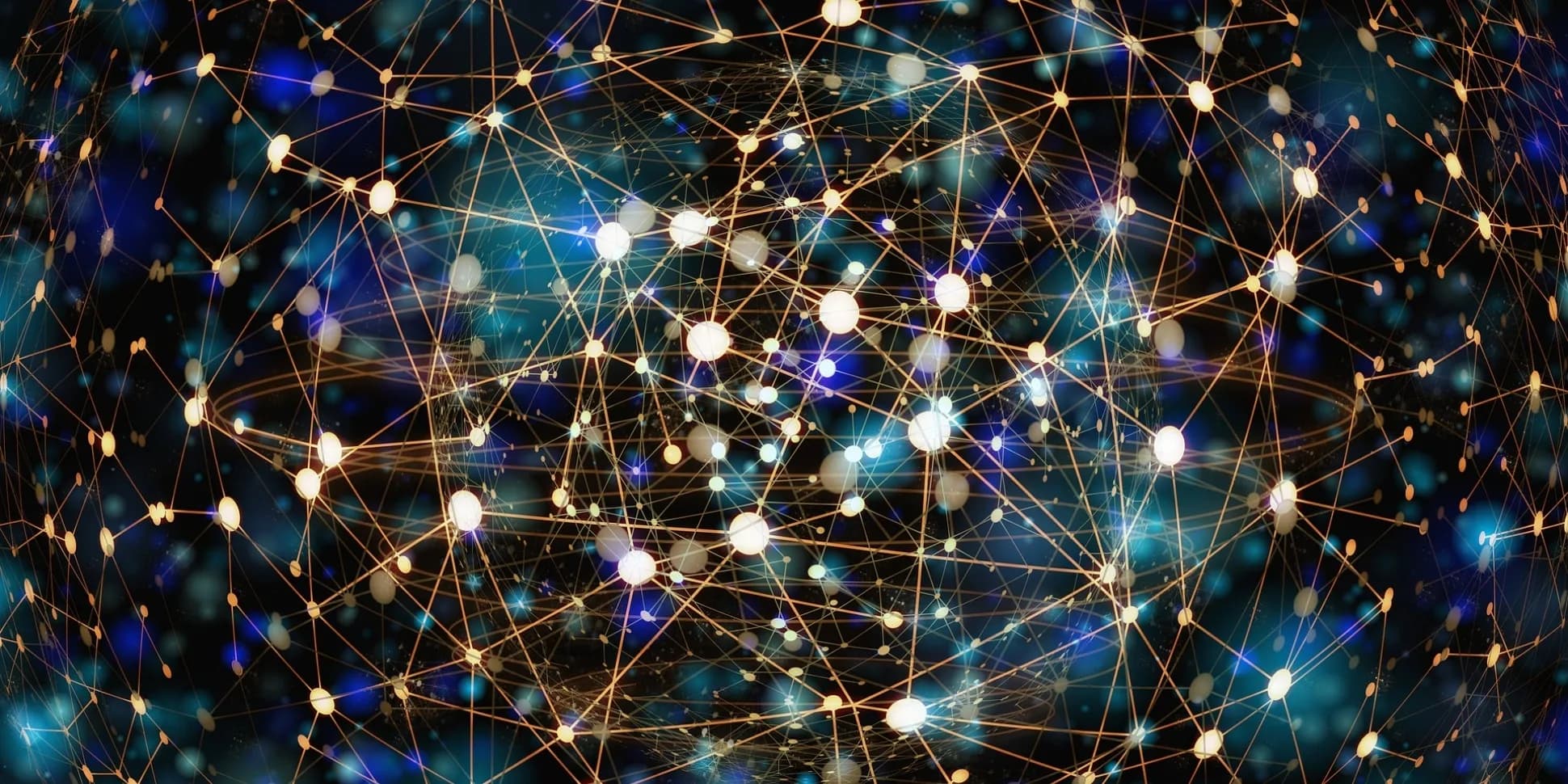
How to measure ROI for data science in business
AUG. 14, 2024
2 Min Read
Here’s a data-driven approach to measuring the ROI of your data science projects.
How to measure ROI for data science in business
Data science initiatives have nearly unbounded potential to transform businesses. We’ve seen companies turbocharge operational efficiency, slash fraud rates through real-time anomaly detection, and improve the customer experience in all sorts of ways.
The opportunity is immense, yet many organizations struggle to quantify the return on their data science investments. This leaves leaders questioning whether their efforts are truly paying off.
Intangible benefits are important, but demonstrating the financial return on your investment is necessary for securing continued support and resources.
Define clear business objectives upfront
To get the most out of your data science projects, your initiatives must be interwoven with your core business objectives from the start. Doing so makes it less likely that they’ll veer off course.
Once you’ve defined objectives that closely align with your business priorities, consider what problems you’re trying to solve or opportunities you want to seize.
Are you looking to boost marketing campaigns? Optimize inventory?
Don’t try to boil the ocean — pinpoint the areas that will really make a difference to your business.
And regardless of what you’re trying to achieve, be sure to set specific and measurable targets. Instead of ‘streamline operations,’ set a goal of ‘reducing average order fulfillment time by 15% within six months.’
This level of specificity allows you to track progress accurately and determine whether you’re delivering the desired results.
