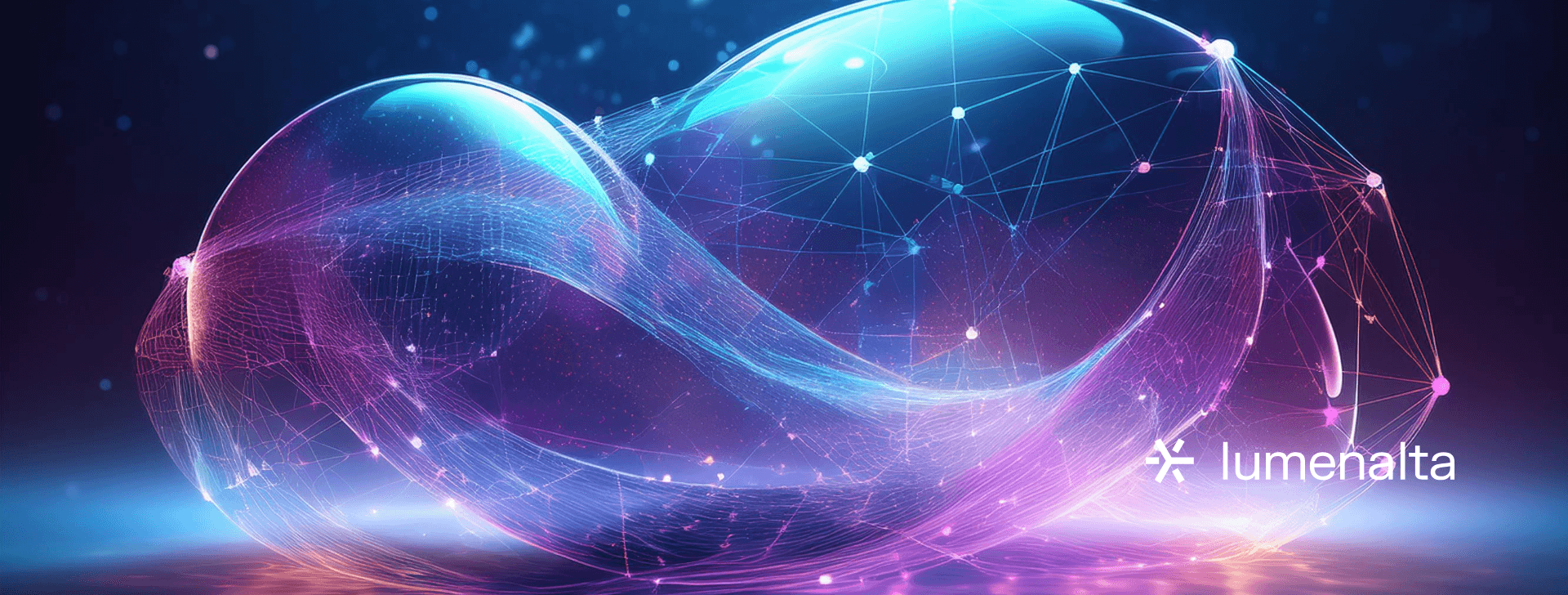
Responsible AI checklist (updated 2025)
MAR. 7, 2025
4 Min Read
AI-powered systems are shaping industries, yet ethical concerns surrounding bias, transparency, and accountability continue to escalate.
Machine learning models influence business strategies, automate processes, and analyze vast data sets, but without structured safeguards, they can produce misleading results, reinforce discrimination, or introduce privacy risks. Companies implementing AI must take responsibility for fairness, accuracy, and governance to minimize unintended consequences. Responsible AI frameworks provide organizations with structured methodologies to ensure AI applications remain ethical, compliant, and aligned with business objectives. Addressing bias, documenting model behavior, and integrating human oversight help maintain accountability across AI-based decisions. Without these safeguards, businesses risk financial penalties, reputational damage, and loss of consumer trust. Establishing ethical AI practices is not just a legal requirement—it is a necessary strategy for sustainable, risk-conscious innovation.
Key Takeaways
- 1. Responsible AI frameworks help businesses mitigate risks related to bias, transparency, and regulatory compliance.
- 2. Bias assessments, model documentation, and explainability techniques improve fairness and accountability in AI systems.
- 3. Privacy safeguards and data protection measures prevent unauthorized access, reducing legal and reputational risks.
