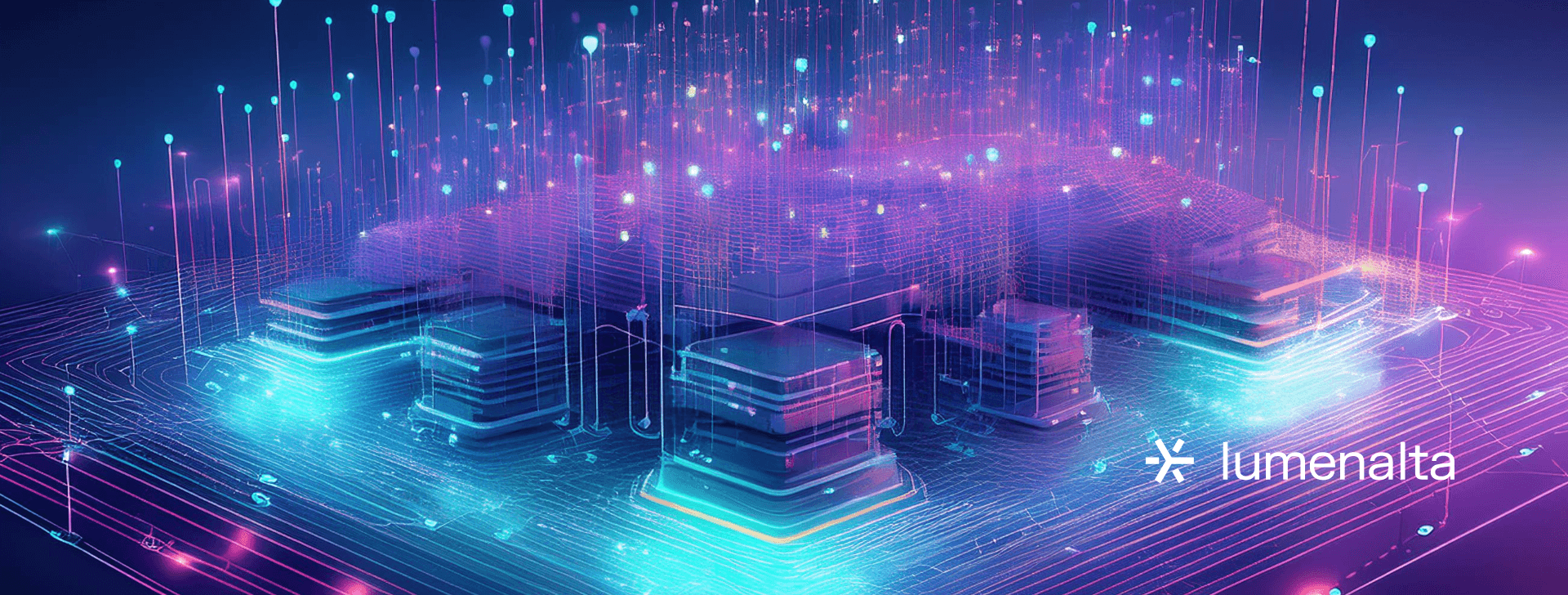
13 data lake advantages and disadvantages
FEB. 14, 2025
7 Min Read
Businesses generate massive volumes of structured and unstructured data from various sources, including customer interactions, IoT devices, transaction records, and multimedia content.
Traditional storage systems require predefined schemas that limit flexibility, making it difficult to accommodate new data formats or scale operations efficiently. A data lake eliminates these constraints by allowing raw data storage without immediate formatting, preserving its original structure for future analysis.
This approach improves operational efficiency and supports advanced analytics, artificial intelligence, and machine learning applications. Organizations gain the ability to analyze information in its native form, extracting insights that improve business outcomes. While data lakes offer significant benefits, they also require governance measures to prevent disorganization, security risks, and unnecessary storage costs.
Key takeaways
- 1. A data lake provides a flexible and scalable storage solution that retains raw data in its native format, supporting structured, semi-structured, and unstructured data.
- 2. Unlike a data warehouse, a data lake does not require predefined schemas, allowing businesses to process data for multiple analytics use cases, including machine learning and artificial intelligence.
- 3. Proper governance is essential to prevent disorganization, security risks, and rising storage costs, as poorly managed data lakes can lead to inefficiencies.
- 4. Various industries use data lakes to enhance fraud detection, customer analytics, predictive maintenance, and operational efficiency.
