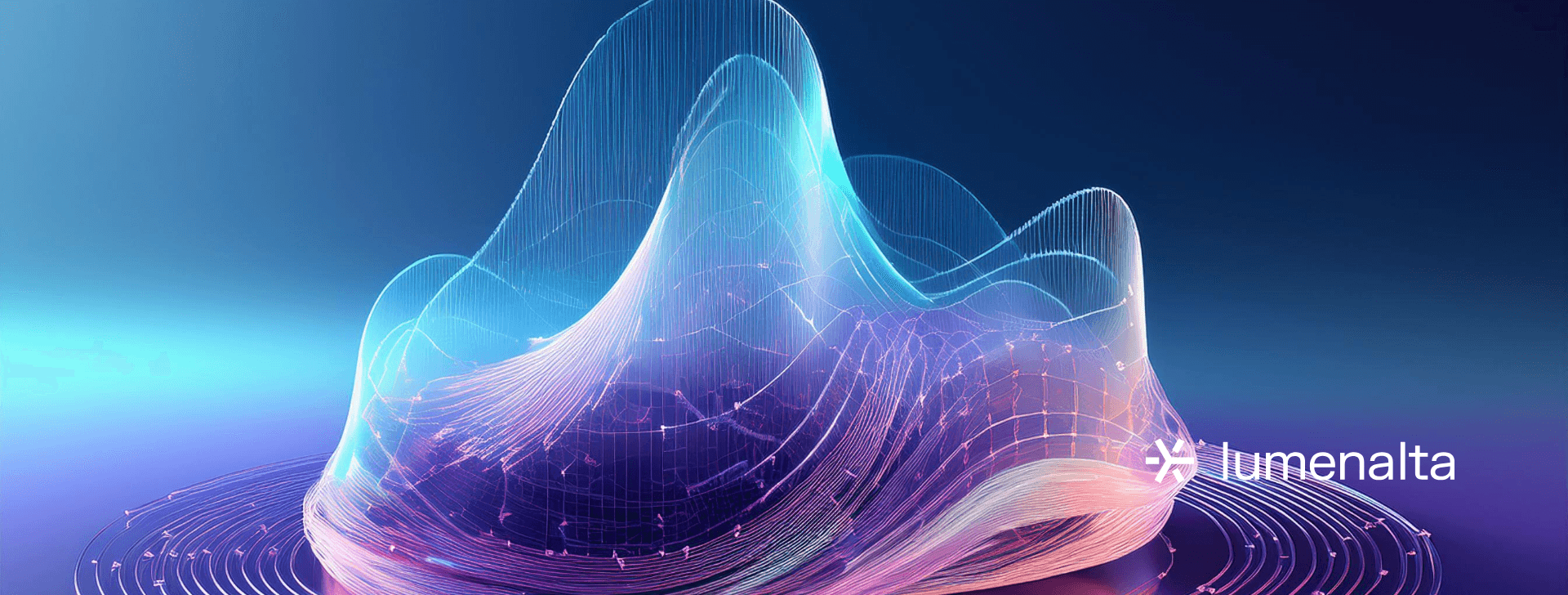
7 data lake solutions in 2025
FEB. 3, 2025
4 Min Read
Data lake solutions provide the scalability, flexibility, and efficiency needed to manage large volumes of structured and unstructured data
Organizations rely on these solutions to consolidate information from multiple sources, making data more accessible for analytics, artificial intelligence, and regulatory compliance. Without a structured approach, businesses risk high storage costs, security vulnerabilities, and inefficient data retrieval. Selecting the right data lake solution and optimizing implementation strategies can significantly impact cost efficiency, governance, and the ability to extract meaningful insights.