- 4. Continuous monitoring and human oversight are essential to maintaining ethical AI performance over time.
- 5. Organizations that implement structured AI governance build trust, minimize liabilities, and improve long-term AI adoption strategies.
Understanding responsible AI
"Responsible AI prioritizes ethical safeguards that reduce bias, improve transparency, and maintain compliance with developing regulations."
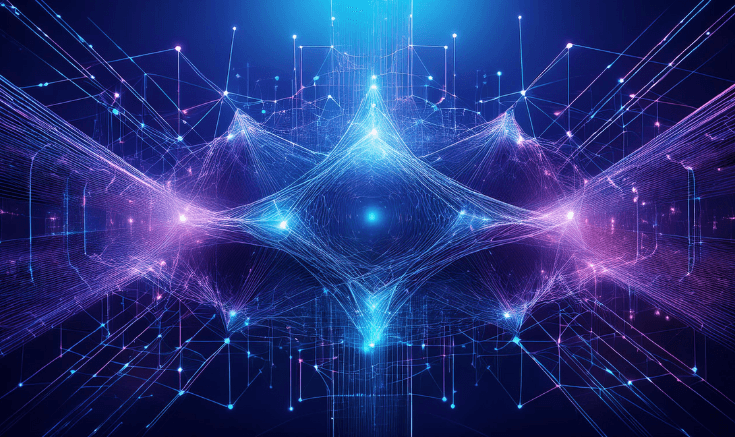
Artificial intelligence is expanding across industries, streamlining operations, and analyzing complex data sets at unprecedented speeds. Companies are adopting AI-powered systems to improve efficiency, automate processes, and extract insights that influence critical business strategies. However, ethical concerns surrounding fairness, transparency, and accountability continue to grow. Algorithmic bias, privacy risks, and unintended consequences can introduce financial, operational, and reputational challenges. AI models may reinforce discrimination, produce misleading outcomes, or expose organizations to regulatory scrutiny without a responsible framework. As reliance on machine learning increases, businesses must adopt structured methodologies to guide ethical AI implementation.
Responsible AI prioritizes ethical safeguards that reduce bias, improve transparency, and maintain compliance with developing regulations. Machine learning models must operate within ethical boundaries that align with legal obligations and business goals. AI governance frameworks address concerns related to fairness, model explainability, and unintended consequences, helping organizations mitigate legal risks while maintaining stakeholder confidence.
A commitment to responsible AI strengthens brand reputation, minimizes liabilities, and supports long-term business sustainability. Organizations that integrate fairness principles into AI development enhance reliability, build consumer trust, and maintain compliance with industry regulations. Ethical AI adoption is not just a regulatory necessity—it is a strategic approach that fosters responsible innovation while protecting both business interests and the wider user community.
Importance of a responsible AI checklist
AI-powered systems are essential in automating operations, analyzing large-scale data, and improving efficiency across industries. However, without structured oversight, these systems can introduce unintended ethical risks. Algorithmic bias, security vulnerabilities, and opaque management processes can lead to financial losses, reputational damage, and regulatory penalties. A structured framework is necessary to mitigate these risks while ensuring AI initiatives align with business objectives and ethical responsibilities.
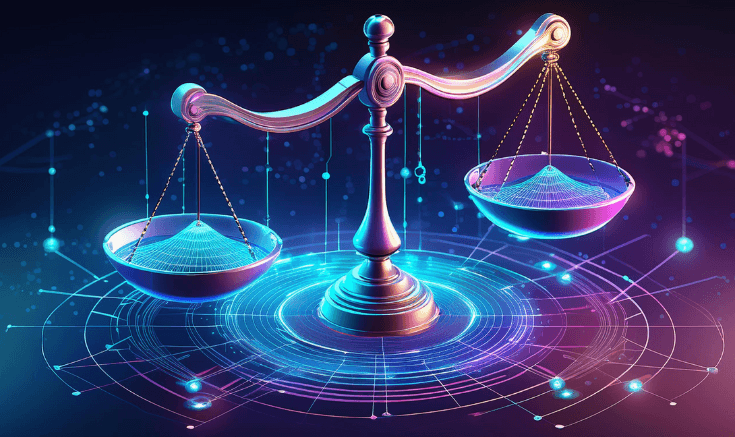
A responsible AI checklist provides a clear methodology for evaluating and implementing AI models with fairness, accountability, and transparency. It serves as a guiding tool that helps organizations identify gaps in governance, assess potential biases, and improve compliance with regulatory standards. AI deployments may reinforce discrimination, produce misleading results, or compromise user privacy without predefined ethical guidelines.
