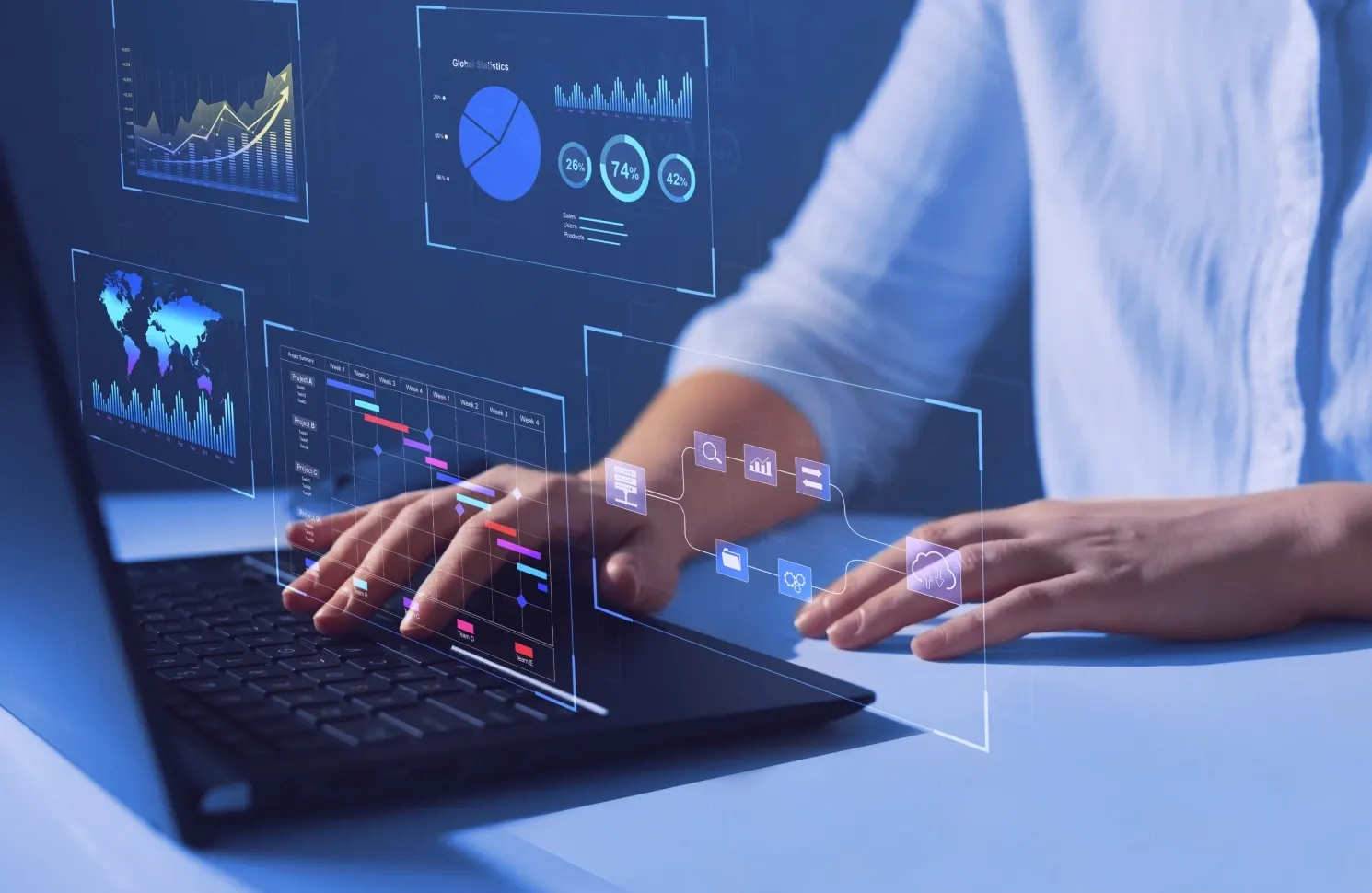
12 ways traditional data assessments lack modern platform capabilities and how to modernize them
APR. 7, 2025
2 Min Read
Traditional assessments fail in today's cloud-native, AI-driven world.
