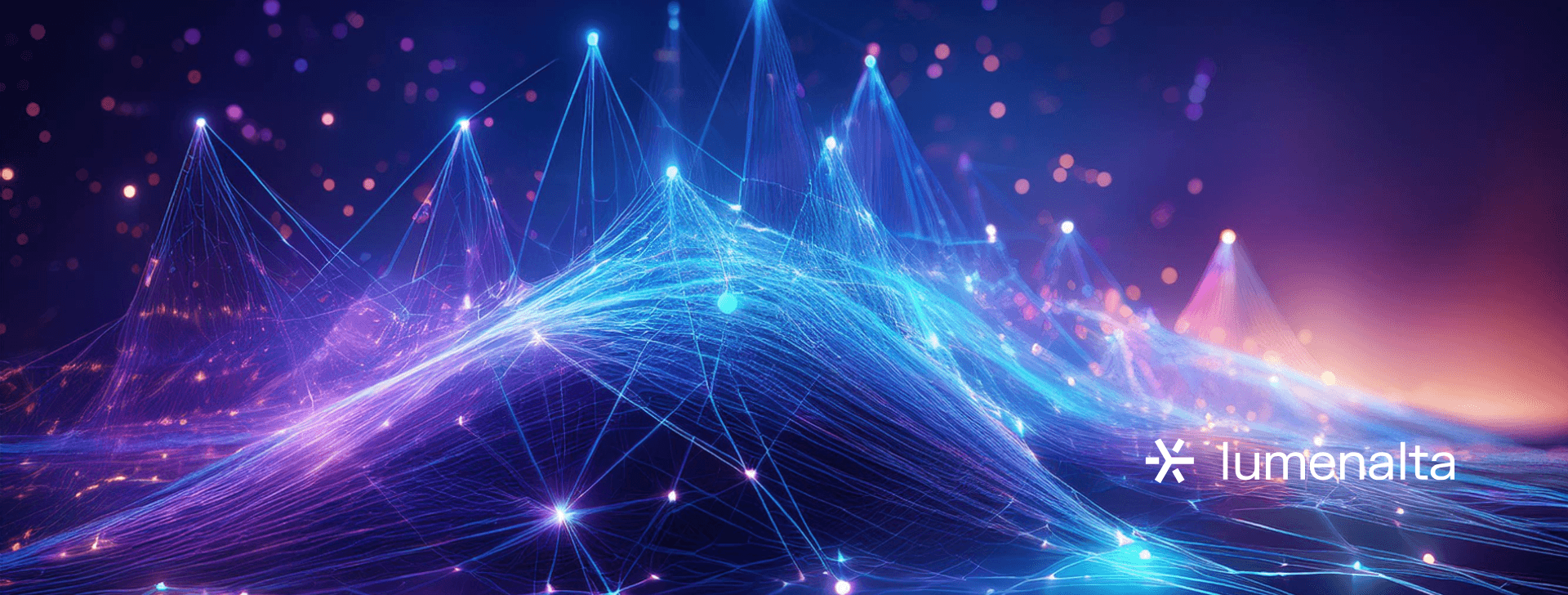
AI governance checklist (updated 2025)
MAR. 4, 2025
6 Min Read
A structured approach to AI governance can unite technology teams, executives, and subject matter experts around clearly defined performance targets.
This starts with assigning dedicated governance roles, followed by ongoing communication that flags risks and promotes collaboration. Some organizations embed these strategies into broader initiatives, such as compliance committees or performance review boards, allowing a single source of truth to handle AI policies.
Key takeaways
- 1. Oversight roles and defined processes protect AI initiatives from risk and maintain consistency.
- 2. Strong data management practices reduce waste, lower costs, and boost model accuracy.
- 3. Formal ethical and regulatory checkpoints prevent legal complications and protect brand reputation.
- 4. Transparent communication and comprehensive security measures safeguard data and drive team confidence.
- 5. Regular monitoring ensures AI solutions remain stable, relevant, and valuable.
What is AI governance?