Adopting a responsible AI checklist improves long-term business viability by reducing operational risks and strengthening stakeholder trust. Organizations that follow a structured approach to ethical AI implementation can enhance reliability, demonstrate regulatory compliance, and safeguard user confidence. Establishing these safeguards is necessary for legal adherence and sustaining credibility in an AI-dependent business environment.
Preparing for responsible AI
AI adoption continues to expand across industries, optimizing processes and unlocking new efficiencies. However, ethical concerns must be addressed before deploying machine learning models into production. AI systems influence hiring practices, lending decisions, healthcare diagnostics, and other high-stakes applications. Without proactive planning, these models may introduce bias, compromise data privacy, or lack transparency in regulation. Responsible AI implementation starts with comprehensive preparation, ensuring ethical considerations are integrated.
Preparation begins with defining ethical principles that align with business objectives and regulatory obligations. Organizations must assess AI models for potential biases, implement safeguards to protect sensitive data and establish governance frameworks that promote accountability. Cross-functional collaboration between data scientists, compliance teams, and business leaders helps integrate fairness principles into AI workflows. Ethical guidelines should be documented, ensuring AI development meets transparency, reliability, and fairness standards.
Ongoing evaluation is necessary to maintain responsible AI practices throughout an AI system’s lifecycle. Regular audits, bias assessments, and user feedback mechanisms help organizations identify risks and refine models accordingly. A structured preparation approach minimizes legal and reputational risks and enhances the effectiveness of AI-backed initiatives. Businesses prioritizing responsible AI from the planning phase can improve trust, reduce liabilities, and sustain long-term AI adoption without ethical compromises.
Checklist for responsible AI (updated 2025)
Implementing responsible AI requires a structured approach to mitigate risks, improve transparency, and maintain ethical integrity. This checklist provides a practical guide for organizations to assess AI models, address potential biases, and align with regulatory requirements. A proactive strategy helps businesses avoid unintended consequences while strengthening trust with users and stakeholders.
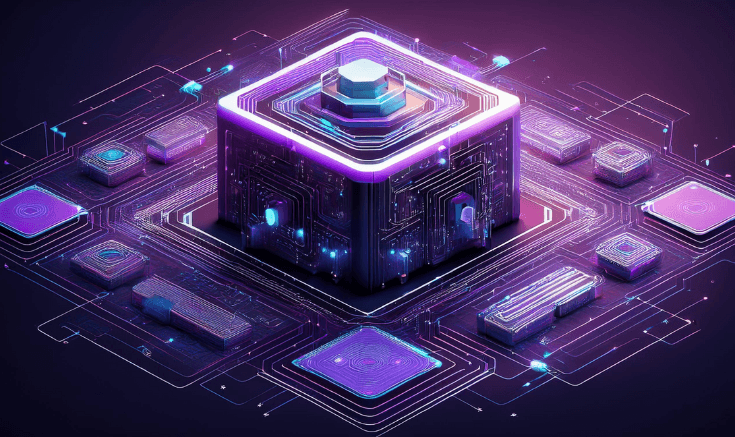
"Ongoing evaluation is necessary to maintain responsible AI practices throughout an AI system’s lifecycle."
- Establish clear ethical principles
- Define fairness, transparency, and accountability standards that align with business goals and regulatory obligations.
- Document ethical guidelines and integrate them into AI development workflows.
- Conduct bias assessments before deployment
- Analyze training data and model outputs to identify and mitigate biases that could lead to unfair or discriminatory outcomes.
- Perform regular evaluations to confirm AI systems operate within ethical boundaries.
- Implement transparent model documentation
- Maintain clear and comprehensive records detailing AI model training, data sources, and evaluation processes.
- Improve regulatory compliance and user trust by ensuring transparency in AI models.
- Apply explainability techniques
- Make AI-driven decisions interpretable and justifiable for both technical and non-technical stakeholders.
- Use explainability tools to validate model fairness and improve accountability.
- Secure user data and privacy
- Adopt strict data protection measures to prevent unauthorized access, leaks, or misuse.
- Ensure AI systems comply with data privacy laws and industry-specific regulations.
