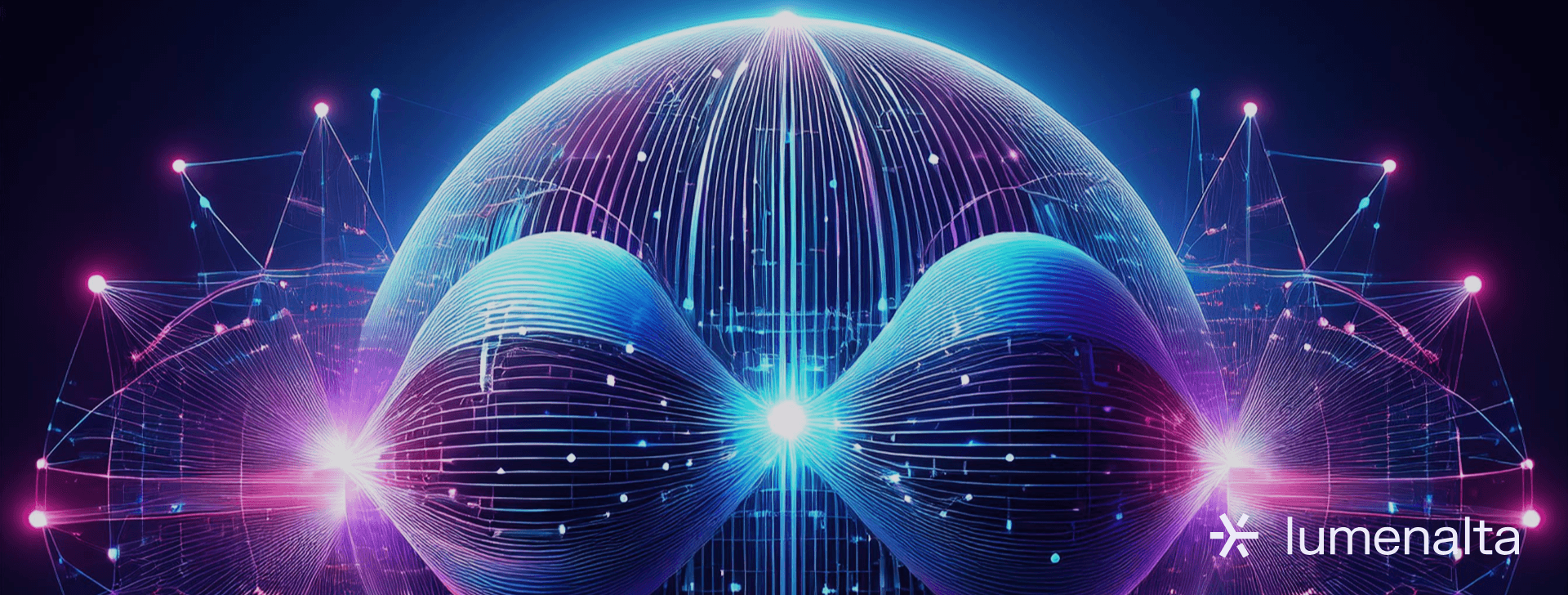
10 AI use cases in private equity
OCT. 29, 2024
8 Min Read
Private equity companies are increasingly adopting artificial intelligence (AI) to enhance their strategies and drive superior outcomes.
From predicting market trends to identifying new deal opportunities, AI tools are reshaping the way organizations operate. This article explores key AI use cases in private equity, providing insight into how companies can leverage AI to stay competitive while managing risks efficiently.
The adoption of AI is transforming how private equity entities operate across multiple phases and use cases:
Key takeaways
- 1. AI-driven predictive analytics allows private equity firms to forecast asset performance, optimize strategies, and improve returns with precision.
- 2. Risk management tools powered by AI help firms identify financial distress early and monitor market volatility in real time.
- 3. Deal-sourcing processes are enhanced by AI, uncovering hidden opportunities and high-growth companies through big data analysis.
- 4. AI facilitates real-time monitoring and automation in portfolio management, driving operational efficiency and sustainable growth.
- 5. Adopting AI successfully requires private equity firms to ensure robust data management, skilled talent, and strategic workflow integration.
