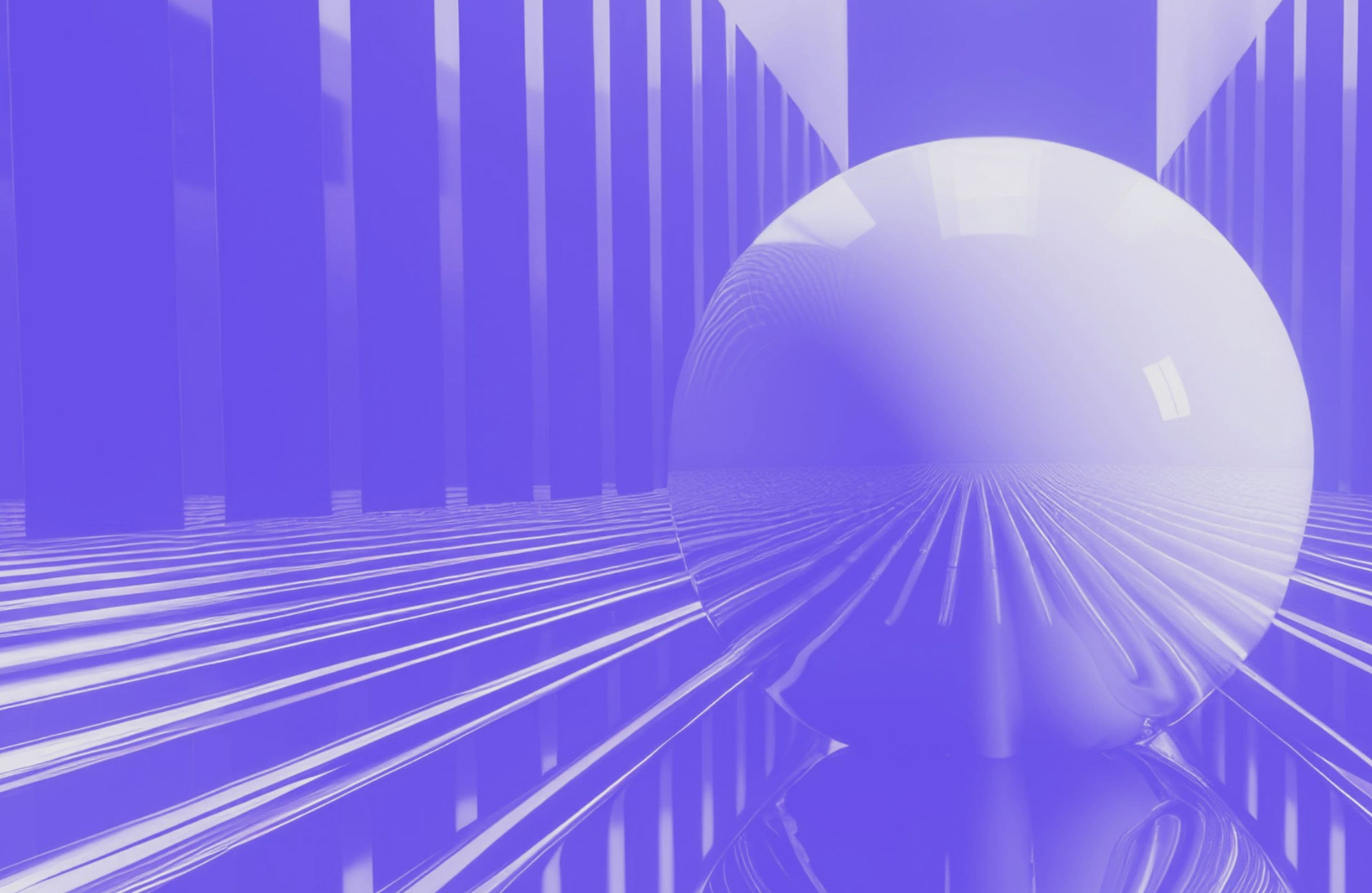
5 AI adoption challenges in data governance
NOV. 7, 2024
2 Min Read
Despite AI’s promise in data governance, businesses face five critical hurdles–but the payoff is worth the climb.
From manufacturing to marketing, AI has infiltrated almost every corner of business, and it’s easy to get swept up in the hype. Triumphs may dominate the headlines, but the reality on the ground is often a different story. AI adoption isn't as easy as it looks.