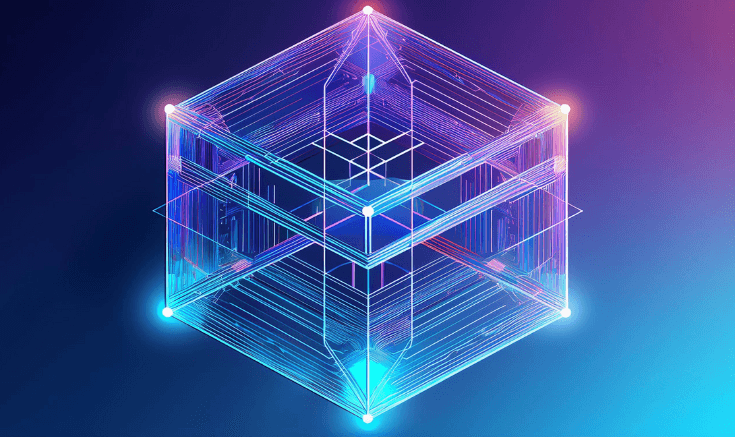
Artificial intelligence governance refers to structured policies, processes, and guidelines that oversee the entire lifecycle of machine learning models. This involves ensuring that data sources are validated, compliance requirements are upheld, and ethical responsibilities are observed. Leaders have found that consistent oversight allows for smoother integration of AI-driven capabilities, from recommendation engines in retail to operational analytics in manufacturing.
Organizations use AI governance to reduce exposure to missteps and optimize performance from the outset. Transparent standards and stakeholder collaboration help align projects with business objectives, while also protecting data. The approach focuses on balancing innovation with responsibility, especially in sectors such as healthcare and logistics, where decisions and results can shape future investments.
“Artificial intelligence governance refers to structured policies, processes, and guidelines that oversee the entire lifecycle of machine learning models.”
Importance of an AI governance checklist
A clear set of guidelines helps you confirm that every phase of an AI project meets strategic and regulatory requirements. Teams benefit from having a structured reference for ethical concerns, risk controls, and quality assurance. This promotes consistency, lowers cost overruns, and streamlines implementation across different departments.
An AI governance checklist also defines roles, responsibilities, and escalation processes. Leaders need a practical framework to identify potential problems early, enabling proactive interventions. With these steps in place, projects stand a better chance of scaling smoothly while staying focused on tangible business outcomes, such as improved efficiency or enhanced customer engagement.
AI cybersecurity & governance considerations
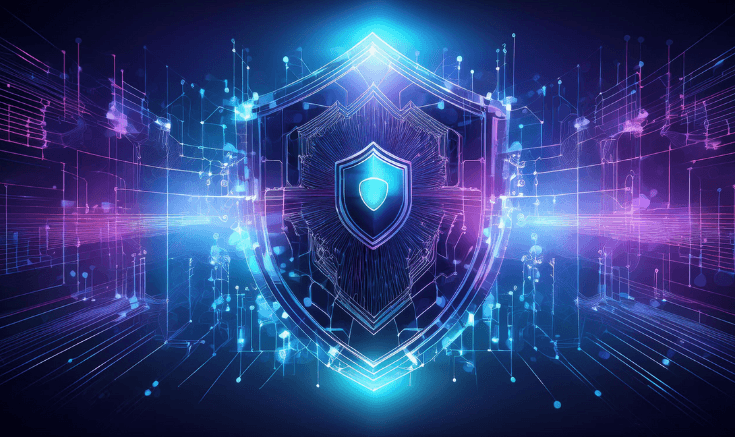
Cybersecurity is woven into every facet of AI initiatives, especially when sensitive data is processed or when automated actions have real-world consequences. Securing data pipelines and verifying access permissions protect the integrity of machine learning (ML) outputs. Financial services, industrial operations, and healthcare organizations often handle regulated information, so encrypting transmissions and monitoring for breaches are essential tactics.
Governance complements cybersecurity by establishing consistent policies for data handling and decision authority. Teams must confirm that quality thresholds are met before models are deployed. This includes verifying that large language model (LLM) tools address relevant compliance mandates. A combined AI cybersecurity & governance checklist can unify technical, ethical, and regulatory elements, ensuring that projects move forward with transparency and accountability.
AI governance checklist
This section provides a practical framework for building trust, optimizing expenditures, and confirming alignment with business objectives. Many teams have discovered that a structured list mitigates common pitfalls, accelerates time-to-market, and creates a foundation for continuous improvement. Leaders from finance to logistics can reference each item as they design or refine their AI strategy, ensuring an intentional approach to oversight. The goal is to integrate guardrails that prevent miscalculations or oversights, while also seizing the greatest possible return on investment. All items are interconnected and support an efficient, consistent governance model. An organization that follows these steps is more likely to spot untapped opportunities, protect data, and maintain stakeholder confidence.