1. Predictive analytics
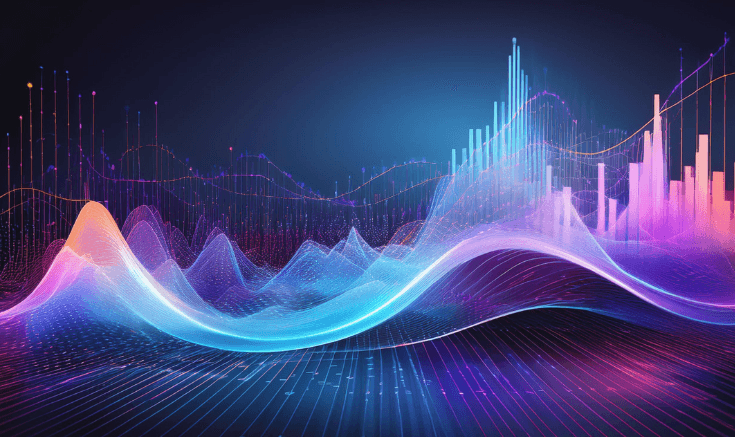
Predictive analytics is revolutionizing how private equity firms shape their investment strategies, giving them a competitive edge by anticipating future trends and returns. AI-driven models process vast amounts of historical and market data, providing insights that guide investment decisions with precision.
Applications of predictive analytics in private equity
- Forecast asset performance with precision: AI models leverage historical performance data, industry benchmarks, and economic factors to predict the future performance of various assets. Private equity companies can now make more accurate projections regarding investment returns, reducing reliance on intuition and market speculation.
- Identify macroeconomic trends proactively: Predictive algorithms monitor key economic indicators—such as GDP, inflation, and interest rates—alongside real-time financial news and industry reports. This continuous stream of insights allows organizations to anticipate economic cycles, market downturns, and sector-specific trends that could impact their portfolio.
- Optimize entry and exit strategies: The ability to time investments is critical to private equity success. AI-powered predictive models analyze market patterns, recommending the ideal times to buy or exit investments. Companies can avoid losses and maximize profits by acting on precise forecasts of market conditions.
- Scenario planning for uncertainty management: Predictive analytics tools enable organizations to model various market scenarios and their potential impact on portfolios. This helps private equity teams assess risks and benefits under different conditions, improving decision-making during uncertain economic climates.
- AI in retail and healthcare predictions: AI plays a crucial role in identifying emerging trends within retail and healthcare—two sectors highlighted by Lumenalta’s survey as most likely to be disrupted by AI. By leveraging predictive analytics, private equity firms can forecast shifts in consumer behavior, enabling them to position portfolio companies for growth or exit opportunities before competitors act.
By integrating predictive analytics into investment strategies, private equity organizations gain better visibility into future outcomes, aligning decisions with long-term ROI objectives and minimizing uncertainty.
"Predictive algorithms monitor key economic indicators—such as GDP, inflation, and interest rates—alongside real-time financial news and industry reports."
2. Risk management
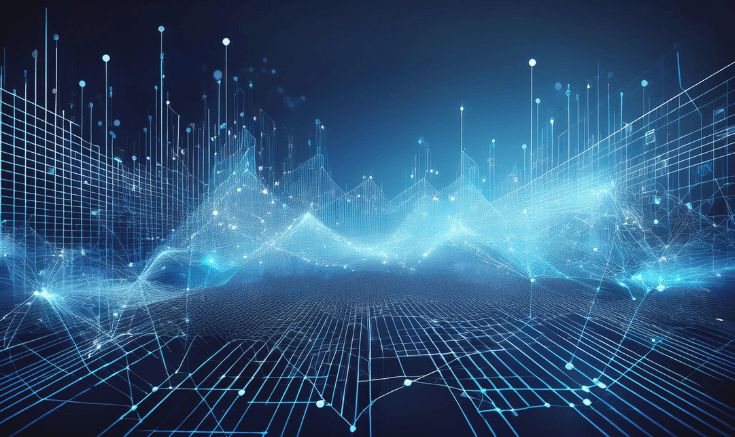
Risk management is a cornerstone of private equity, and AI is transforming how companies approach it. Traditional risk management methods often miss subtle patterns that could signal future problems, but AI tools can detect such patterns early and mitigate risks before they escalate.