- 5. A well-managed data lake improves data accessibility across teams, eliminating silos and allowing centralized access to business intelligence tools.
What is a data lake?
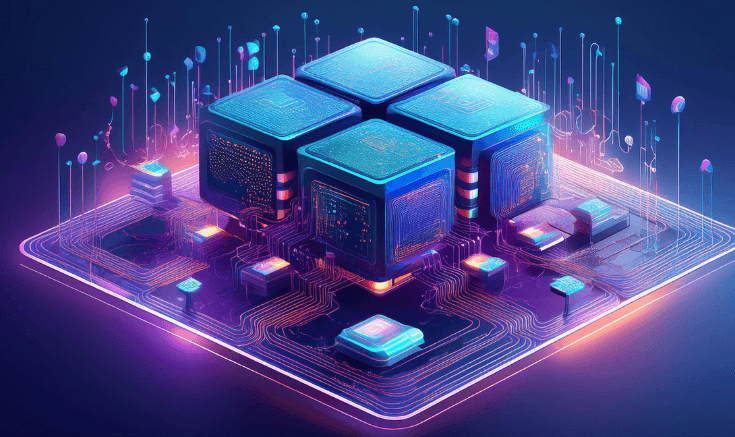
A data lake is a centralized repository designed to store raw data from multiple sources in a flexible and scalable format. Unlike traditional databases that require immediate structuring, a data lake allows businesses to collect, retain, and process information without predefined schemas. This flexibility makes it easier to support changing analytics needs and accommodate large volumes of structured and unstructured data.
Structured data includes information from relational databases, spreadsheets, and transactional systems, while unstructured data consists of emails, videos, images, and social media interactions. A data lake can also store semi-structured formats such as JSON and XML, making it possible to run complex queries across multiple data types.
Without proper organization, data lakes can become difficult to manage, leading to inefficiencies in retrieval and analysis. Security controls, indexing strategies, and governance frameworks help maintain accessibility and reliability. When implemented correctly, a data lake provides a cost-effective storage model that supports future analytics initiatives without requiring frequent restructuring.
"Unlike traditional databases that require immediate structuring, a data lake allows businesses to collect, retain, and process information without predefined schemas."
13 data lake advantages and disadvantages
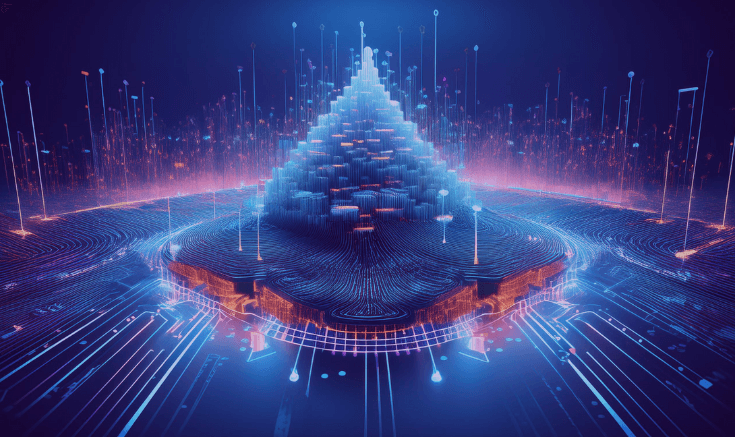
Organizations generate vast amounts of structured and unstructured data from multiple sources, including application logs, IoT devices, financial transactions, and customer interactions. Managing this data efficiently requires a storage solution that supports flexibility, scalability, and advanced analytics. A data lake provides a centralized repository that stores raw data in its original format, eliminating the need for immediate structuring or transformation. This approach allows businesses to analyze information based on developing needs while retaining access to historical datasets.
While a data lake offers multiple benefits, it also presents challenges that must be addressed to maximize efficiency. Without proper governance, a data lake can become disorganized, leading to inefficiencies that reduce its value. Understanding the advantages and disadvantages of a data lake helps organizations determine the best implementation strategy for their specific use cases.
