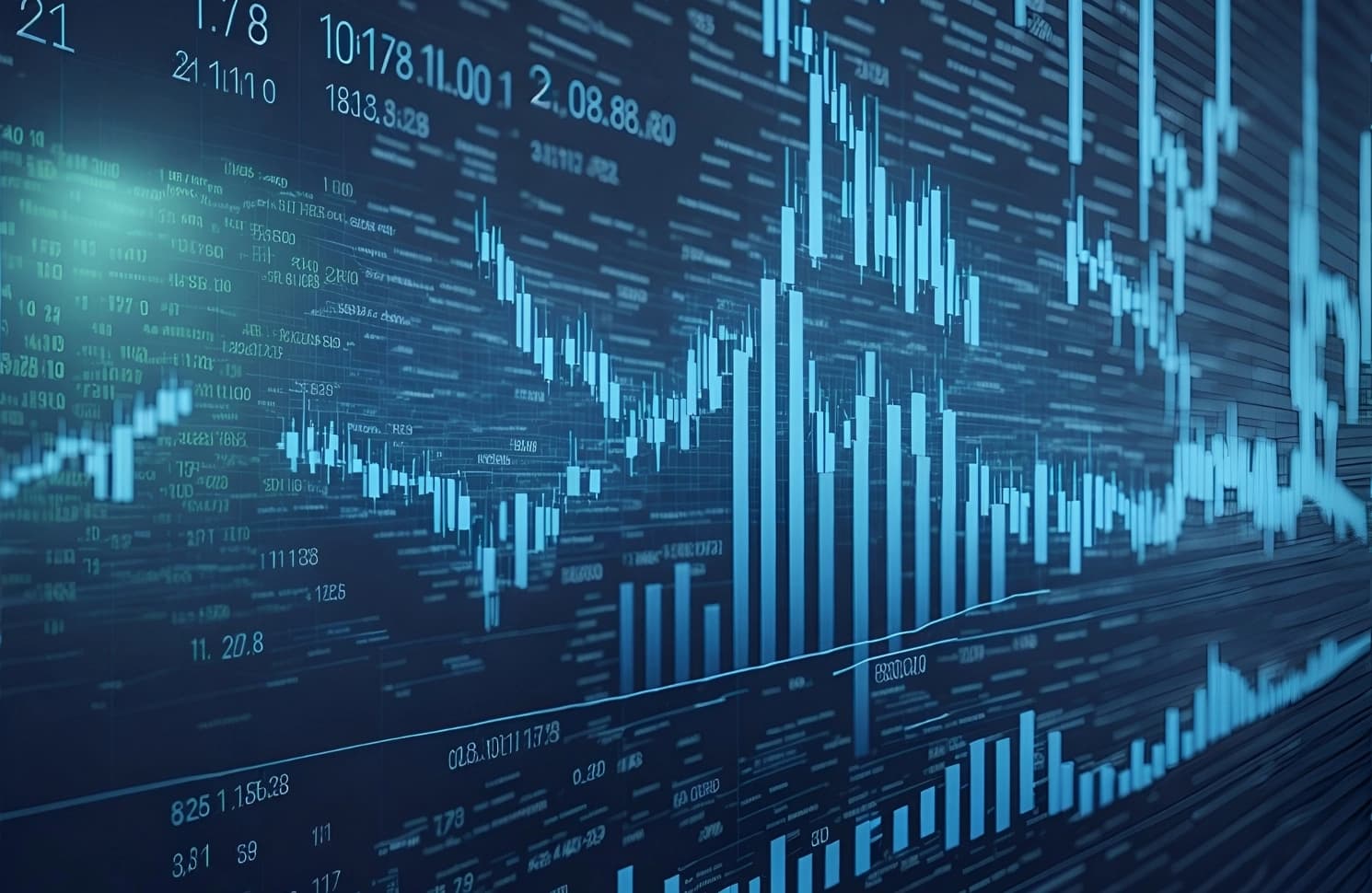
Supercharge your embedded finance initiatives with strategic AI
JUL. 10, 2024
2 Min Read
Blending embedded finance and AI enables hyper-personalization, process automation, and quick anomaly detection.
Embedded finance has changed dramatically in just a few years. Whereas non-financial services companies targeted consumers with financial services, a shift towards corporate-embedded finance is quickly gaining ground.
These rapid changes have been fueled by several factors, including:
- Increased adoption of non-financial companies offering financial services
- Personalization with AI and machine learning that tailor offerings to individual needs
- Technological advancements, such as cloud computing and APIs that have simplified the integration of financial services
- Expansion into new sectors such as healthcare, education, and manufacturing
- Integration with emerging technologies such as IoT devices and blockchain
During this evolution, non-financial services companies have recognized the impact of embedded finance on market share, revenue, and customer satisfaction, to name a few. If your company isn’t already offering embedded finance services, you’re falling behind.