Applications of AI in risk management
- Credit risk analysis through machine learning: AI models analyze a company's historical financial statements, cash flows, and industry data to identify early signs of financial distress or credit risk. This analysis provides a more nuanced view of a company's creditworthiness, helping organizations avoid high-risk investments.
- Monitoring market volatility in real time: AI tools provide real-time insights into market fluctuations, identifying sudden changes that could affect portfolio companies. Entities can act quickly on these insights, adjusting strategies to minimize exposure to volatile markets.
- Regulatory compliance alerts: AI algorithms track changes in regulatory frameworks, automatically flagging investments or companies that might be at risk of non-compliance. By staying ahead of regulatory updates, organizations can avoid costly penalties and reputational damage.
- Proactive cybersecurity assessments: Cybersecurity remains a top concern, with AI tools offering proactive assessments by identifying vulnerabilities across portfolio companies’ networks. Beyond tech challenges, human capital risks must also be managed. Lumenalta’s survey found that 46% of firms cite talent shortages as a significant obstacle to AI adoption. Integrating AI-driven risk management tools helps mitigate operational risks while identifying the talent needed to leverage AI effectively.
AI-driven risk management offers private equity organizations the ability to predict, monitor, and mitigate risks more effectively, ensuring that their investments remain protected and aligned with their strategic goals.
3. Deal sourcing
Deal sourcing is fundamental to private equity success, and AI tools are streamlining this process by uncovering opportunities that traditional methods might miss. With the ability to process massive datasets quickly, AI accelerates deal sourcing and expands the range of opportunities available to companies.
How AI supports better deal sourcing
- Identifying high-growth companies through pattern recognitionAI analyzes market data, financial performance indicators, and consumer sentiment to pinpoint companies with high growth potential. This helps private equity organizations focus on emerging leaders across various sectors.
- Discovering hidden opportunities with NLP toolsNatural language processing (NLP) algorithms sift through unstructured data, including news articles, blogs, press releases, and social media posts, to identify companies or sectors gaining momentum. These insights reveal opportunities that might be overlooked by competitors.
- Optimizing outreach strategies for better engagementAI tools analyze past deal interactions and outcomes to recommend personalized messaging strategies for potential targets. This increases the likelihood of a successful approach, improving engagement and deal closure rates.
- Efficient screening of potential investmentsAutomated screening tools process thousands of potential investments rapidly, prioritizing high-value targets based on predefined criteria. This allows firms to focus their efforts on the most promising opportunities, saving time and resources.
AI-driven deal sourcing ensures that private equity entities maintain a healthy pipeline of opportunities, giving them the insights needed to move swiftly on high-potential investments.
"AI models analyze a company's historical financial statements, cash flows, and industry data to identify early signs of financial distress or credit risk."
4. Portfolio management
Effective portfolio management requires continuous monitoring, operational optimization, and strategic decision-making. AI tools enhance these processes by providing real-time insights, automating workflows, and identifying new growth avenues within portfolio companies.
AI’s impact on portfolio management
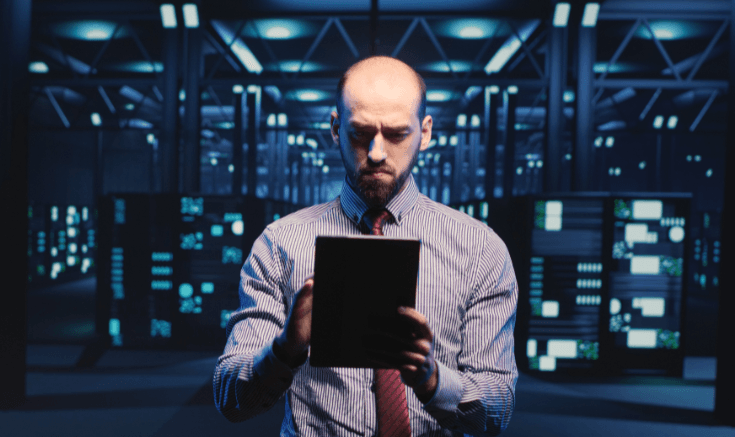
- Monitoring key performance indicators (KPIs) with precision: AI-powered dashboards offer real-time visibility into the performance metrics of portfolio companies, enabling private equity teams to identify issues early and act quickly to resolve them.