In our recent survey, we asked business leaders to share their AI adoption experiences, and the results were eye-opening. While some organizations have successfully integrated AI into their data governance strategies, the majority still face serious hurdles.
Less than a third of respondents felt their companies were “very successful” in deploying AI/ML, and more than 80% highlighted the same top five pain points:
Less than a third of respondents felt their companies were “very successful” in deploying AI/ML, and more than 80% highlighted the same top five pain points:
- Data quality and integrity
- Complexity and expertise
- Integration with existing systems
- Security and privacy
- Cost
Sound familiar? Let’s dive into these challenges and explore how to conquer them.
1. Data quality and integrity
“Garbage in, garbage out.” This timeless adage couldn’t be more true when it comes to AI. In fact, nearly all (98%) of the business leaders we surveyed cited data quality and integrity as a major hurdle in their AI adoption journey.
Data is the foundation of your AI initiatives. If it’s unreliable, inaccurate, or incomplete, your AI models will amplify those flaws, leading to poor outcomes across the board.
So, what are the characteristics of high-quality data?
Data is the foundation of your AI initiatives. If it’s unreliable, inaccurate, or incomplete, your AI models will amplify those flaws, leading to poor outcomes across the board.
So, what are the characteristics of high-quality data?
- Accuracy: Data must be precise and accurately reflect the real-world information it represents. Errors and inconsistencies can easily mislead your AI models.
- Completeness: Missing data is like a missing puzzle piece—without it, AI models can’t form a clear picture. Ensure your data is thorough and includes all necessary elements for accurate analysis.
- Consistency: Your data should look and feel the same across all systems. Variations in formats, naming conventions, or definitions can lead to confusion and faulty results.
- Timeliness: Stale information can lead to misguided decisions and missed opportunities. Refresh your data regularly so that it reflects the current state of your business.
2. Complexity and expertise
While AI offers tremendous potential, it’s far from a plug-and-play solution. According to our survey, complexity and limited expertise were major barriers to AI adoption for 97% of respondents.
Effective AI-driven data governance demands a coordinated approach. Business leaders, IT teams, and data teams need to be on the same page, aligning on goals, frameworks, and the tools required for successful implementation.
As new concepts like explainability, bias mitigation, and model robustness emerge, they bring additional layers of sophistication that require specialized knowledge and skills.
To get their arms around this complexity, organizations need to invest in both internal talent development and strategic external partnerships. Upskilling your data teams through training and mentorship programs can empower them to fully embrace AI and contribute effectively to your data strategy.
Additionally, adopting AI governance tools that automate tasks like data documentation and insight generation can ease the technical burden, allowing internal teams to focus on higher-level initiatives.
3. Integration with existing systems
Merging AI with your existing technology infrastructure comes with a host of complexities. 93% of the business leaders we surveyed admitted that integrating AI with their existing systems was a major AI adoption roadblock.
Many mid-market organizations operate with a patchwork of legacy platforms, each with its own data structures, definitions, and documentation. Because AI demands a seamless flow of information between systems, this lack of standardization can create major headaches.
Many mid-market organizations operate with a patchwork of legacy platforms, each with its own data structures, definitions, and documentation. Because AI demands a seamless flow of information between systems, this lack of standardization can create major headaches.
Whether it's connecting AI models to existing data warehouses or ensuring smooth data transfers between old and new platforms, integration hiccups can lead to bottlenecks, inefficiencies, and a whole lot of frustration for all involved.
Successful AI integration requires bridging the gap between your new AI tools and your existing technology ecosystem. Doing so calls for a strategic approach that addresses several factors:
Compatibility
First and foremost, make sure your AI solutions can play nice with your existing hardware and software. This might involve upgrading outdated systems, adopting new data formats, or investing in middleware that can act as a translator between different platforms.
Data integration
AI thrives on data, but that data is often scattered across different systems within an organization. Integrating these disparate data sources is crucial. Building data pipelines, implementing data lakes, and leveraging cloud-based data integration services are all great ways to bring all your data under the same roof.
Process alignment
Aligning your AI implementation with your existing workflows and processes is vital for ensuring a smooth transition and maximizing adoption. This might involve re-engineering existing processes, developing new workflows, or re-training employees to work effectively with AI-powered tools.
Scalability
As your AI initiatives grow and evolve, your technology infrastructure needs to keep pace. Ensure that your systems can handle the increasing demands of AI workloads by investing in cloud-based scalability or on-premises infrastructure upgrades.