But there’s good news: this sector is rampant in opportunities and shows no signs of slowing down. Recent research suggests embedded finance will reach $622.9 billion by 2032 with a 25.4% annual growth rate (CAGR). Boosting conversion, loyalty, and customer lifetime value by providing financial services at the point of sale is done by many companies, yet embedded finance is only half the story.
The real power comes from its crossover with AI.
By combining embedded finance with machine learning, natural language processing, and predictive analytics, companies can hyper-personalize offerings, automate complex processes, and detect anomalies immediately.
However, this combination brings new opportunities and risks, from data privacy and algorithmic bias to cybersecurity threats. But the upside is too large to ignore — the future of finance is embedded, intelligent, and happening now.
Personalize customer experiences and processes with AI
Spoiled by smooth buying journeys on Amazon and other e-commerce platforms, customers expect the same elsewhere. Embedded finance and AI help meet those expectations.
The front-end: Highly strategic personalized journeys powered by embedded finance
Embedded finance thrives within an ecosystem of partners across TradFi, neobanks, banking-as-a-service (BaaS) providers, Fintech, and consumer and commercial commerce. Furthermore, these capabilities can be augmented with first-party data to fuel insights and decision support to tailor customer experiences.
AI algorithms have the ability to predict individual preferences and risk profiles by analyzing customer data. You can use these insights to embed personalized offerings into your customer journeys.
Consider these examples:
Shopify Capital
Shopify Capital analyzes over 70 million data points on sales history and merchant performance to calculate optimal loan amounts and eligibility for sellers. Their AI models determine the right funding amount and terms for each seller. Using these capabilities, Shopify Capital has provided thousands of merchants with over $5 billion in funding.
Stripe
Stripe’s AI and machine learning-powered tools analyze real-time transaction data to optimize payment routing, enhance fraud detection, and make smarter credit decisions. The result is a more seamless, secure, and personalized checkout experience that can help businesses increase revenue.
Starbucks
Starbucks’s AI-powered mobile app uses various financial services, including a stored value account and a rewards program. They use AI to personalize offers and predict customer behavior, which indirectly affects their embedded finance offerings.
Amazon
Amazon uses AI extensively in its embedded finance offerings. For instance, Amazon Lending uses machine learning algorithms to determine which sellers on its platform are eligible for loans. Their AI also helps in detecting fraudulent transactions and in personalizing financial product offerings to customers.
Tesla
Tesla sells more than electric vehicles. To offer insurance to customers, they use AI to analyze driving data and adjust insurance premiums in real time based on driving behavior.
With the right technologies, non-financial companies across many sectors can deliver the right financial service at the right digital moment. Embedded finance enables them to offer a tailored loan to a small business or adjust credit limits on the spot based on data-driven insights.
They can also build resilience by managing revenues and answering customers’ needs within unpredictable markets. Lastly, it empowers them to innovate on a global scale and gain market share while keeping their brand in front of the buyer.
Read also: Do’s and Don’ts for navigating custom GPTs
The back-end: Streamlined financial processes
AI can also streamline and automate complex back-end processes. Technologies like computer vision, natural language processing, and predictive analytics can shrink financial processes from weeks to minutes.
Loan applications are a great example. The traditional approach involves stacks of paperwork, multiple touchpoints, and long decision cycles. Embedded lending uses AI to simplify the process by analyzing customer data to predict individual preferences and risk profiles.
Yaacov Martin, co-founder and CEO of The Jifiti Group, explains, “From an embedded lending point-of-view, one of the most significant benefits of AI is how it can help lenders and merchants personalize the customer loan journey, tailoring financing offers and recommendations based on a customer’s credit profile, purchase size and spending habits, in addition to assisting with real-time decisioning.”
Financial service providers can automate workflows like credit decisions, risk assessment, and fraud detection by analyzing customer data with machine learning models. These innovations reduce manual work and minimize errors.
Square uses ML models to analyze transaction data and provide financing offers. Deposited funds can be available the next business day, and Square calculates automatic repayments based on a fixed percentage of daily card sales. This entire process integrates into the seller experience with minimal friction.
Strengthen security and compliance with AI
Embedded finance providers are lucrative targets for fraudsters given their sensitive customer data — after all, cybercrime is projected to grow to $23.84 trillion by 2027. At the same time, banks, Fintechs (i.e., PayPal and Stripe), and consumer partners operate within an evolving patchwork of data privacy and financial regulations.
Failure on either front can erode customer trust, attract regulatory scrutiny, and result in penalties. AI helps build defenses and manage compliance complexity:
- Real-time risk assessment: AI monitors transactions and user behavior, identifying potential risks by detecting anomalies and suspicious patterns.
- Enhanced fraud detection & prevention: AI models can detect sophisticated fraud schemes that traditional rule-based systems might miss. They can also automate manual reviews to make detection more efficient and scalable.