Data assessments remain critical for measuring organizational data maturity, identifying gaps, and defining transformation roadmaps. These evaluations have long helped enterprises strengthen their data strategy, governance, architecture, analytics, security, culture, and AI/ML capabilities.
However, traditional assessments primarily focus on legacy environments—evaluating structured data, on-premises infrastructure, and static governance models. They inadequately address modern data platform capabilities, including:
- Cloud-native architectures
- AI-driven automation
- Self-service analytics
- Data mesh and data fabric models
- MLOps and real-time decision intelligence
- Cost and sustainability optimization
At Lumenalta, we've conducted data assessments across numerous industries. While traditional frameworks provide valuable insights, they frequently overlook the dynamic, scalable, and AI-powered nature of today's data ecosystems.
What are the key areas where traditional assessments fall short? In this two-part series I’ll offer insights on how to reimagine assessment methodology for the modern data landscape.
1. Data strategy and governance: From static policies to adaptive frameworks
Traditional data governance approaches are rapidly becoming obsolete. While organizations historically focused on basic governance questions—such as strategy existence, review frequency, and role definition—these approaches fail to address the complex challenges of modern data ecosystems.
Legacy governance models create significant organizational barriers:
- Centralized decision-making slows critical business processes
- Restrictive access policies fragment data across organizational silos
- Inflexible frameworks struggle to adapt to multi-cloud and hybrid environments
The path to modern data governance
Modernizing governance requires a fundamental shift from documentation-centric to dynamic, value-driven approaches. Organizations must:
- Assess governance automation maturity
- Measure real-time policy enforcement effectiveness
- Validate alignment with business objectives
By embracing these modern capabilities, organizations can transform data governance from a restrictive administrative function to a strategic enabler of business innovation and efficiency.
Key capabilities of modern governance:
- Agile governance: Real-time policy enforcement with decentralized ownership
- Automated compliance: AI-driven continuous data profiling and anomaly detection
- Self-service controls: Dynamic SLA and metadata management
- Strategic alignment: Direct connection between governance and business outcomes
2. Data architecture: From centralized warehouses to distributed mesh and fabric
Traditional data architectures are rapidly becoming a competitive liability. Organizations trapped in legacy systems face critical challenges that undermine their ability to innovate, collaborate, and respond to dynamic business environments.
Monolithic architectures create significant organizational barriers:
- Limited scalability and flexibility
- Restricted cross-functional data accessibility
- Inefficient resource allocation and high infrastructure costs
- Inability to adapt to rapidly changing technological landscapes
The path to modern data architecture
Modernizing requires a fundamental shift from static infrastructure to dynamic, intelligent systems. Organizations must:
- Evaluate comprehensive data-sharing models
- Assess real-time processing capabilities
- Measure architecture flexibility and performance
By leveraging distributed, intelligent architectures, organizations can transform data from a static resource to a dynamic strategic asset—dramatically improving accessibility, reducing costs, and accelerating innovation.
Key capabilities of modern architecture
- Distributed ownership: Global interoperability with decentralized data management
- Event-driven processing: Real-time streaming architectures replacing batch systems
- AI-optimized transformation: Automated, metadata-driven data processing
- Adaptive scalability: Seamless resource optimization aligned with business needs
3. Analytics and business intelligence: From reports to AI-driven insights
Traditional business intelligence approaches are increasingly insufficient in today's data-driven landscape. Organizations clinging to manual reporting and static dashboards risk falling behind more agile, intelligent competitors.
Standard BI models create significant organizational constraints:
- Low tool adoption due to complexity
- Delayed decision-making processes
- Missed opportunities for AI-driven automation
- Inability to generate forward-looking, actionable insights
The path to modern analytics
Modernizing requires transforming analytics from retrospective reporting to predictive, intelligent decision support. Organizations must:
- Integrate real-time analytics capabilities
- Embed AI-driven decision intelligence
- Assess predictive analytics maturity
By embracing AI-driven, self-service intelligence, companies can transform data from a historical record to a strategic forecasting tool—accelerating decision-making and uncovering hidden opportunities.