- Enhancing operational efficiency through automation: AI automates routine tasks such as financial reporting and supply chain management, reducing operational costs across portfolio companies. This allows companies to focus more resources on strategic growth initiatives.
- Supporting revenue growth with actionable insights: By analyzing customer behavior, market trends, and product performance, AI tools provide recommendations for new revenue streams and customer acquisition strategies, helping companies grow sustainably.
- Improving governance and regulatory compliance: Automated compliance tools ensure that portfolio companies adhere to regulatory standards across markets. This reduces the burden on management teams, allowing them to focus on strategic objectives.
- Real-time ESG tracking: With increasing investor focus on sustainability, AI tools now integrate environmental, social, and governance (ESG) metrics directly into portfolio management platforms. These tools provide real-time updates on portfolio companies' ESG performance, helping private equity firms ensure compliance with regulatory standards while meeting investor demands for sustainable investments.
AI-powered portfolio management transforms how entities oversee and optimize their investments, driving value creation across every stage of the investment lifecycle.
5. Investment screening and analysis
AI technology empowers private equity companies to sift through vast amounts of structured and unstructured data during the screening process. Traditional manual methods are limited by time and human capacity, but AI automates this task with precision.
- Predictive analytics: AI-powered tools forecast company performance based on historical financials, market trends, and macroeconomic indicators. This allows organizations to focus on high-potential companies early in the process.
- Natural language processing (NLP): NLP algorithms analyze text-based data such as earnings call transcripts, annual reports, and news articles to extract sentiment and detect signals of growth or distress.
- Automated scoring models: AI systems score companies based on custom-defined investment criteria, prioritizing opportunities aligned with the firm’s strategy, such as sustainable growth, undervaluation, or market leadership.
- Faster pipeline management: AI tools assist firms in optimizing deal flow, rapidly filtering and organizing opportunities for in-depth analysis, saving weeks of effort.
AI-supported screening ensures that investment teams allocate their time to opportunities with the highest return potential while eliminating human error from early-stage assessments.
6. Due diligence
AI's role in accelerating due diligence extends beyond financial analysis. Companies can now automate the review of regulatory filings, IP documentation, and news mentions to flag potential risks early. Our findings suggest that organizations leveraging AI for due diligence achieve faster deal cycles, helping them capitalize on time-sensitive opportunities.
- Financial audits and anomaly detection: AI algorithms review financial statements for discrepancies, patterns of fraud, or operational inefficiencies that might be overlooked manually.
- Legal and regulatory review: Machine learning models automate the evaluation of legal contracts, compliance reports, and regulatory filings, flagging areas of concern such as lawsuits, IP issues, or compliance breaches.
- Reputational analysis: AI monitors news, social media, and other online platforms to assess the public perception of target companies, helping firms identify reputational risks before acquisition.
- Operational metrics review: AI tools evaluate key operational metrics such as workforce efficiency, supply chain robustness, and customer churn rates to provide a complete picture of the target company's strengths and weaknesses.
AI-driven due diligence increases accuracy and speeds up the review process, allowing private equity entities to complete deals faster and with more confidence.
7. Exit strategies
AI is transforming exit planning by identifying the most favorable conditions and partners for portfolio exits. Traditionally, firms relied on market intuition and industry knowledge, but AI now provides real-time data-driven insights.
- Optimal exit timing: Predictive analytics assess market trends, economic indicators, and industry performance to suggest the best timing for a profitable exit.
- Valuation analysis: AI models analyze comparable deals, industry benchmarks, and financial forecasts to establish the optimal valuation for portfolio companies.
- Buyer and partner identification: AI algorithms identify potential buyers or partners by matching industry trends, buyer preferences, and market signals with the firm’s portfolio companies.
- Simulated exit scenarios: AI tools create scenario models to predict various exit outcomes, helping entities evaluate the impact of different market conditions on returns.
This strategic approach minimizes uncertainty, maximizes returns, and ensures the smooth transfer of portfolio companies to new ownership.
8. Investment research
In the research phase, AI enables firms to stay ahead of market developments, uncover new trends, and identify disruptive technologies.
- Trend analysis and market insights: AI tracks macroeconomic trends, geopolitical risks, and industry developments in real time, identifying sectors with high growth potential.
- Advanced data aggregation: AI tools compile data from multiple sources, including financial reports, patents, and market research, to present a holistic view of investment opportunities.
