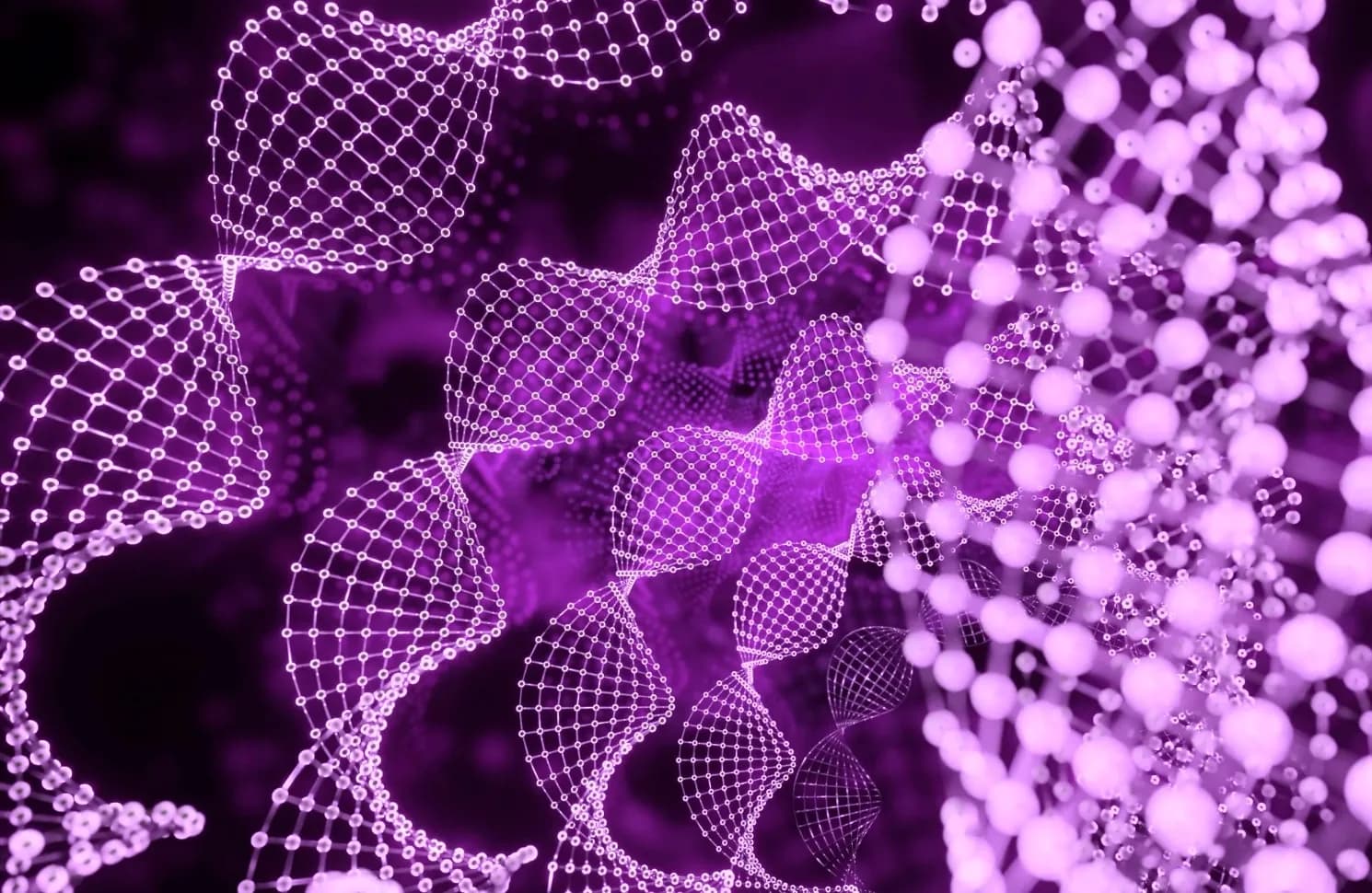
The forgotten potential: Deep learning and genetic algorithms
AUG. 26, 2024
2 Min Read
Here is a fresh perspective on genetic algorithms and deep learning so you can build tailored technology solutions for your organization.