Key capabilities of modern business intelligence:
- Conversational insights: Natural language AI analytics replacing static dashboards
- Embedded intelligence: Analytics integrated directly into business workflows
- Automated analysis: AI-powered insights with explainability and bias detection
- Predictive decision support: Forward-looking analytics driving strategic choices
4. Data security and compliance: From static rules to AI-driven threat detection
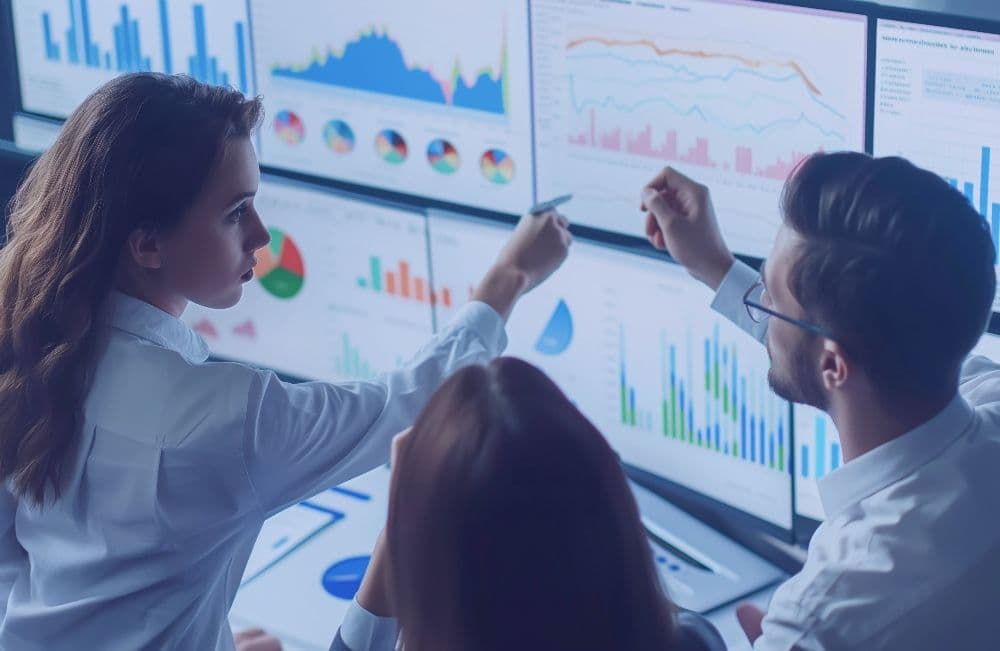
Traditional security approaches are increasingly vulnerable in today's complex digital landscape. Organizations relying on static policies and manual compliance checks are exposed to unprecedented cybersecurity risks.
Legacy security approaches create significant organizational vulnerabilities:
- Reactive rather than proactive threat detection
- Slow compliance enforcement
- Limited multi-cloud security capabilities
- Inability to adapt to evolving cyber threats
The path to modern security
Modernizing requires transforming security from a reactive checklist to an intelligent, adaptive system. Organizations must:
- Implement real-time threat intelligence
- Deploy AI-driven access controls
- Ensure dynamic regulatory compliance
AI-driven security frameworks enable businesses to transform data protection, from a compliance burden to a strategic competitive advantage—helping them anticipate threats and ensure robust, intelligent defense.
Key capabilities of modern security include:
- Dynamic access control: AI-powered anomaly detection replacing static policies
- Automated data protection: Intelligent masking of sensitive information
- Proactive threat intelligence: Continuous monitoring and predictive risk management
- Adaptive compliance: Real-time alignment with evolving regulatory requirements
5. Data culture and skills: From isolated expertise to organizational intelligence
Traditional approaches to data skills create barriers that prevent organizations from fully leveraging their most valuable asset—data. Siloed knowledge and limited training leave most employees disconnected from the transformative potential of modern data technologies.