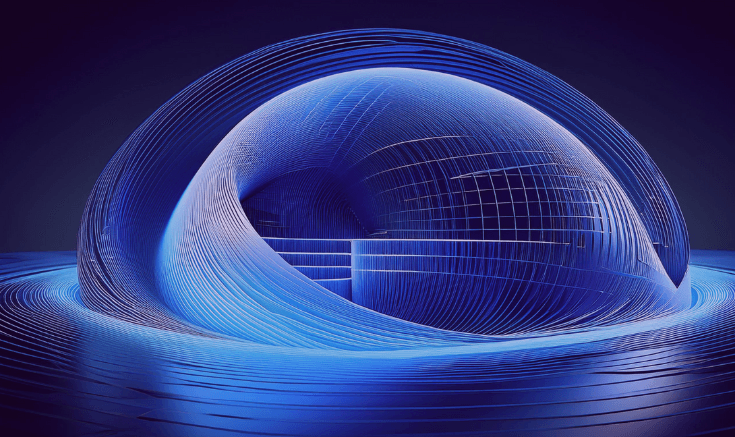
Key takeaways
- 1. A data lake solution consolidates structured and unstructured data, improving analytics, governance, and cost efficiency.
- 2. On-premise solutions provide complete control over security and compliance, while cloud-based options offer scalability and reduced infrastructure management.
- 3. Optimizing storage with a tiered model balances performance and cost while maintaining accessibility for analytics.
- 4. Integrating machine learning and automation tools enhances predictive capabilities and streamlines operational workflows.
- 5. Governance frameworks, including encryption and role-based access controls, strengthen data security and compliance efforts.
What are data lake solutions
"Organizations managing vast amounts of structured and unstructured data need scalable storage solutions that integrate seamlessly with analytics platforms."
Organizations generate vast amounts of structured and unstructured data across various systems, applications, and digital channels. Managing this volume while extracting valuable insights requires a scalable and flexible storage solution. Traditional databases impose rigid structures that limit adaptability, making storing and processing raw data efficiently difficult. Data lake solutions address this challenge by providing a centralized repository that accommodates information in its original format, allowing businesses to store, access, and analyze data without the constraints of predefined schemas.
Data lake solutions support a wide range of use cases, including analytics, artificial intelligence, and operational intelligence. This approach allows organizations to integrate various data sources, such as IoT sensor outputs, log files, social media interactions, and transactional records. Instead of filtering or converting data before ingestion, these solutions retain full datasets, preserving their integrity for advanced analytics and future use cases. The flexibility of this model improves cost efficiency, enhances governance strategies, and simplifies regulatory compliance.
Selecting the best data lake solutions depends on scalability, integration capabilities, and governance frameworks. The right solution enhances business intelligence, accelerates time to insights, and optimizes operational efficiency. Data lake solution improves accessibility, supports advancing business needs, and maximizes the long-term value of data assets.
7 data lake solutions in 2025
Organizations managing vast amounts of structured and unstructured data need scalable storage solutions that integrate seamlessly with analytics platforms. Data lake solutions provide flexible storage architectures that allow businesses to consolidate information from multiple sources while maintaining governance, security, and accessibility. These platforms optimize analytics workflows, support machine learning models, and enhance regulatory compliance, making them essential for industries handling large-scale data operations. The best data lake solutions in 2025 combine performance, cost efficiency, and seamless integration with existing technology stacks. Some prioritize cloud-based scalability, while others focus on hybrid and on-premise deployments.
1. Amazon S3-based data lake solution
Amazon Simple Storage Service (S3) remains one of the most widely adopted cloud-based data lake solutions due to its scalable architecture and deep integration with analytics tools. Organizations can store structured and unstructured data while utilizing AWS Glue, Amazon Athena, and Amazon Redshift Spectrum to process and query datasets.
Advanced security features, including encryption and role-based access controls, provide a strong governance framework. A pay-as-you-go pricing model allows businesses to optimize storage costs while supporting high-performance analytics workloads.
2. Google Cloud Storage for data lakes
Google Cloud Storage offers a scalable, cost-effective infrastructure for high-volume data processing. Multi-region redundancy enhances data availability, while built-in lifecycle policies allow businesses to manage storage costs efficiently.