In all the excitement around new AI tools, it’s easy to overlook that established techniques like genetic algorithms and pre-GPT models of deep learning have been contributing to AI progress for decades.
These approaches, both individually and in combination, have untapped potential, especially with recent advances in hardware, data availability, and algorithmic design.
Genetic algorithms, inspired by the principles of natural selection, have long been powerful tools for optimization and problem-solving across various industries.
Meanwhile, deep learning has revolutionized fields like computer vision, speech recognition, and natural language processing. The synergy between these two approaches offers exciting possibilities for innovation and problem-solving.
It’s time for a fresh perspective on these methods so you can build tailored technology solutions for your organization. By understanding the strengths and limitations of both genetic algorithms and deep learning, as well as their potential when used together, you can unlock new opportunities for technological advancement and business optimization.
The significance of deep learning and genetic algorithms
Deep learning and genetic algorithms have both played crucial roles in the development of AI, each with its own unique strengths and applications. Here’s a quick overview of their evolution:
- 1940s-1960s: Introduction of neural networks and the perceptron
- 1960s-1970s: Development of genetic algorithms by John Holland
- 1960s-1980s: Advancement of backpropagation and convolutional neural networks
- 1980s-1990s: Decline of interest in deep learning during the “AI winter”, but continued development of genetic algorithms.
- 2000s: Resurgence of deep learning driven by advanced computational power and large datasets, leading to performance improvements in various domains
- 2010s-present: Increased interest in combining deep learning and genetic algorithms for enhanced problem-solving capabilities
Both deep learning and genetic algorithms have evolved from academic curiosities to powerful tools for end-to-end digital solutions and tech transformation. Their combination offers even greater potential for solving complex problems and driving innovation across industries.
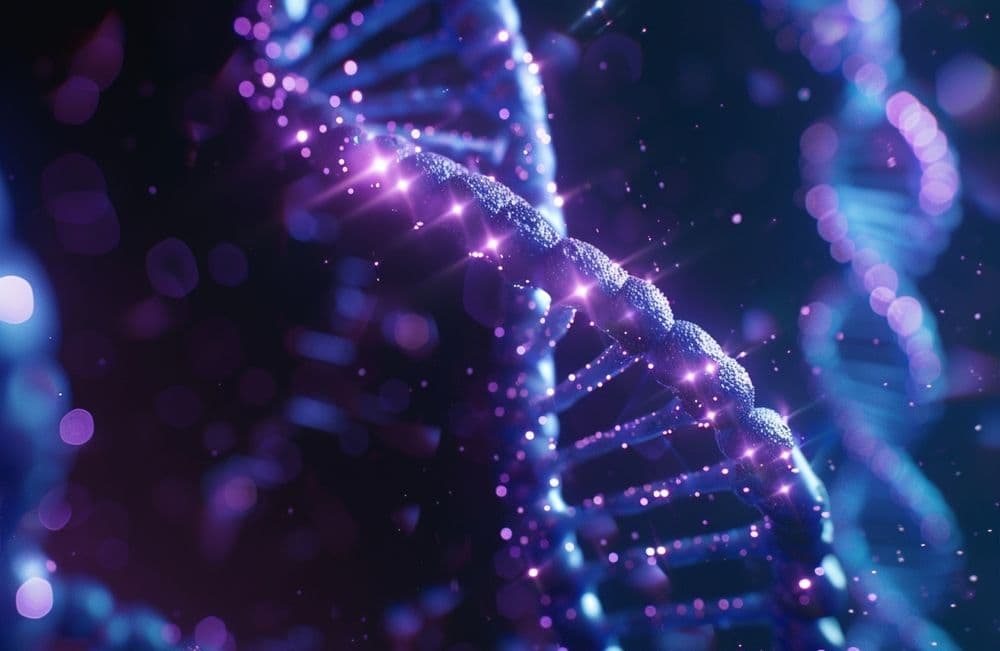
Where these applications are strong
Deep learning strengths
Deep learning excels at building models from large volumes of complex, unstructured data. It continuously learns and improves from new information, enabling it to quickly adapt to changing market conditions, emerging trends, and evolving customer needs.