- Sentiment analysis: NLP algorithms analyze social media, news, and public forums to gauge market sentiment toward companies and industries.
- Alternative data sources: AI taps into unconventional data such as satellite imagery, weather patterns, or online reviews, offering unique insights into market conditions and operational performance.
AI-powered research ensures firms can make well-informed, forward-looking investment decisions with precision and speed.
9. Portfolio company reporting
AI transforms reporting by providing real-time dashboards, automation, and actionable insights. This enables private equity organizations to monitor the performance of their portfolio companies closely and adjust strategies when necessary.
- Automated reporting and variance analysis: AI automatically generates financial and operational reports, highlighting any deviations from projections or industry benchmarks.
- KPI monitoring: Companies can use AI tools to track KPIs like revenue growth, profitability, cash flow, and customer retention in real time.
- Predictive performance alerts: AI models can predict underperformance risks early, allowing organizations to intervene before issues escalate.
- Visualization tools: Interactive dashboards provide a clear view of individual and aggregate portfolio performance, improving decision-making and stakeholder communication.
These AI capabilities offer deeper transparency, faster reporting cycles, and data-backed insights, helping firms maintain a competitive edge.
10. Capital preservation
Capital preservation is essential for mitigating risks and protecting investments, especially in volatile markets. AI helps organizations proactively manage risks and preserve capital by monitoring market conditions and internal operations.
- Risk monitoring and early warning systems: AI continuously monitors portfolio performance, detecting signs of financial stress or operational risks.
- Compliance automation: Machine learning tools automate compliance checks, ensuring portfolio companies adhere to regulatory standards and minimizing exposure to fines or legal action.
- Stress testing and scenario analysis: AI-powered simulations model different economic conditions, helping firms identify potential risks and develop contingency plans.
- Fraud detection: AI algorithms analyze transactional data and behavioral patterns to detect fraudulent activities in portfolio companies.
By leveraging these AI capabilities, organizations can make data-driven decisions that reduce risk exposure and ensure the long-term sustainability of their investments.
Challenges and considerations
While AI offers immense benefits for private equity companies, implementing these technologies effectively requires careful planning and strategic alignment. Below are the core challenges that organizations need to navigate to unlock AI's full potential.
Ensuring data quality and accessibility
AI systems thrive on high-quality, structured data. For accurate insights, companies must gather, cleanse, and organize vast datasets from multiple sources, such as financial statements, industry reports, and customer data. However, poor data quality can lead to unreliable models, skewing predictions and insights. Private equity entities need to invest in robust data management strategies, including automated data pipelines and governance frameworks, to ensure that data is accessible, clean, and relevant for AI models. Additionally, access to proprietary datasets or third-party data can give organizations a competitive advantage but may require strategic partnerships or investments in data acquisition tools.
Bridging talent and expertise gaps
AI tools require specialized knowledge in data science, machine learning, and predictive modeling—expertise that many private equity companies may not have in-house. Hiring and retaining AI specialists can be challenging, especially as demand for these professionals grows across industries. Organizations must decide whether to build internal AI teams or collaborate with external technology partners to implement and maintain AI solutions. Another consideration is training existing employees to work alongside AI systems, ensuring seamless integration of human and machine intelligence within investment and portfolio management teams.
Navigating regulatory and ethical considerations
AI tools must comply with increasingly complex regulatory frameworks, including data privacy laws, financial regulations, and industry-specific rules. Misalignment with these regulations can lead to fines, reputational damage, or even the loss of investor trust. Ethical considerations are equally important—AI models need to be transparent, fair, and free from bias to maintain credibility. Private equity organizations must implement governance frameworks to monitor AI systems, ensuring they adhere to ethical standards and regulatory requirements. Staying updated on evolving regulations and engaging in industry forums or working groups can also help companies stay ahead of compliance challenges.
Aligning AI tools with existing workflows
One of the biggest challenges organizations face is ensuring that AI solutions integrate seamlessly with existing operational workflows. Misaligned AI implementations can create inefficiencies, disrupt established processes, or lead to resistance from employees. Companies need to approach AI adoption incrementally, ensuring that tools complement and enhance current operations. Adopting a phased implementation strategy can help entities test AI solutions on smaller processes before scaling them across the organization. Change management initiatives, including employee training and communication strategies, are also crucial to foster a culture of AI adoption.