Integration with BigQuery, Dataflow, and Dataproc tools accelerates analytics and machine learning workflows. Automated classification and security tools help maintain compliance with industry regulations, making this an ideal solution for organizations focused on governance and operational efficiency.
3. Snowflake Data Cloud
Snowflake’s fully managed data lake solution eliminates the complexities of infrastructure management while supporting structured, semi-structured, and unstructured data. The platform provides a highly scalable compute layer for analytics, allowing organizations to allocate resources dynamically based on workload requirements.
Performance optimizations, including automatic indexing and query acceleration, improve efficiency. Multi-cloud compatibility lets businesses maintain flexibility in their data strategies while ensuring seamless data sharing across cloud providers.
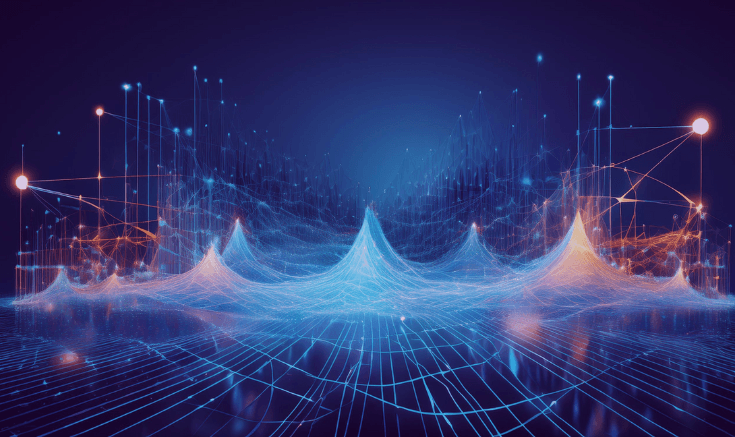
4. Databricks Lakehouse Platform
Databricks offers a unified data lakehouse architecture that supports large-scale analytics, machine learning, and streaming data processing. Built on Apache Spark, this solution combines the scalability of data lakes with the performance of data warehouses, providing a structured yet flexible analytics environment.
Optimized storage, high-performance querying, and built-in security controls make this platform well-suited for organizations that require robust data governance. The ability to process structured and unstructured data efficiently enhances its usability across multiple industries.
5. Cloudera Data Platform
Cloudera Data Platform (CDP) delivers a hybrid data lake solution designed for enterprises that require strong governance, scalability, and security. Built on open-source technologies such as Apache Hadoop and Apache Iceberg, CDP supports both on-premise and cloud deployments.
Multi-function analytics capabilities allow businesses to run batch, real-time, and machine learning workloads in a unified environment. Integrated governance tools simplify policy enforcement, ensuring compliance with industry regulations.
6. Oracle Cloud Infrastructure Data Lake
Oracle Cloud Infrastructure (OCI) provides a data lake solution for businesses requiring high-performance processing combined with strong security and compliance capabilities. Deep integration with Oracle’s database and analytics tools allows enterprises to run complex queries and advanced analytics with minimal latency.
Automated security and access controls help organizations manage regulatory requirements efficiently. The platform’s hybrid and multi-cloud capabilities provide flexibility, allowing businesses to scale storage and compute resources based on operational needs.
7. Teradata VantageCloud Lake
Teradata VantageCloud Lake offers a high-performance data lake solution for advanced analytics and machine learning applications. The platform’s scalable architecture supports multi-cloud deployments while maintaining strong governance and security frameworks.
Organizations using Teradata’s solution gain access to powerful analytics tools that optimize query performance and resource allocation. The ability to process large-scale data efficiently while integrating with various cloud ecosystems makes this a strong choice for enterprises prioritizing analytics-based strategies.
Selecting the right data lake solution requires evaluating scalability, integration capabilities, and governance frameworks. The best platforms in 2025 prioritize cost-effective storage, security, and seamless interoperability with analytics tools. Whether an organization requires a cloud-native, hybrid, or on-premise data lake solution, these options provide the flexibility and performance necessary to support long-term data strategies.