Below is a seven-point checklist that highlights important considerations for adopting a sound governance structure. Each point begins with a directive and concludes with a measurable outcome. Decision-makers can adapt these items to address industry-specific regulations, investor expectations, or organizational objectives. After every point, there is a short, direct-action checkbox list you can follow to remain on track.
1. Establish clear accountability
Assign ownership of AI initiatives to specific teams or individuals, confirming that responsibilities are understood at every level. Leaders sometimes rely on cross-functional committees to handle oversight, while smaller enterprises might appoint a single manager with the requisite expertise. The important step is to formalize who approves data sources, who reviews model outputs, and who addresses exceptions when they arise.
Centralizing accountability reduces confusion and shortens the feedback loop. It becomes easier to keep a project on track and on budget because the decision structure is defined in advance. A measurable benefit is faster alignment across stakeholders, resulting in fewer administrative delays and more predictable outcomes.
Action checklist: Use these quick checkboxes to verify you have assigned accountability properly:
- Identify one primary stakeholder who oversees each AI project.
- Define approval steps for data, model updates, and final deployment.
- Create an escalation plan for errors or performance shortfalls.
Building out accountability ensures that AI projects remain aligned with strategic targets. With these steps in place, you avoid ambiguous decision structures, save time, and reinforce organizational consistency.
2. Define data collection and quality standards
Document requirements for data accuracy, format, and completeness before training any model. This could include specifying relevant time periods, removing duplicates, and auditing sources for potential biases. Standardizing data inputs decreases the likelihood of skewed or erroneous results, which can undermine confidence in the entire project.
Teams that maintain strong data governance spend less time troubleshooting and more time producing value from analytics. Projects with reliable datasets often yield more accurate forecasts, leading to better resource allocation and improved bottom-line impact. This clarity around data also helps everyone understand what is possible within an AI system’s scope.
Action checklist: Evaluate and maintain data quality with these tangible checkpoints:
- Compile a data inventory, including sources, owners, and formats.
- Create a protocol for identifying and removing duplicates or invalid entries.
- Perform periodic audits to check bias and completeness in your datasets.
Clear data standards simplify the entire machine-learning lifecycle. Teams benefit from fewer revisions, higher confidence in insights, and more consistent outcomes across various use cases.
3. Implement ethical and regulatory checkpoints
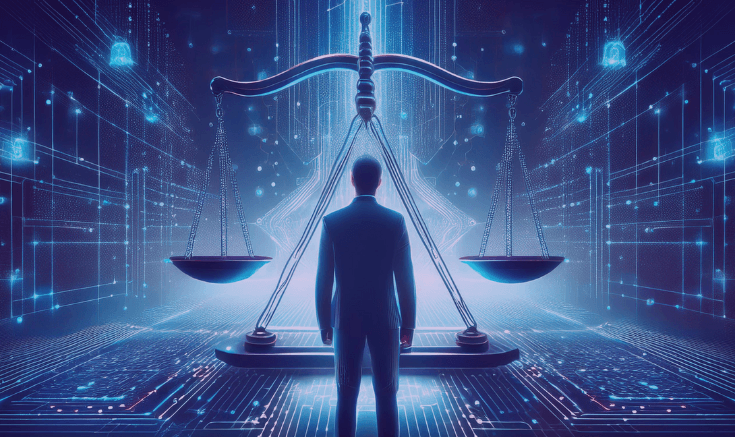
Embed ethics and compliance reviews into each phase of development, ensuring that your AI solution aligns with legal guidelines. This might involve verifying adherence to federal or industry-specific regulations, establishing transparency for end users, or addressing fairness concerns that arise from algorithmic decisions. Some industries require formal audits, while others depend on voluntary standards.
A consistent ethical review framework fosters user trust and protects your organization from fines or reputational harm. These checkpoints confirm that the AI solution is poised to scale responsibly, adding value without introducing unwarranted risks. The measurable outcome is an infrastructure that meets both internal policies and external expectations.
Action checklist: Ensure your AI solution remains within legal and ethical boundaries:
- Develop a documented process for ethical impact reviews at each milestone.
- Cross-reference current regulations to confirm your project aligns with sector-specific guidelines.
- Conduct user feedback sessions to test whether the AI’s outputs maintain fairness and clarity.
Following these steps decreases the probability of significant compliance issues. You also reinforce trust with customers, partners, and regulatory bodies, preserving both reputation and revenue.