Traditional data skill development creates significant organizational challenges:
- Low adoption of data-driven decision-making
- Persistent skills gaps in emerging technologies
- Limited cross-functional data collaboration
- Resistance to technological transformation
The path to modern data literacy
Modernizing requires transforming data from a specialized function to an organizational capability. Organizations must:
- Implement comprehensive data literacy programs
- Provide hands-on AI and automation training
- Enable cross-functional data sharing
By cultivating a data-intelligent culture, organizations can transform data from a technical specialty to a universal organizational language—driving innovation, efficiency, and strategic decision-making.
Key capabilities of modern data culture:
- Widespread data literacy: Training that empowers all employees
- Cross-functional collaboration: Breaking down departmental data silos
- AI skills development: Practical experience with cutting-edge technologies
- Self-service analytics: Democratizing data access and insights
6. Master data management (MDM): From static repositories to intelligent data ecosystems
Traditional master data management approaches are rapidly becoming obsolete in an era of complex, distributed business environments. Organizations clinging to rigid, centralized data models risk operational inefficiency and competitive disadvantage.
Traditional master data management creates significant organizational challenges:
- Persistent data inconsistencies
- Limited scalability across cloud platforms
- High maintenance costs for outdated systems
- Inflexible data governance frameworks
The path to modern MDM
Modernizing requires transforming master data from a static repository to a dynamic, intelligent asset. Here’s how companies can achieve this:
- Implement AI-driven data quality mechanisms
- Enable flexible, domain-driven data ownership
- Integrate seamlessly with cloud and SaaS ecosystems
An intelligent master data management helps companies transform data from a passive record to an active, strategic asset—driving operational efficiency and enabling more informed decision-making.
Key capabilities of modern MDM:
- Intelligent Data Matching: AI-powered deduplication and enrichment
- Federated Governance: Balancing global standards with local flexibility
- Cloud-Native Integration: Seamless support for hybrid and multi-cloud environments
- Adaptive Data Management: Continuous quality and accuracy improvements
7. Metadata management
Traditional metadata approaches are rapidly becoming inadequate in complex, data-driven organizations. Static catalogs and manual tracking create significant barriers to understanding and leveraging organizational data assets.
Traditional metadata approaches create significant organizational challenges:
- Limited visibility into data assets
- Increased compliance and security risks
- Reduced efficiency in analytics and reporting
- Difficulty tracking data transformations
The path to modern metadata management
Modernizing requires transforming metadata from a passive record to an active intelligence layer. To do this, organizations must:
- Implement AI-driven metadata discovery
- Enable intelligent, context-aware recommendations
- Ensure comprehensive data lineage tracking
By embracing intelligent metadata management, they can transform data documentation from a compliance burden to a strategic asset—providing unprecedented visibility and insights across their data ecosystem.