On-premise vs cloud data lake solutions
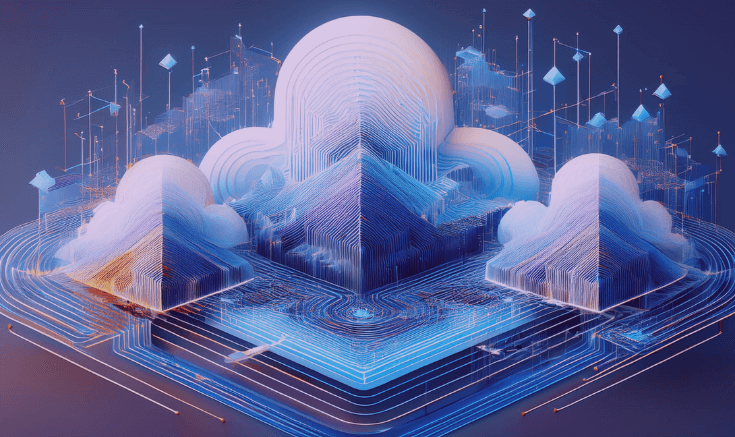
The main difference between on-premise and cloud data lake solutions lies in infrastructure ownership, scalability, and cost management. On-premise solutions require businesses to manage their storage, security, and compute resources, while cloud-based options provide a flexible, pay-as-you-go model with built-in scalability.
On-premise data lake solution offers greater control over data security and compliance, making it a preferred choice for industries with strict regulatory requirements. Organizations with legacy systems or highly sensitive data benefit from keeping storage and processing infrastructure within their data centers. However, the upfront investment in hardware, ongoing maintenance costs, and capacity limitations make on-premise solutions less adaptable for businesses with fluctuating data needs.
Cloud data lake solution provides unlimited storage and processing power, allowing businesses to scale resources based on demand. Integrating seamlessly with cloud-based analytics, artificial intelligence, and automation tools enhances efficiency. Cloud providers handle infrastructure maintenance, security updates, and disaster recovery, reducing the operational burden on IT teams. While cloud storage offers flexibility, ongoing costs can vary based on data retrieval, compute usage, and compliance-related expenses.
Choosing between on-premise and cloud data lake solutions depends on security requirements, budget constraints, and scalability needs. Businesses with stable, long-term storage demands may find an on-premise approach cost-effective, while organizations seeking agility and reduced infrastructure management often benefit from cloud-based solutions. Hybrid approaches, combining on-premise control with cloud scalability, provide an alternative for businesses needing security and flexibility.
Implementing data lake solutions with ROI
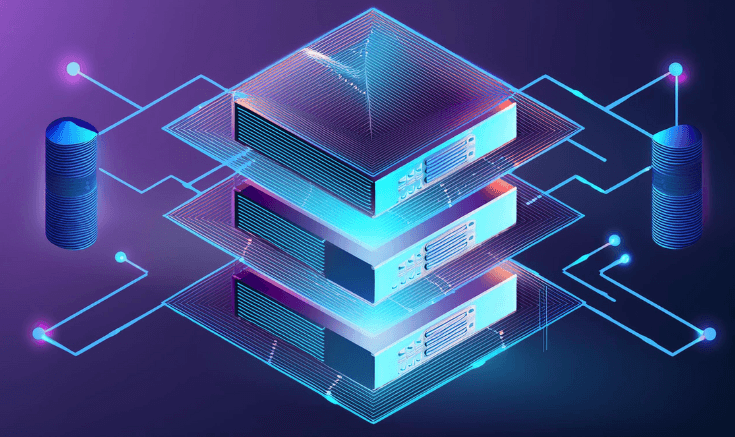
Investing in a data lake solution provides long-term value when implemented with a clear strategy. Simply storing large volumes of data does not guarantee meaningful insights or operational benefits. Data lake solutions enhance analytics capabilities, strengthen governance, and improve cost efficiency. Maximizing ROI requires aligning the technology with business objectives, optimizing storage strategies, and ensuring that data remains accessible and actionable for analytics and machine learning models. Several key factors influence the success of a data lake solution. Without a structured approach, excessive storage costs, inefficient query performance, and poor governance can limit the value of the investment.