Key advantages of data lakes
1. Stores all data types in a single repository
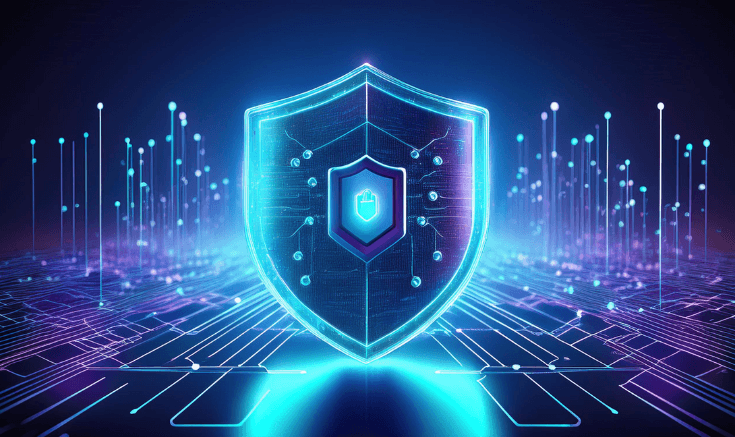
A data lake can store structured, semi-structured, and unstructured data without predefined schemas. Traditional databases impose formatting restrictions, making it difficult to accommodate raw information. A data lake removes these limitations, allowing storage of various formats, including JSON, XML, text, images, and video.
This flexibility permits businesses to collect and analyze information without worrying about compatibility issues. Retaining data in its native form allows future processing and analysis without losing valuable details.
2. Provides scalable storage at a lower cost
Large datasets require scalable storage solutions that do not introduce unnecessary expenses. A data lake optimizes costs by using cloud-based or on-premise infrastructure that scales based on usage. Unlike structured databases that require expensive hardware upgrades, a data lake can expand as needed without excessive infrastructure costs.
Cloud providers offer pay-as-you-go pricing models, reducing upfront expenses while providing access to high-capacity storage. This approach allows organizations to allocate resources efficiently, avoiding unnecessary expenditures on underutilized storage.
3. Supports machine learning and artificial intelligence applications
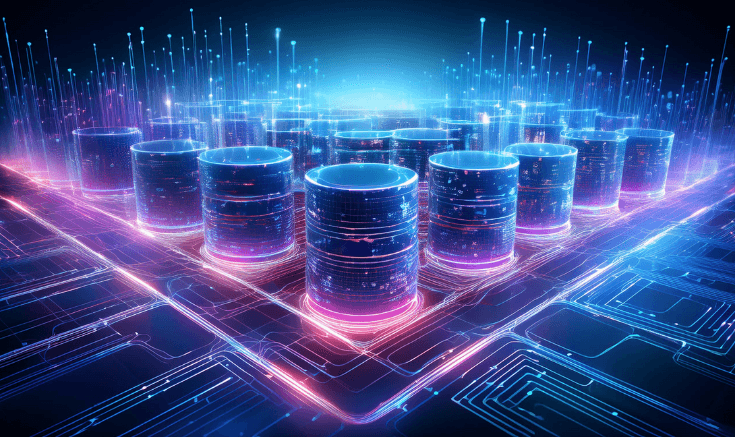
A data lake is a foundation for machine learning and artificial intelligence models, providing access to raw datasets that can be processed in multiple ways. Traditional databases limit data analysis to predefined structures, restricting exploration and experimentation. A data lake allows data scientists to apply different algorithms, enabling predictive analytics, natural language processing, and anomaly detection.
This flexibility enhances research and development efforts, allowing businesses to reveal patterns and trends that would be difficult to identify using traditional methods. Advanced analytics powered by a data lake contributes to data-based business strategies.
4. Allows real-time and historical data analysis
A data lake allows organizations to process real-time and historical data within the same repository. Traditional databases often separate transactional data from analytical workloads, requiring additional resources to integrate insights. A data lake eliminates these barriers, making it possible to run queries on live data streams while analyzing past trends.
This dual capability improves operational efficiency, supporting immediate insights and long-term strategic planning. Businesses can track customer behavior, monitor system performance, and detect anomalies in real time without needing separate storage solutions.
5. Centralizes data access for multiple teams
Data stored across multiple systems creates barriers to collaboration. A data lake centralizes storage, eliminating silos and making it easier for different teams to access relevant information. Data scientists, analysts, and business intelligence teams can work with the same datasets without creating redundant copies.
With proper governance, access permissions can be tailored based on roles, ensuring that sensitive information remains protected while maintaining transparency across departments. Centralized storage enhances productivity by reducing the time spent retrieving and consolidating data.