- Improved compliance efficiency: AI can automate labor-intensive compliance tasks, reducing manual errors and freeing up resources for higher-value work.
Examples of strategic AI innovation in embedded finance
AI is already being used to strengthen security and compliance in embedded finance. For example.
Bank of America uses AI for fraud detection and customer alerts. Its AI system analyzes transactions, comparing them against historical data and known fraud patterns. If it detects suspicious activity, the system automatically triggers alerts for the customer and the bank’s fraud team. This setup has helped Bank of America significantly reduce fraud losses and improve its customer experience.
J.P. Morgan analyzes legal documents using natural language processing (NLP). Their COIN (Contract Intelligence) system extracts critical data points and clauses from complex contracts. This technology has reduced operational risk and time spent on manual document review from 360,000 hours to just seconds.
Taulia uses AI in corporate financing, including inventory management. Their platform leverages AI and machine learning to help businesses optimize their working capital and supply chain finance processes. Their AI-powered system analyzes spending patterns and supplier relationships, products cash flow needs, optimizes payment terms, and identifies opportunities for early payment discounts. This helps both buyers and suppliers manage their cash flow more effectively and reduces the need for traditional inventory financing in some cases.
Deploy AI strategically and responsibly
Embedding AI into financial offerings is complex and has significant implications for data privacy, fairness, transparency, and security.
As a strategic decision-maker, you play a critical role in ensuring responsible AI deployment to drive business value while mitigating risks. Here are some areas to focus on.
Develop robust data governance frameworks
Strong data governance is the foundation for accurate, unbiased, and compliant AI models. Following standards and industry regulations is essential. Additionally:
- Ensure data quality and consistency. Implement data validation checks, lineage tracking, and master data management to maintain data integrity.
- Protect sensitive financial data. Implement strict access controls, encryption, and monitoring to safeguard customer information.
- Mitigate algorithmic bias. Develop transparent guidelines and review processes to identify biases, and regularly audit your models using techniques such as disparate impact analysis.
Data management and data governance are critical to your AI implementation. You shouldn’t rush into AI until you’re confident of your data quality, accuracy, consistency, security, and compliance. The easiest thing about AI is AI, but maturing your data estate is less glamorous and often the most time-consuming and resource-intensive part of AI projects.
Implement MLOps into your AI lifecycle
MLOps (Machine Learning Operations) provides processes and tools to manage models from development and testing to deployment and monitoring. Here are some key aspects to consider:
- Apply DevOps principles to machine learning. Implement version control for code, data, and model versions. Establish reproducible pipelines for training, testing, and deployment.
- Automate model testing and validation. Integrate it into the CI/CD pipeline to ensure model quality and performance.
- Monitor models in production. Monitor for data drift, performance degradation, and anomalies. Set up automated alerts and response workflows to proactively identify and address issues.
- Enforce security and compliance throughout the lifecycle. Apply access controls, security scans, and compliance checks at each stage of the model lifecycle to maintain the highest standards.
- Set up LLMOps tools. ML can augment and improve existing processes and decision-making, particularly around compliance, operations for onboarding, regulatory reporting, fraud, and privacy. Make sure to leverage an evaluation framework in MLOps, along with observability to drive continuous improvement.
Collaborate and learn from industry peers
Deploying AI in embedded finance is complex and fast-evolving. Collaboration and shared learning are crucial because no organization has all the answers. Here's how to stay informed of responsible AI practices:
- Engage with industry consortia. Collaborate with industry consortia, regulators, academia, and cross-sector initiatives to define standards and best practices for responsible AI.
- Join relevant groups. Consider joining organizations like the Partnership on AI (PAI), the Fair Isaac Corporation (FICO), and the Institute of International Finance (IIF) to stay connected with the AI ethics community.
- Stay informed and benchmark. Attend conferences, join working groups, and participate in benchmarking studies to stay informed about emerging practices and assess your organization’s maturity.
Whether you’re a bank, Fintech, or non-financial company, joining this ecosystem of innovation and forming partnerships can translate into advantages that can further position you competitively in the market.
Embedded and AI-powered: finance’s present and future
Embedded finance use cases are expanding to include insurance, investments, cryptocurrency purchases, and loyalty programs. In addition, an increasing number of sectors embracing it further highlights its potential and benefits.
Now is the time for banks and other ecosystem partners that already haven’t to seize the opportunity and capture more market share or cement their position as comprehensive financial service providers with a robust embedded finance solution.
As a strategic planner, you’re in a unique position to shape this future. By strategically investing in AI-powered embedded finance, you can unlock new revenue streams, drive innovation, and deliver value to customers.
The race is on, and the winners will be those who act decisively. Will you be one of them?
More AI in financial services: The place of AI in open banking solutions