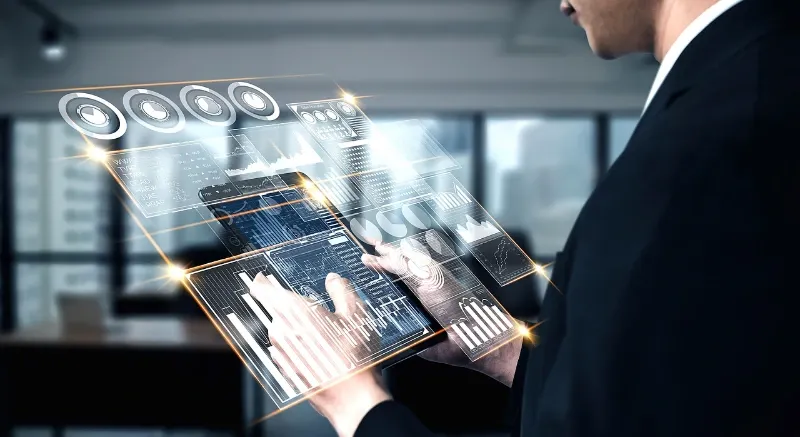
4. Security and privacy
For all its promise, AI also introduces risks related to privacy and security, particularly when handling sensitive data. In our survey, 86% of respondents expressed these concerns.
The core of these risks involves the potential for unauthorized access, data breaches, and exposure of personally identifiable information (PII). Given that AI models often rely on large datasets, they may inadvertently process sensitive information, posing compliance challenges under regulations like GDPR and CCPA.
The core of these risks involves the potential for unauthorized access, data breaches, and exposure of personally identifiable information (PII). Given that AI models often rely on large datasets, they may inadvertently process sensitive information, posing compliance challenges under regulations like GDPR and CCPA.
However, these risks can be managed effectively with AI-driven governance tools. Machine learning algorithms can continuously monitor data access, identify vulnerabilities, and protect sensitive information through methods like data masking.
A robust security strategy should also include encryption, access controls, and regular audits to create a fortress around your data.
5. Cost
AI promises long-term cost savings through improved efficiency, but the upfront investment can be a significant barrier for many mid-market companies. The overwhelming majority (85%) of survey respondents reported that cost was a major factor holding them back from broader AI adoption.
The high cost of AI stems from various factors. Beyond the initial investment in AI infrastructure—such as data storage, processing power, and specialized tools—there are ongoing expenses related to maintenance, talent acquisition, and system upgrades.
Furthermore, the cost-saving benefits of AI, like process automation or reduced manual intervention, may take time to materialize, putting pressure on short-term budgets.
But don’t let these challenges scare you off—the long-term ROI of AI is often well worth it. Our whitepaper revealed organizations that successfully implemented AI in data governance saw measurable improvements in risk mitigation, operational efficiency, and customer satisfaction.
The high cost of AI stems from various factors. Beyond the initial investment in AI infrastructure—such as data storage, processing power, and specialized tools—there are ongoing expenses related to maintenance, talent acquisition, and system upgrades.
Furthermore, the cost-saving benefits of AI, like process automation or reduced manual intervention, may take time to materialize, putting pressure on short-term budgets.
But don’t let these challenges scare you off—the long-term ROI of AI is often well worth it. Our whitepaper revealed organizations that successfully implemented AI in data governance saw measurable improvements in risk mitigation, operational efficiency, and customer satisfaction.
A cost-effective AI strategy often starts with a phased approach. Focus on pilot programs that deliver early wins and prioritize tools that offer immediate efficiency gains to pave the way for broader adoption.
The AI advantage: Why the journey is worth it
The path to AI-powered data governance has its fair share of roadblocks, but they’re not insurmountable. Those who stay the course end up building a resilient data architecture that sets the stage for better decision-making and sustainable growth.
Our whitepaper showcases how organizations that embraced AI in data governance saw significant improvements in decision-making, scalability, and compliance. So, don’t let the obstacles deter you. Start small, focus on quick wins, and watch AI transform your approach to data governance.
Curious to learn more? Download our whitepaper for a deeper dive into conquering these challenges and maximizing your AI ROI.
Curious to learn more? Download our whitepaper for a deeper dive into conquering these challenges and maximizing your AI ROI.
Ready to unlock the benefits of artificial intelligence in your organization?