Measure current baseline performance
Just as scientists establish a control variable to measure experimental results, data science projects require a baseline for comparison. This benchmark provides a snapshot of your organization’s starting point that gives you a better sense of the impact of your initiatives.
Measure baseline performance by identifying key performance indicators (KPIs) that align with your business objectives.
Some common KPIs include revenue, customer acquisition cost, churn rate, and production efficiency. These metrics should be quantifiable and reflect the areas you aim to improve.
Monitor KPIs after deployment of data science models
The work doesn’t stop once your data science models go live. Continuous monitoring of your KPIs is essential to gauge the real-world impact of your initiatives. Consistently evaluate how your models are performing and compare the results against your established baseline.
- Are conversion rates climbing?
- Is customer churn declining?
- Have operational costs decreased?
Tracking these changes allows you to directly attribute improvements to your data science efforts, solidifying their value to the organization.
Along with validating the effectiveness of your models, ongoing monitoring can provide valuable insights for continuous improvement. Use these insights to refine and optimize your models over time.
Related: How to avoid SaaS data fragmentation
Calculate the monetary value of improvements
Once you’ve identified progress on your KPIs, it’s time to figure out the economic impact of these performance improvements.
Good data science projects deliver plenty of benefits that can’t be measured in dollars and cents, but calculating the financial ROI from your efforts is still imperative. It demonstrates their value to your CEO and gives you the green light for future projects.
Let’s say your data science model enabled more effective marketing campaigns, which led to increased sales. Calculate the increase in revenue attributable to the model. If it’s 5%, apply that to your total sales figures to estimate the monetary gain.
Financial improvements can extend beyond the top line, though. Your efforts could focus on cost savings through streamlined operations or optimized inventory management.
For instance, if your data science application led to a decrease in customer churn, calculate the lifetime value of those retained customers and the resulting increase in revenue. Alternatively, if your model improved fraud detection, estimate the financial losses prevented by identifying and stopping fraudulent activity.
Account for development & deployment costs
A comprehensive ROI evaluation requires more than just tallying up financial gains. It demands a thorough assessment of all associated costs, many of which can easily go unnoticed.
Consider your initial investment in data acquisition, model development, testing, and deployment. And remember ongoing expenses like maintenance, infrastructure, and the salaries of your data science team. These costs can quickly add up, impacting the overall return on your investment.
Accurately accounting for expenditures like these allows you to calculate the net ROI, which provides a more precise understanding of the true financial performance of your projects.
Build value attribution models
Unraveling the specific impact of your data science efforts can be tricky.
- Did that uptick in sales come from your new recommendation engine, or was it simply a seasonal trend?
- How much did your fraud detection model actually save you?
Data science analytics are indispensable here, particularly value attribution models. They can be instrumental in isolating the precise financial impact of your data science endeavors.
Using predictive models to quantify value attribution
Value attribution requires separating signal from noise, and predictive modeling techniques offer a powerful way to do just that. This approach leverages historical data and relevant variables to isolate and quantify the impact of your initiatives on desired business outcomes.
For instance, regression analysis can estimate the specific contribution of your data science model to revenue growth, separating its impact from other influencing factors like market trends or seasonal fluctuations. Controlling for these external variables pinpoints the exact financial value your model adds to the bottom line.
To ensure the reliability of your predictive models, it’s crucial to adhere to best practices such as cross-validation and model validation. These techniques assess the accuracy and robustness of your models, ensuring they deliver accurate insights into the true impact of your data science efforts.
Advanced techniques like controlled experiments
Controlled experiments offer a more rigorous approach to measuring the causal impact of data science initiatives.
These experiments, such as A/B testing or randomized trials, involve dividing your audience into two groups: a treatment group exposed to the data science model and a control group that isn’t. Random assignment ensures that both groups are comparable, allowing you to isolate the effect of the data science model on specific outcomes.
For example, an e-commerce company could use an A/B test to evaluate the impact of a personalized recommendation algorithm on sales. The company could directly measure the algorithm’s impact on revenue by comparing the performance of the treatment group versus the control group.
Techniques like matching methods further refine this approach by ensuring that the treatment and control groups have similar characteristics. This strengthens the validity of your conclusions and provides even more precise insights into the causal impact of your model.
Set target ROI thresholds
Data science ROI goals shouldn’t be pulled out of thin air. To boost the credibility of your data science efforts, collaborate with other leaders in your business to establish realistic ROI targets for each initiative.
These goals should mirror your overarching business objectives and serve as a tangible way to assess whether your investments are paying off.
Regularly review the performance of your projects against these ROI goals to ensure initiatives remain on track. If a project falls short of expectations, it’s an opportunity to reassess, adjust strategies, or explore alternative approaches to maximize your return on investment.
Ensuring data science projects deliver
At the end of the day, data science for business needs to deliver a compelling ROI.
A data-driven approach, along with the right expertise, can empower your organization to meticulously track performance, demonstrate business value, and make informed decisions about future investments.
A data engineering expert like Lumenalta can guide you on this journey. Our team of senior engineers boasts years of experience in data science research and is here to help you design and implement scalable, secure data systems that support your technical and business teams to demonstrate the data science initiative’s ROI to stakeholders.
Read next: CIO’s guide to data governance strategies