6. Reduces processing time for analytics workflows
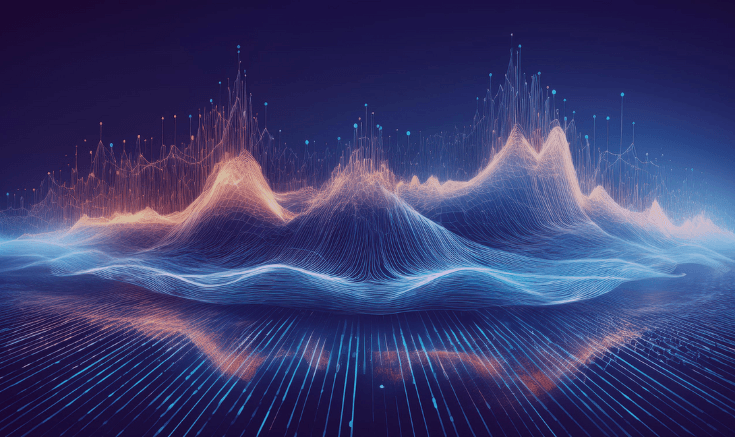
Analyzing large datasets requires significant processing power. A data lake optimizes data retrieval and analysis by allowing parallel processing across distributed storage. This approach reduces the time needed to generate reports, train machine learning models, and perform complex queries.
Organizations benefit from improved performance, allowing teams to derive insights without delays. As datasets grow, distributed processing capabilities ensure that performance remains consistent without overwhelming computing resources.
7. Improves long-term data retention strategies
Compliance requirements often mandate the retention of historical data for audits, security, or regulatory purposes. A data lake simplifies long-term storage by efficiently archiving data without frequent reformatting or restructuring. Unlike structured databases that require ongoing optimization, a data lake preserves raw data until needed.
This approach ensures businesses can access legacy datasets without incurring unnecessary processing costs. When properly managed, a data lake supports compliance initiatives while providing a reliable archive for future analysis.
Key disadvantages of data lakes
8. Requires governance to prevent disorganization
A poorly managed data lake can become challenging, leading to a "data swamp" filled with redundant, outdated, or irrelevant data. The absence of predefined schemas increases the risk of disorganization, making it harder to locate valuable datasets.
Organizations must implement metadata tagging, indexing, and access controls to keep data structured and retrievable. Without these measures, a data lake can quickly lose its value, making information retrieval inefficient.
9. Slower performance for structured queries
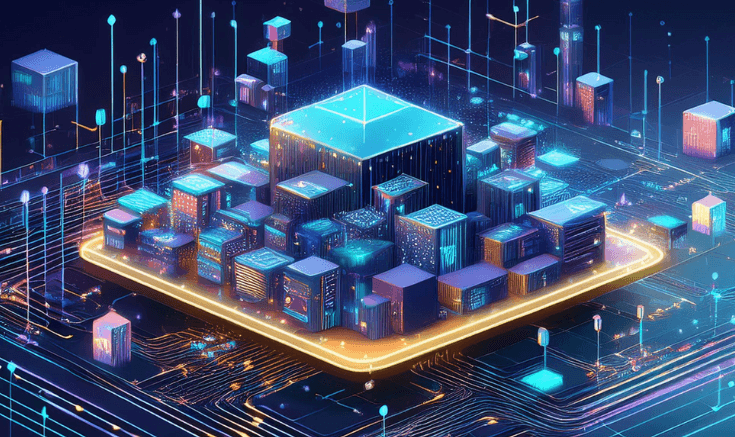
Traditional databases optimize query performance by enforcing predefined schemas and indexing strategies. A data lake, by contrast, stores data in its raw format, requiring additional processing before analysis. Complex queries can take longer to execute, especially when dealing with large datasets.
Optimizing storage architecture and implementing caching mechanisms can mitigate these challenges. However, businesses relying on real-time analytics may need additional solutions to enhance query speeds.
10. Higher storage costs for excessive data retention
Although a data lake provides cost-effective scalability, unregulated storage practices can increase expenses. Storing unnecessary datasets without lifecycle management raises cloud costs and compliance risks.
Implementing automated data retention policies helps control expenses by archiving or deleting outdated records. Without proper oversight, data lakes can accumulate excessive information, increasing costs without delivering corresponding value.
11. Complexity in integrating with existing infrastructure
Legacy systems designed for structured databases may require additional processing layers to interface with a data lake. Existing analytics tools and reporting platforms may not support raw data formats, creating integration challenges.