- Establish human oversight mechanisms
- Introduce human review processes to monitor AI decisions, especially in high-stakes applications such as finance, healthcare, and hiring.
- Reduce risks associated with automated errors through structured oversight.
- Monitor AI performance continuously
- Regularly evaluate AI models for accuracy, fairness, and unintended biases.
- Implement ongoing monitoring processes to detect issues early and make necessary adjustments.
- Align AI governance with legal and compliance frameworks
- Ensure AI initiatives comply with local, national, and industry-specific regulations.
- Prevent legal liabilities and regulatory penalties through structured governance.
- Implement AI audit trails
- Maintain logs of AI-driven decisions to support accountability and traceability.
- Use audit trails to gain insights into model performance and address compliance inquiries.
- Incorporate diverse stakeholder input
- Engage with various teams, including ethicists, policymakers, and impacted user groups, to refine AI strategies.
- Use inclusive feedback to improve fairness and reduce unintentional biases.
- Develop an AI incident response plan
- Prepare for unintended AI failures or biases by establishing a clear remediation process.
- Minimize negative business impacts and reinforce accountability through rapid response strategies.
- Educate employees on responsible AI practices
- Provide ongoing training for teams working with AI to reinforce ethical best practices.
- Implement awareness programs to maintain responsible AI deployment across all business functions.
A structured checklist helps businesses implement AI ethically while mitigating financial, regulatory, and reputational risks. Following these principles fosters responsible innovation, strengthens compliance efforts, and improves trust in AI-powered solutions.
Implementing fairness in AI
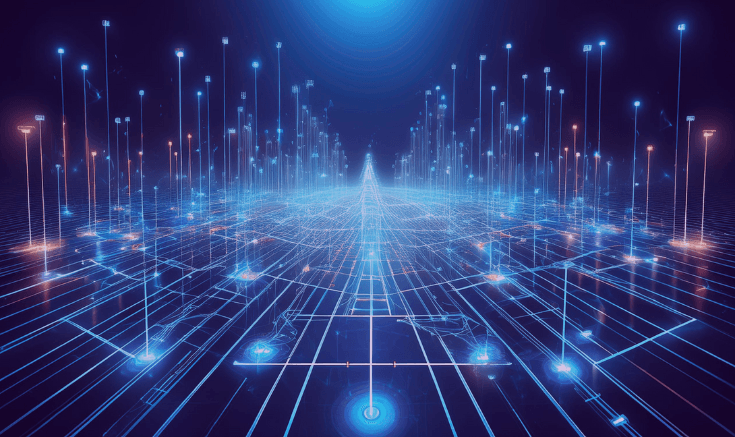
AI models influence hiring processes, financial decisions, medical diagnoses, and other critical applications. If fairness is not prioritized, these systems can reinforce discrimination, exclude marginalized groups, or produce biased results. Addressing fairness requires proactive intervention at every stage of AI development, from data collection to deployment. Organizations that integrate fairness principles into AI workflows reduce legal risks and maintain public trust.
Bias in AI often originates from imbalanced training data or flawed algorithms. Mitigating these risks starts with data audits to identify disparities and ensure varied representation in datasets. Statistical techniques such as reweighting, adversarial debiasing, and fairness-aware training can reduce bias before models are deployed. Algorithmic transparency facilitates fairness, allowing stakeholders to scrutinize decision logic and identify potential biases.
Human oversight strengthens fairness by introducing ethical review processes that complement algorithmic assessments. Multidisciplinary teams should participate in AI evaluations, including ethicists, legal experts, and affected user groups. Regular monitoring and fairness audits help organizations detect new biases as models interact with developing data sets. Businesses adopting these strategies improve AI systems' reliability while reinforcing equitable outcomes for all users.
Responsible AI is more than compliance—it’s a commitment to ethical, transparent, and accountable innovation. Businesses implementing fairness principles and structured oversight create AI systems that build trust while delivering measurable value. At Lumenalta, we specialize in AI solutions that align with ethical best practices, ensuring your AI initiatives remain fair, reliable, and scalable. Let’s chart a brighter path forward.
Common questions about responsible AI
What is a responsible AI checklist?
How can businesses prevent AI bias?
Why is transparency important in AI systems?
How does responsible AI improve compliance?
What role does human oversight play in responsible AI?
Want to learn how AI can bring more transparency and trust to your operations?