Genetic algorithms strengths
Genetic algorithms, on the other hand, are particularly adept at optimization problems, finding efficient solutions in large, complex search spaces where traditional methods may struggle. They can handle multiple objectives and constraints simultaneously, making them ideal for real-world business problems.
When combined, these capabilities accelerate innovation, allow agile responses to business challenges, and make deep learning versatile across industries.
Consider the following examples:
Autonomous farming systems
Companies like John Deere use deep learning to analyze photos from robots or drones, detecting ripe produce, weeds, and diseases. Genetic algorithms can then optimize the routing and scheduling of farming equipment based on this data.
Manufacturing quality control
Factories use computer vision and deep learning algorithms to analyze images of products on the production line for defects and anomalies. Genetic algorithms can optimize the production process parameters to minimize these defects.
Art restoration
Researchers have applied deep neural networks to analyze textures, patterns, and styles of famous artists to reconstruct missing or faded parts of paintings. Genetic algorithms can be used to fine-tune the restoration process, exploring different combinations of brushstrokes and colors.
This list isn’t exhaustive. The combination of deep learning and genetic algorithms shows up everywhere, from B2C applications like content recommendations on streaming platforms to arcane B2B settings like predictive maintenance in industrial equipment.
This versatility offers opportunities to drive innovation across multiple business functions and tech transformations of entire value chains.
Limitations and challenges
Despite their strengths, genetic algorithms and deep learning have limitations to consider before you radically engage with it for your organization’s strategy.
Requires large amounts of training data (deep learning)
Finding high-quality, labeled data to train deep learning models is a significant challenge. While they can quickly adapt once trained, the initial process of finding high-quality, labeled data can be time-consuming and expensive, especially in domains with scarce or hard-to-obtain data, like healthcare, niche agricultural practices, and space exploration.
Computational intensity (both)
Training complex models and running genetic algorithms for large-scale problems requires powerful hardware, like GPUs and substantial memory resources. This equipment consumes energy and time, straining your organization’s infrastructure and sustainability goals.
You should account for these resource demands when planning IT infrastructure investments or consider cloud computing services to support deep learning initiatives.
Lack of interpretability (deep learning)
The complex, layered structure of deep neural networks makes it difficult to understand their decisions (also called “interpretability”). This “black box” nature hinders error identification and raises questions about the model's trustworthiness.
In highly regulated industries like finance or healthcare, the lack of interpretability can create compliance challenges, requiring additional oversight and risk management measures.
Solution quality and reliability concerns (genetic algorithms)
Genetic algorithms sometimes converge on suboptimal solutions if they’re not properly configured.
Your teams should have the skills and time to evaluate solutions produced by genetic algorithms before applying them to real-world business problems to mitigate this risk.
Expertise and resource intensity (both)
You need to weigh the costs of acquiring talent and computing resources against the benefits of these AI technologies. Assess if your organization can support this technology or if partnerships with specialized firms are more viable.