A hybrid approach that combines a data lake with structured data warehouses can improve compatibility. Establishing clear data pipelines and transformation workflows ensures seamless interoperability between systems.
12. Increased costs for data processing and indexing
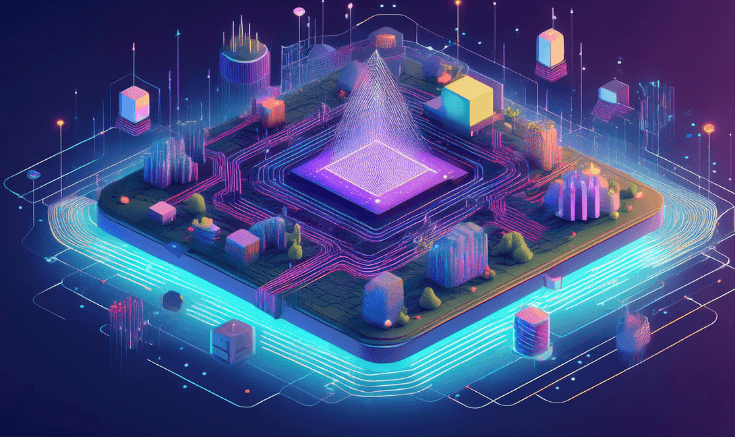
Extracting insights from raw data often requires additional processing, indexing, and transformation steps. These processes introduce computational costs that may offset initial storage savings.
Implementing efficient data indexing techniques and optimizing storage layers reduces processing overhead. Organizations may experience inefficiencies when working with large-scale analytics workloads without these strategies.
13. Requires skilled professionals for maintenance
Managing a data lake requires expertise in cloud storage, distributed processing, and governance frameworks. Businesses without a dedicated team may struggle to maintain an efficient storage and retrieval system.
Hiring data engineers and architects is essential for maintaining system performance. Without proper expertise, a data lake can become inefficient, increasing maintenance costs. A data lake provides flexibility, scalability, and cost-efficient storage for organizations managing vast amounts of structured and unstructured data. It authorizes advanced analytics, supports artificial intelligence applications, and allows real-time processing while maintaining historical records. However, improper management can result in inefficiencies that reduce usability, increase costs, and introduce security risks.
A structured approach that includes governance policies, access controls, and optimized storage strategies ensures that a data lake remains a valuable asset rather than a liability. Businesses that implement best practices can maximize the benefits while mitigating potential challenges, allowing them to extract insights, improve operational efficiency, and maintain compliance with industry regulations.
"Understanding the advantages and disadvantages of a data lake helps organizations determine the best implementation strategy for their specific use cases."
Data lake examples by industry
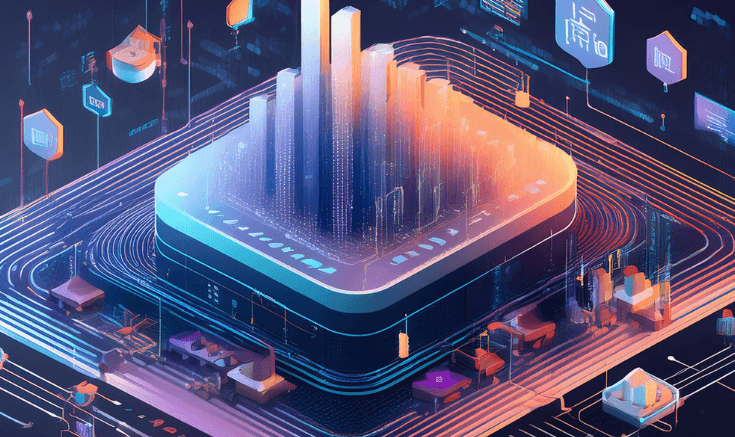
Organizations across industries generate vast amounts of structured and unstructured data that require effective storage, processing, and analysis. Traditional databases often impose rigid structures, limiting the ability to efficiently store and analyze raw datasets. A data lake provides a scalable solution that retains data in its original format, allowing advanced analytics, artificial intelligence, and machine learning applications to extract valuable insights. This approach enhances operational efficiency, supports predictive modeling, and lets organizations centralize vast data sources without compromising flexibility.