4. Integrate security measures throughout the pipeline
Apply robust safeguards to data ingestion, processing, and output generation stages. Responsibilities include monitoring for unauthorized access, encrypting data transmissions, and verifying that only approved models and components make it into production. Implementing a security-first mentality protects not only your customers but also your intellectual property and operational continuity.
Reinforcing security from design to deployment minimizes the likelihood of damaging breaches or compromised insights. Leaders can quantify these benefits by comparing risk levels before and after implementing safeguards. The outcome is not just compliance; it also builds a competitive edge when clients know their data is treated with care.
Action checklist: Strengthen your AI system’s security posture by checking off the following:
- Implement role-based access control for all data handling and model updates.
- Use encryption for data in transit and at rest to protect sensitive information.
- Configure real-time monitoring and alerts for unauthorized access or unusual activity.
These measures limit vulnerabilities and reinforce trust among users and stakeholders. When security is integrated from the start, AI projects become more resilient and cost-effective over their lifecycle.
5. Evaluate model performance with real metrics
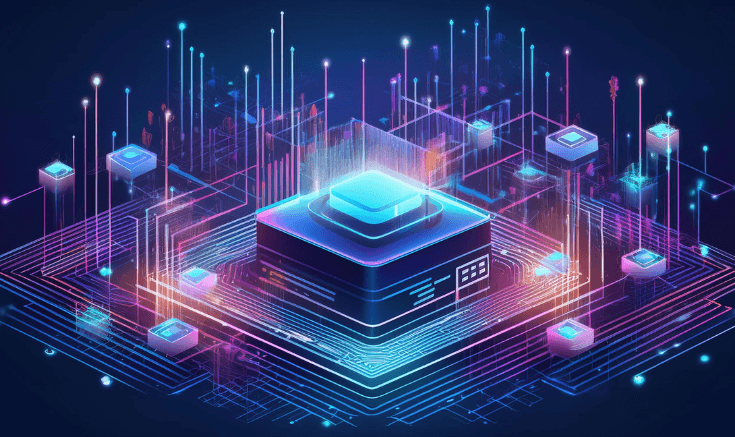
Identify relevant performance indicators, such as accuracy or recall, to measure how well a model meets its intended function. Conduct periodic evaluations to detect drift, which can happen when market conditions, user behavior, or data availability shift. Decision-makers should set a schedule for re-training or recalibrating models based on results.
Actionable metrics provide clarity about whether a machine learning initiative is delivering a meaningful return on investment. Using defined benchmarks allows teams to spot patterns and pivot promptly if performance declines. Improved insights often translate into cost savings or new revenue streams, ultimately reinforcing the rationale for AI adoption.
Action checklist: Keep your AI models on track and adaptable:
- Define performance benchmarks, such as accuracy thresholds or precision targets, for your specific use case.
- Schedule regular performance reviews to detect issues before they become critical.
- Create a re-training protocol that identifies when and how to refresh model parameters.
Regular reviews prevent performance decay and validate the ongoing value of AI solutions. Setting deliberate metrics also helps justify AI-related expenditures and fosters a culture of continuous optimization.
6. Establish a transparent communication plan
Define how insights, updates, and concerns are shared within the organization. Teams can outline key communication channels, specify who is notified of major results, and document how user feedback is incorporated. This approach aligns developers, data scientists, and business units under a single vision, clarifying the overall direction and progress of AI solutions.
Structured communication helps pinpoint obstacles early and encourages collective ownership of AI initiatives. Transparency often increases confidence among senior leaders and end users, because they can see how decisions are made and how issues are resolved. A tangible benefit is smoother collaboration, leading to faster concept-to-deployment cycles and better outcomes for clients or customers.
Action checklist: Ensure an open flow of information within your AI projects:
- Develop a clear reporting schedule for sharing updates with stakeholders.
- Assign a specific channel for urgent alerts, such as model anomalies or security breaches.
- Publish post-implementation feedback for teams to learn from successes and challenges.
Well-coordinated communication limits surprises, simplifies problem resolution, and boosts morale. This openness to feedback encourages shared responsibility, making it easier for everyone to stay informed and engaged.
7. Plan for ongoing monitoring and improvements
Schedule regular reviews to confirm models remain relevant and accurate. Outline how updates will be tested and introduced, ensuring that changes do not disrupt production workflows. This might include scenario planning for unexpected data shifts, new regulatory mandates, or expansions into additional markets.