Balancing the cost of adoption with expected returns
The financial investment required to develop, implement, and maintain AI tools can be substantial. Organizations must carefully evaluate the costs against the potential benefits to ensure that AI adoption drives positive ROI.
According to our survey, 50% of private equity professionals identified system integration as the biggest hurdle to AI adoption. Firms must adopt a phased implementation strategy, testing AI solutions on smaller processes before scaling them organization-wide. Building partnerships with AI service providers can also bridge internal talent gaps, ensuring smoother implementation and faster results.
Beyond initial deployment, ongoing costs—such as software licensing, infrastructure upgrades, and continuous model optimization—must be factored into the equation. To maximize returns, private equity entities need to align AI initiatives with their strategic objectives, focusing on areas where AI can deliver the most significant impact, such as deal sourcing or portfolio management. Regular performance evaluations will help ensure that AI investments continue to provide value over time.
By addressing these challenges, private equity organizations can build a robust foundation for AI adoption, enabling them to extract value across every stage of the investment process. A thoughtful approach to implementation ensures that AI becomes a strategic enabler, driving long-term success.
"AI-powered tools assess the security posture of portfolio companies by scanning their networks for vulnerabilities."
The evolving role of AI in private equity
AI's role in private equity is poised to evolve as new technologies emerge and more advanced applications become accessible. As companies explore the potential of AI, several developments are expected to shape the future of private equity operations.
AI-powered fundraising platforms
AI-driven platforms are beginning to transform how private equity organizations engage with investors, streamlining the fundraising process. These tools use advanced algorithms to match entities with potential investors based on shared goals, risk appetite, and industry focus. By automating investor outreach and engagement, AI platforms reduce the time and effort needed to secure funding while increasing the likelihood of building long-term partnerships.
Real-time investment performance tracking
Companies are increasingly adopting AI-powered dashboards that provide up-to-the-minute insights into portfolio performance. These systems aggregate data from multiple sources, enabling real-time monitoring of key financial metrics. Private equity teams can quickly identify underperforming assets, adjust strategies as needed, and capitalize on emerging opportunities. With continuous performance tracking, organizations are better equipped to respond proactively to market changes.
AI-driven ESG analysis
As environmental, social, and governance (ESG) considerations gain prominence, AI tools are becoming essential for assessing companies' sustainability metrics. Algorithms analyze vast datasets related to environmental impact, social responsibility, and governance practices, providing organizations with objective insights into potential investments. AI-powered ESG analysis allows private equity entities to align their portfolios with socially responsible investing principles, meeting the growing demand from investors for sustainable business practices.
Predictive financial modeling tools
Predictive financial models are becoming more sophisticated, allowing private equity companies to simulate future economic scenarios with greater accuracy. These tools account for various market factors—such as interest rates, inflation, and geopolitical events—helping organizations anticipate how their investments will perform under different conditions. By leveraging predictive modeling, firms can refine their investment strategies and position themselves for long-term success, even in volatile markets.
The future of private equity hinges on talent as much as technology. The survey found that 66% of firms prioritize hiring executives with AI expertise to drive digital transformation strategies. By creating new AI-focused roles, private equity companies are positioning themselves to lead in an increasingly data-driven industry.
AI offers private equity organizations the opportunity to optimize every aspect of their operations, from deal sourcing to risk management and portfolio enhancement. By embracing these technologies, firms can unlock new levels of efficiency and insight. If you're ready to explore how AI-driven solutions can transform your yprivate equit strategy, Lumenalta's experts are here to help.
table-of-contents
- 1. Predictive analytics
- 2. Risk management
- 3. Deal sourcing
- 4. Portfolio management
- 5. Investment screening and analysis
- 6. Due diligence
- 7. Exit strategies
- 8. Investment research
- 9. Portfolio company reporting
- 10. Capital preservation
- Challenges and considerations
- The evolving role of AI in private equity
- Common questions about AI use cases in private equity
Common questions about AI use cases in private equity
How is AI transforming private equity strategies?
What role does predictive analytics play in private equity?
How does AI improve risk management in private equity investments?
What AI tools are essential for effective portfolio management?
What challenges do private equity firms face in AI adoption?
Want to learn how artificial intelligence can bring more transparency and trust to your operations?