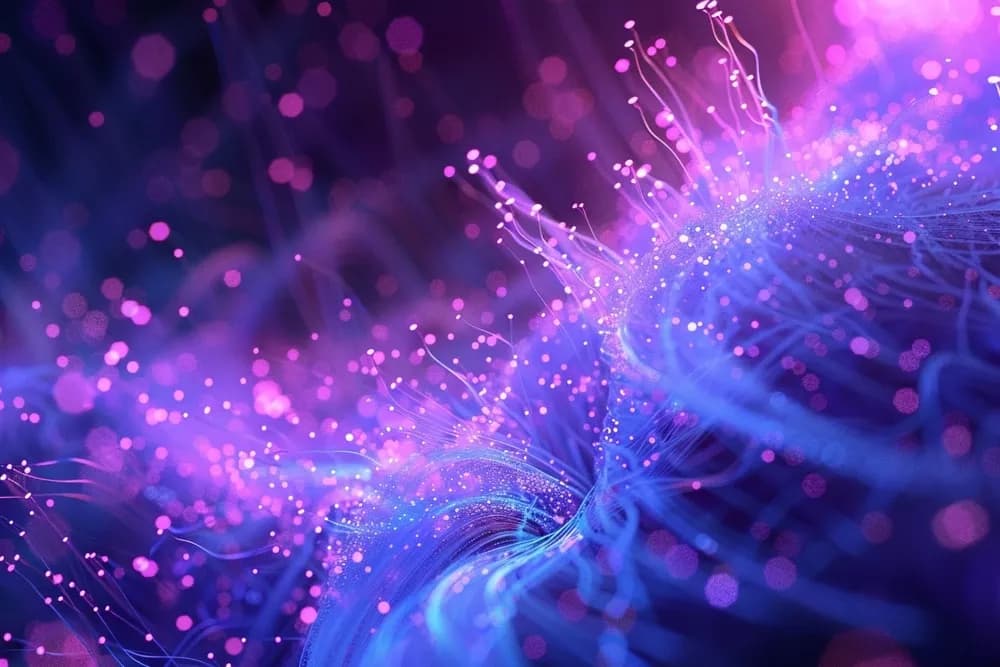
The power of combining deep learning and genetic algorithms
Despite these challenges, the combination of deep learning and genetic algorithms offers powerful solutions to complex problems. Here’s how they can complement each other:
Hyperparameter optimization: Genetic algorithms can be used to optimize the hyperparameters of deep learning models, potentially improving their performance and reducing the need for manual tuning.
Neural architecture search: Genetic algorithms can evolve the structure of neural networks, potentially discovering more efficient or effective architectures than those designed by humans.
Feature selection: Genetic algorithms can help identify the most relevant features for deep learning models, potentially improving their accuracy and reducing computational requirements.
Reinforcement learning: The combination of deep learning and genetic algorithms has shown promise in reinforcement learning tasks, where an agent learns to make decisions through trial and error.
The endurance of deep learning and genetic algorithms
The combination of these technologies with modern computational capabilities is especially promising. Cloud-based GPU solutions and distributed computing provide the power to train and run models at scale, even if your organization can’t afford or manage this infrastructure in-house. Therefore, organizations of all sizes can now radically engage with these technologies.
Advancements like transfer learning in deep learning and the ability of genetic algorithms to adjust their settings automatically make the tools more powerful, accessible, and affordable.
The synergy between these approaches allows for more robust and flexible AI solutions that can tackle a wider range of complex problems.
The deep learning — genetic algorithm combo in action
Combining deep learning with genetic algorithms can light the way to powerful and innovative tech builds in various fields.
Molecular behavior prediction in materials science
Neural networks can model complex molecular interactions, while genetic algorithms can optimize molecular structures. This synergistic approach is beneficial in chemistry and materials science, where understanding molecule and material behavior is crucial.
In manufacturing or R&D-heavy industries, this technology accelerates product development and reduces the costs of physical testing, resulting in faster time to market and better performance.
Optimized manufacturing workflows
Deep learning and genetic algorithms can collaborate to solve complex optimization problems. For example, they can tackle the Flexible Job Shop Scheduling Problem (FJSP), which involves scheduling multiple jobs with several operations across various machines.
Deep learning models can predict production times and quality outcomes based on historical data, while genetic algorithms can use these predictions to optimize scheduling. This combination enables businesses to solve FJSP challenges and improve production workflows more effectively than either technique alone.