Key capabilities of modern metadata management:
- Automated Discovery: AI-powered continuous metadata capture
- Intelligent Insights: Metadata-driven governance and security recommendations
- End-to-End Traceability: Comprehensive data lineage and impact analysis
- Self-Service Intelligence: Empowering users with discoverable, actionable metadata
8. Data quality and observability: From reactive monitoring to predictive intelligence
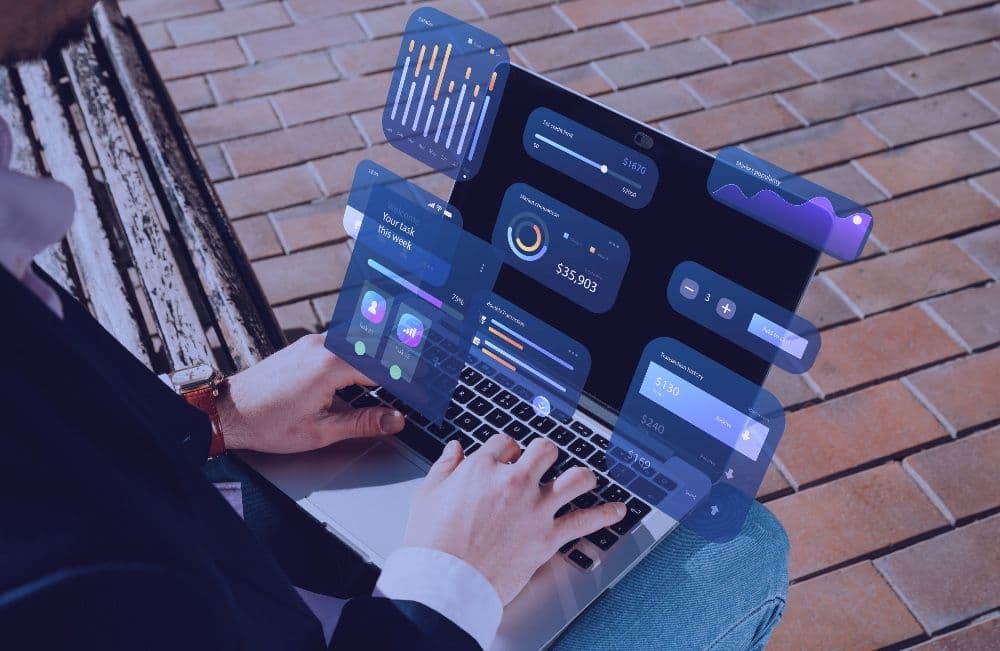
Traditional data quality approaches are increasingly insufficient in today's complex, data-driven environments. Manual monitoring and reactive problem-solving create significant risks for organizational decision-making.
Traditional data quality models create critical organizational challenges:
- Persistent data inconsistencies
- Reactive rather than proactive issue resolution
- High operational costs of manual monitoring
- Eroding trust in analytics and insights
The path to modern data quality
Modernizing requires transforming data quality from a reactive checklist to an intelligent, predictive system. Organizations must:
- Implement AI-driven continuous monitoring
- Enable real-time data health tracking
- Develop predictive anomaly prevention
Intelligent data quality frameworks enable businesses to transform data validation from a technical burden to a strategic advantage—ensuring decision-making is consistently accurate, reliable, and insights-driven.
Key capabilities of modern data quality include:
- Automated Anomaly Detection: AI-powered continuous quality monitoring
- End-to-End Observability: Real-time tracking of data health and lineage
- Predictive Issue Resolution: Proactive prevention of data failures
- Intelligent Validation: Automated, context-aware data reconciliation
9. Data monetization: From internal reporting to strategic asset
Traditional approaches to data value creation are increasingly inadequate in a digital economy where data represents a critical competitive resource. Organizations that view data merely as a reporting tool miss significant opportunities for revenue and strategic advantage.
Traditional data utilization creates substantial organizational constraints:
- Unrealized revenue potential from data assets
- Ineffective competitive differentiation
- Limited insights for strategic decision-making
- Missed opportunities in emerging data marketplaces
The path to modern data monetization
Modernizing requires transforming data from a passive resource to an active revenue generator. Organizations must:
- Develop comprehensive data-as-a-service strategies
- Create AI-powered insights platforms
- Explore direct and indirect monetization models
With intelligent data, organizations can turn their data from a cost center to a strategic asset—generating revenue, driving innovation, and creating unprecedented business value. Key capabilities include:
- Data as a service (DaaS): Generating revenue through APIs and partnerships
- AI-Driven insights: Enhancing products with intelligent analytics
- Embedded monetization: Creating diverse revenue streams from data assets
- Strategic value generation: Transforming data into a competitive differentiator
10. Cloud and infrastructure modernization: From static deployments to dynamic ecosystems
Traditional cloud approaches are rapidly becoming obsolete in an era of complex, distributed computing environments. Organizations clinging to monolithic infrastructure models risk significant competitive disadvantages.