A structured plan for continuous oversight secures long-term value and stability for AI solutions. Model decay or sudden changes in data can significantly reduce effectiveness if left unchecked. Persistent monitoring extends the product lifecycle, lowers maintenance costs, and preserves the trust of investors, customers, and internal stakeholders.
Action checklist: Keep AI solutions robust and ready for new challenges:
- Implement a version control system for managing and rolling back model updates.
- Conduct stress tests or pilot runs before deploying updated models to production.
- Plan recurring roadmap reviews to align AI projects with evolving organizational objectives.
Proactive monitoring ensures that AI models stay current and relevant. This forward-thinking approach reduces downtime, prevents cost overruns, and supports stable, sustainable returns on AI investments.
Crafting a strong AI governance checklist with the points above often highlights additional gaps or new possibilities. Leaders can then incorporate those insights, whether they revolve around advanced cybersecurity practices or new processes for cross-department collaboration. The key is to keep refining and iterating, ensuring that governance protocols stay relevant to the organization’s goals and external market conditions. Once this checklist is integrated into standard operating procedures, it becomes second nature for teams to follow consistent, responsible pathways. Progress is easier to measure and justify, which builds momentum for further adoption and alignment.
“Reinforcing security from design to deployment minimizes the likelihood of damaging breaches or compromised insights.”
Implementing AI governance strategies
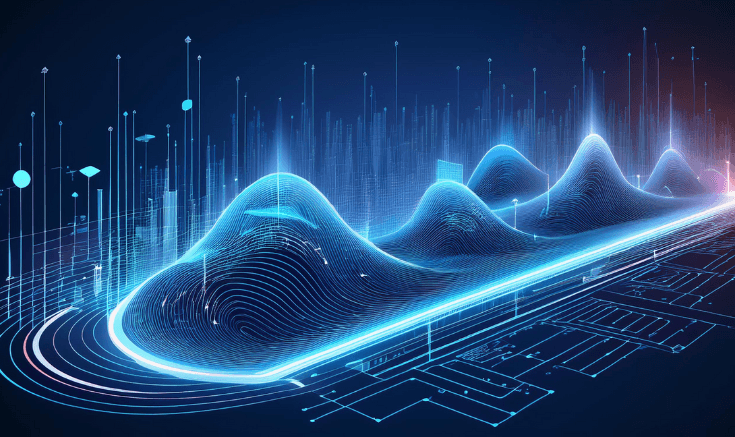
A structured approach to governance can unite technology teams, executives, and subject matter experts around clearly defined performance targets. This starts with assigning dedicated governance roles, followed by ongoing communication that flags risks and promotes collaboration. Some organizations embed these strategies into broader initiatives, such as compliance committees or performance review boards, allowing a single source of truth to handle AI policies.
A thorough plan includes training programs for data scientists and product managers, as well as refresher sessions for leadership. Consistency is easier to achieve when team members follow a shared framework, validated by external audits or industry best practices. This reduces the likelihood of project failure, fosters a sustainable innovation culture, and positions AI as an integral part of achieving business goals.
AI governance is not just a regulatory checkbox—it’s a path to more responsible, transparent, and scalable data-driven operations. It helps organizations allocate resources effectively, protect stakeholder trust, and prioritize impactful outcomes. At Lumenalta, we specialize in designing tailored governance frameworks that align with your business goals, ensuring you’re equipped to lead. Let’s chart a brighter path together.
Table of contents
- What is AI governance?
- Importance of an AI governance checklist
- AI cybersecurity & governance considerations
- AI governance checklist
- 1. Establish clear accountability
- 2. Define data collection and quality standards
- 3. Implement ethical and regulatory checkpoints
- 4. Integrate security measures throughout the pipeline
- 5. Evaluate model performance with real metrics
- 6. Establish a transparent communication plan
- 7. Plan for ongoing monitoring and improvements
- Implementing AI governance strategies
- Common questions about AI governance
Common questions about AI governance
How can an AI governance checklist improve collaboration among departments?
What makes AI cybersecurity & governance checklist efforts unique for regulated industries?
Do smaller businesses need large language model capabilities if they adopt governance protocols?
Which metrics indicate a healthy return on investment for AI governance efforts?
Can an AI governance framework adapt to changing strategies and market shifts?
Want to learn how AI can bring more transparency and trust to your operations?