You can often integrate these AI solutions with existing ERP and MES systems. This combination enables real-time production schedule optimization based on inventory, orders, and resource availability data.
Adaptive energy management and sustainability reporting
Genetic algorithms can evolve neural network architecture, making them more efficient and effective. For example, in smart grids:
1. Deep learning models can predict energy consumption patterns and renewable energy supply fluctuations.
2. Genetic algorithms can then optimize the distribution and storage of energy based on these predictions.
This combination allows businesses in the energy sector to achieve better load balancing, reduce waste, and improve the reliability of power distribution through continuous optimization of the network’s structure.
Deep learning and genetic algorithms can also support energy compliance and CSR initiatives:
- Emissions monitoring: Deep learning models can provide real-time monitoring and prediction of emissions, helping companies comply with regulations and reduce the risk of non-compliance fines.
- Sustainability reporting: AI systems can automate data collection and analysis for sustainability reports. This automation improves accuracy and reduces the time spent on reporting.
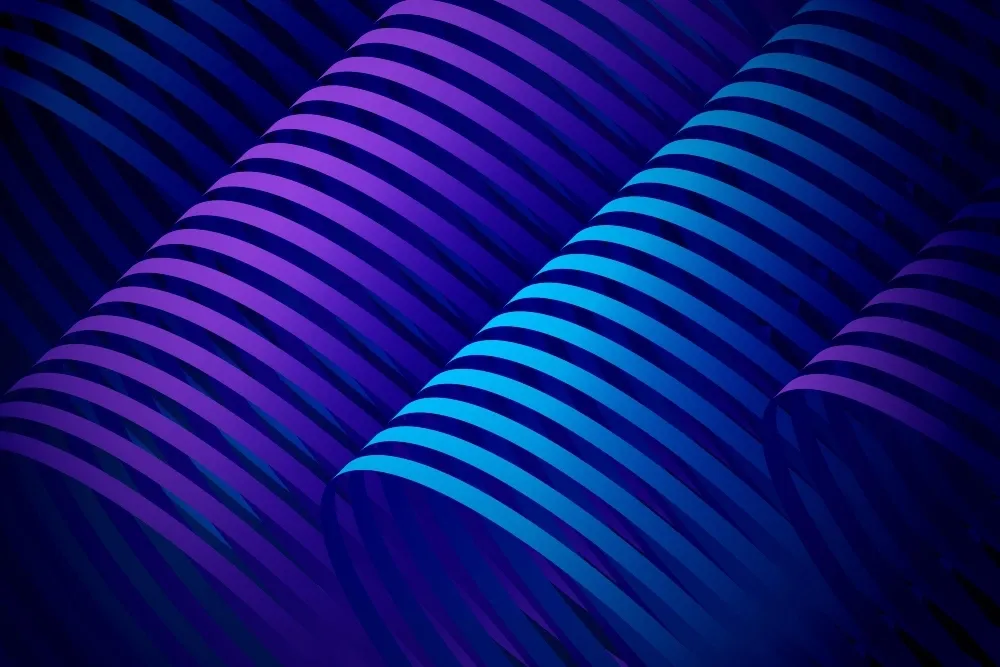
Always maintain a balanced approach to AI development
With all the possibilities of deep learning, genetic algorithms, and their combination, it’s tempting to see these technologies as a default solution for every problem. However, it’s crucial to remember that each tool has unique pros and cons for specific technical challenges and domain requirements.
AI’s evolving capabilities also unlock new possibilities when combined with other technologies like blockchain, the Internet of Things (IoT), and Big Data analytics.
The synergy between deep learning and genetic algorithms is just one example of how combining different AI approaches can lead to more powerful and flexible solutions.
Don’t lock in on a specific approach too quickly when considering what technologies to use to solve a business problem.
Build background on recent innovations first, then consider whether they can provide tailored technology solutions when combined with other tools — including long-established ones your competition might overlook.
Read next: The anatomy of a learning organization