Traditional cloud adoption creates substantial organizational challenges:
- Escalating infrastructure costs
- Limited technological flexibility
- Increased security and compliance risks
- Reduced operational agility
The path to intelligent cloud infrastructures
Modernizing requires transforming cloud strategy from a technical implementation to a strategic business capability. To do this, organizations must:
- Develop multi-cloud and hybrid strategies
- Implement serverless, scalable architectures
- Enable intelligent cost optimization
A modern cloud strategy enables a company to transform infrastructure from a cost center to a dynamic, value-generating ecosystem—driving innovation, flexibility, and competitive advantage.
Key capabilities of modern infrastructures:
- Multi-cloud flexibility: Leveraging best-of-breed cloud providers
- Serverless architecture: Reducing operational overhead
- FinOps optimization: Intelligent resource management
- Sustainable computing: Balancing performance and cost efficiency
11. Real-time data processing: From batch updates to continuous intelligence
Traditional data processing approaches are increasingly inadequate in a world demanding instant insights and immediate action. Organizations relying on batch processing risk becoming obsolete in a rapidly evolving digital landscape.
Standard data processing models create critical organizational challenges:
- Inability to respond to time-sensitive events
- Significant delays in critical business analytics
- Missed opportunities for proactive decision-making
- Limited operational responsiveness
The path to real-time data processing
Modernizing requires transforming data processing from static updates to dynamic, continuous intelligence. Organizations must:
- Implement event-driven architectures
- Enable real-time streaming analytics
- Develop operational intelligence platforms
By embracing intelligent streaming architectures, organizations can transform data processing from a retrospective exercise to a forward-looking, dynamic business capability—driving innovation, responsiveness, and competitive advantage.
Key capabilities of modern data streaming:
- Event-driven architecture: Capturing and analyzing data in motion
- Streaming analytics: AI-powered insights from real-time data streams
- Operational intelligence: Enhancing decision-making across business processes
- Continuous optimization: Transforming data into immediate actionable insights
12. Ethical AI and responsible data use: From compliance checkbox to strategic responsibility
Traditional approaches to AI ethics are rapidly becoming insufficient in an era of increasing technological complexity and societal scrutiny. Organizations treating ethical AI as a mere compliance exercise risk significant legal, reputational, and operational challenges.
Legacy AI ethics models create critical organizational risks:
- Potential regulatory non-compliance
- Systemic bias in decision-making algorithms
- Erosion of stakeholder trust
- Limited transparency in AI-driven processes
The path to real-time data processing
Modernizing requires transforming ethical AI from a reactive compliance function to a proactive strategic capability. Organizations must:
- Implement comprehensive bias mitigation strategies
- Develop robust explainability frameworks
- Ensure rigorous privacy and governance standards
By leveraging intelligent ethical AI frameworks, organizations can transform AI governance from a potential liability to a strategic differentiator—building trust, ensuring fairness, and demonstrating technological leadership.
Key capabilities of ethical AI include:
- Fairness by design: Proactive bias detection and mitigation
- Transparent decision-making: Interpretable and justifiable AI models
- Regulatory alignment: Comprehensive compliance with global standards
- Responsible innovation: Balancing technological advancement with ethical considerations
Measurable data maturity for modern data assessments
This table provides measurable criteria for each data maturity category, focusing on quantifiable aspects and outcomes to enable a more objective assessment. These metrics can be tailored to the specific needs and goals of an organization, offering a flexible yet comprehensive roadmap for digital transformation.