- Define measurable business objectives: Establishing clear goals for data usage improves the ability to extract value from stored information. Typical objectives include enhancing customer insights, optimizing operational workflows, and streamlining regulatory compliance. Aligning storage and analytics capabilities with these goals improves short-term efficiency and long-term data usability.
- Adopt a cost-efficient storage strategy: Storing high volumes of data without a structured plan leads to unnecessary expenses. A tiered storage model categorizes data based on access frequency, assigning frequently used information to high-performance storage while archiving less critical datasets in lower-cost options. This approach optimizes storage costs without compromising accessibility.
- Enhance analytics with machine learning integration: A data lake solution becomes more valuable when integrated with machine learning platforms. Predictive analytics and automated data processing improve operational efficiency, allowing businesses to detect patterns, forecast trends, and optimize evaluation strategies. Connecting structured and unstructured datasets with machine learning applications broadens analytical capabilities and strengthens positioning.
- Strengthen governance and security controls: Poor data governance can create compliance risks and limit usability. Role-based access controls, data encryption, and structured metadata management improve data integrity while supporting regulatory requirements. A strong governance framework increases trust in analytics outputs and prevents unauthorized access to sensitive information.
- Optimize query performance for scalability: Inefficient query execution increases processing costs and slows analytics workflows. Indexing strategies, partitioning techniques, and caching mechanisms reduce query latency while maintaining cost efficiency. Cloud-based and hybrid data lake solutions benefit from automated query optimization tools that allocate resources dynamically based on workload demand.
- Automate ingestion and processing workflows: Standardizing data ingestion and transformation minimizes manual intervention and reduces processing errors. Automated pipelines improve the accuracy and efficiency of data collection, accelerating access to structured information for analytics teams. Metadata tagging and cataloging enhance discoverability, improving usability across departments.
- Monitor data lake performance and adjust strategies: Regular evaluation of storage utilization, processing efficiency, and analytics impact helps refine implementation strategies. Cost monitoring tools provide insights into usage patterns, allowing organizations to adjust retention policies, scale resources, and optimize query execution. Continuous improvement ensures the data lake solution delivers measurable business impact over time.
"Maximizing ROI requires aligning the technology with business objectives, optimizing storage strategies, and ensuring that data remains accessible and actionable for analytics and machine learning models."
Data lake implementation improves operational efficiency, strengthens governance, and enhances the ability to generate meaningful insights. A well-executed solution minimizes costs, accelerates analytics, and supports scalable data strategies, making stored information a valuable asset rather than a passive repository.
A data lake solution is more than just storage—it is the foundation for efficient, scalable, and insightful data management. With the right strategy, organizations can improve analytics, optimize costs, and strengthen security while ensuring compliance with industry standards. At Lumenalta, we specialize in building tailored data solutions that align with your business needs, providing the flexibility and governance required for long-term success. Let’s create a brighter path forward.
Table of contents
- What are data lake solutions
- 7 data lake solutions in 2025
- 1. Amazon S3-based data lake solution
- 2. Google Cloud Storage for data lakes
- 3. Snowflake Data Cloud
- 4. Databricks Lakehouse Platform
- 5. Cloudera Data Platform
- 6. Oracle Cloud Infrastructure Data Lake
- 7. Teradata VantageCloud Lake
- On-premise vs cloud data lake solutions
- Implementing data lake solutions with ROI
- Common questions about data lake solutions
Common questions about data lake solutions
What is a data lake solution, and how does it work?
What are the benefits of cloud data lake solutions over on-premise solutions?
How can a data lake solution improve analytics and business intelligence?
What industries benefit the most from data lake solutions?
How can businesses control costs when implementing a data lake solution?
Want to learn how data lake can bring more transparency and trust to your operations?