- Financial services - Fraud detection and risk assessment: Banks and financial institutions process millions of transactions daily, requiring advanced analytics to detect fraudulent activity. A data lake aggregates transaction records, behavioral data, and geolocation tracking, allowing fraud detection systems to identify anomalies and suspicious patterns in real time. Credit scoring models evaluate financial history by analyzing structured and unstructured data sources, helping lenders assess risks and prevent financial losses. Regulatory reporting improves as compliance teams access centralized data without delays.
- Healthcare - Predictive diagnostics and patient analytics: Hospitals and medical research institutions collect data from electronic health records, wearable devices, and diagnostic imaging. A data lake consolidates these sources, allowing artificial intelligence models to analyze genetic information, identify disease risks, and recommend personalized treatment plans. Patient monitoring benefits from predictive analytics, supporting early intervention strategies that reduce hospital readmissions and improve long-term health outcomes.
- Retail and e-commerce - Customer behavior and inventory forecasting: Retailers track consumer purchase history, browsing habits, and demand fluctuations to optimize pricing, inventory levels, and marketing campaigns. A data lake supports machine learning models that analyze shopping patterns, refine product recommendations, and personalize customer engagement strategies. Supply chain operations benefit from demand forecasting models that predict stock levels and prevent inventory shortages, reducing costs while improving availability.
- Manufacturing - IoT-based predictive maintenance and production efficiency: Factories and production facilities generate continuous data streams from industrial sensors, machine logs, and quality control systems. A data lake processes these data points, allowing predictive maintenance models to detect equipment failures before they occur. Production schedules improve as historical performance data informs efficiency optimizations, minimizing downtime and extending the lifespan of critical machinery.
- Media and entertainment - Audience engagement and content recommendations: Streaming platforms, social media companies, and digital publishers analyze content consumption patterns to optimize user experiences. A data lake centralizes video streams, user reviews, and interaction metrics, allowing machine learning models to tailor recommendations and refine advertising strategies. Audience segmentation enhances targeted marketing, improving viewer retention and monetization opportunities.
- Energy and utilities - Grid optimization and renewable energy forecasting: Power providers analyze energy consumption data from smart meters, weather models, and infrastructure sensors. A data lake aggregates these inputs to improve power distribution, detect outages, and forecast energy needs. Renewable energy planning benefits from predictive analytics that assess solar and wind production trends, supporting sustainability initiatives while reducing operational inefficiencies.
- Transportation and logistics - Route optimization and fleet efficiency: Logistics providers, airlines, and shipping companies track fleet performance, delivery schedules, and GPS-based routing data. A data lake consolidates this information, allowing artificial intelligence models to optimize delivery routes, minimize fuel consumption, and reduce delays. Warehouse operations integrate historical shipping data to enhance supply chain planning, improving order fulfillment and shipment tracking accuracy.
A data lake provides scalable storage, advanced analytics capabilities, and centralized access to structured and unstructured data. Organizations benefit from improved operational efficiency, enhanced risk assessment, and cost-effective resource management. Businesses implementing structured governance strategies prevent data swamps, ensuring information remains accessible and actionable. By integrating a data lake with analytics platforms and machine learning models, industries maximize the value of their data assets, improving workflows and unlocking new opportunities for innovation.
Data lakes offer a scalable and efficient way to manage large volumes of structured and unstructured data, permitting businesses to extract insights that drive strategy and innovation. A structured governance and storage optimization approach ensures data remains accessible, secure, and cost-effective. Lumenalta specializes in tailored data solutions that align with business goals and deliver measurable impact. Let’s build a data strategy that maximizes your potential.
table-of-contents
- What is a data lake?
- 13 data lake advantages and disadvantages
- 1. Stores all data types in a single repository
- 2. Provides scalable storage at a lower cost
- 3. Supports machine learning and artificial intelligence applications
- 4. Enables real-time and historical data analysis
- 5. Centralizes data access for multiple teams
- 6. Reduces processing time for analytics workflows
- 7. Improves long-term data retention strategies
- 8. Requires governance to prevent disorganization
- 9. Slower performance for structured queries
- 10. Higher storage costs for excessive data retention
- 11. Complexity in integrating with existing infrastructure
- 12. Increased costs for data processing and indexing
- 13. Requires skilled professionals for maintenance
- Data lake examples
- Common questions about data lake
Common questions about data lakes
What are the primary advantages of a data lake?
How does a data lake differ from a data warehouse?
What industries benefit the most from using a data lake?
What are the security risks of a data lake?
How does a data lake support artificial intelligence and machine learning?
Want to learn how data lakes can bring more transparency and trust to your operations?