Data category | Basic (1) | Developing (2) | Mature (3) | Advanced (4) | Leading (5) |
---|---|---|---|---|---|
Data strategy & governance | Documented strategy, manual oversight, reactive | Formalized strategy with KPIs, regular reviews, role-based access | Automated policy enforcement, data contracts, aligned with business goals | Real-time policy enforcement, AI-driven governance, self-service data access | Industry benchmark, drives innovation & business value |
Data architecture | Siloed, on-premises, batch-oriented | Hybrid, some cloud adoption, ETL-based | Data lakehouse/fabric, serverless, AI/ML-optimized | Multi-cloud, event-driven, cost-optimized | Dynamic, adaptable, and future-proof |
Analytics & BI | Manual reporting, limited adoption | Standardized reports, dashboards, some self-service | Embedded analytics, self-service AI/ML, automated insights | Predictive analytics, NLP-driven insights, decision intelligence | Pervasive, real-time insights drive all decisions |
Data security & compliance | Basic access controls, manual compliance | Role-based access, encryption, regular audits | Automated data masking, AI-driven threat detection | Zero-trust, dynamic access control, proactive compliance | Anticipates and adapts to future threats and regulations |
Data culture & skills | Limited data literacy, siloed work | Data training programs, some collaboration | Cross-functional data teams, self-service analytics | Data-driven culture, AI/ML literacy, citizen data scientists | Data is a core strategic asset, culture of innovation |
MDM | Siloed data, manual processes | Centralized repository, basic data quality rules | AI-driven data matching & cleansing, cloud integration | Federated MDM, golden record for key entities, data lineage | Trusted single source of truth, drives business outcomes |
Metadata management | Manual metadata entry, limited lineage | Metadata repository, basic lineage tracking | Automated metadata discovery, active metadata | End-to-end data lineage, impact analysis | Metadata drives automation and insights |
Data quality & observability | Reactive data quality checks | Data quality KPIs, periodic audits | Automated data quality monitoring, anomaly detection | End-to-end observability, proactive issue resolution | Data quality is a competitive advantage |
Data monetization | No monetization strategy | Explores data monetization, basic metrics | Data products, revenue generation, clear ROI | Data as a Service (DaaS), AI-driven insights | Significant revenue and competitive advantage from data |
Cloud & infrastructure | On-premises, limited cloud | Cloud migration in progress, basic cost management | Multi-cloud, serverless, FinOps | Cloud-native, optimized, sustainable | Drives agility and innovation |
Real-time Processing | Batch processing, high latency | Near real-time, some streaming | Event-driven architecture, streaming analytics | Real-time insights, automated actions | Business operates in real-time |
Ethical AI & data use | No formal guidelines | AI ethics policy, basic bias detection | Explainable AI, fairness assessments | Proactive bias mitigation, AI compliance | Ethical AI is a core value, drives trust |
Rethinking data assessments for the AI-driven era
Traditional data assessments, while foundational, are no longer sufficient in an era defined by AI-driven innovation and cloud-scale complexity. As highlighted throughout this post, legacy frameworks fail to account for critical modern capabilities such as agile governance, data mesh architectures, real-time decision intelligence, and ethical AI enforcement. Organizations that rely solely on outdated methodologies risk inefficiency, compliance gaps, and missed opportunities for data monetization.
The path forward demands a holistic approach to assessment—one that evaluates not just technical infrastructure but also cultural readiness, automation maturity, and ethical alignment. Modern data ecosystems thrive when assessments prioritize:
- Adaptability: Policies and architectures that evolve with business needs and technological advancements.
- Automation: AI-driven governance, self-service analytics, and proactive data quality enforcement.
- Actionable insights: Real-time analytics embedded into workflows and AI-powered predictive capabilities.
- Accountability: Ethical AI practices, transparency, and cross-functional collaboration.
At Lumenalta, we’ve seen firsthand how organizations that modernize their assessment strategies unlock faster innovation cycles, reduce operational costs, and build stakeholder trust. The transition from static checklists to dynamic, value-driven evaluations isn’t just an upgrade—it’s a strategic imperative.
In a world where data is the ultimate differentiator, your assessment framework must be as agile, intelligent, and forward-looking as the platforms it evaluates. By measuring capabilities like AI-driven automation, decentralized governance, and real-time observability, businesses can transform data from a passive asset into a catalyst for growth.
The time to rethink is now: Future-proof your data strategy by measuring what truly matters in the age of AI and cloud. Let your assessments reflect the speed, scale, and sophistication of the modern data ecosystem—or risk falling behind those who do.
Ready to transform your data assessment framework in your organization?