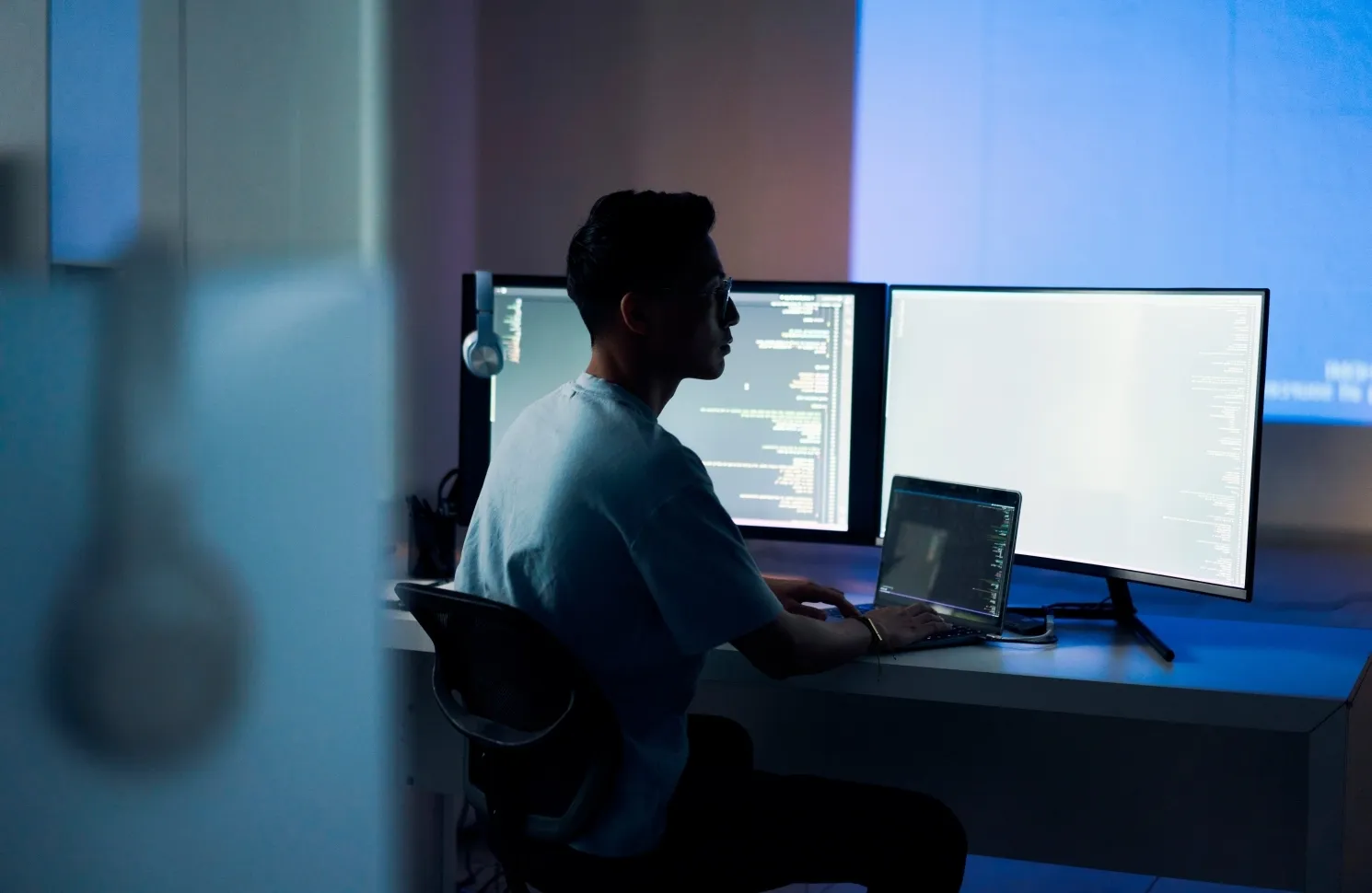
7 ways AI boosts your software QA process
MAR. 11, 2024
2 Min Read
AI improves the software QA process in seven significant areas that add value and speed to the testing process.
Software quality assurance (QA) has come a long way with the support of AI resources in the past few years. It expedites testing processes and increases accuracy that can be achieved without human mediation.
At first glance, the more predictive analytical capabilities and cognitive solutioning of AI don’t seem to translate into the QA space; however, AI not only accelerates the process of testing, but also introduces a level of efficiency that significantly elevates overall software quality.
As Product Quality Lead at Lumenalta, I’ve managed the testing process of multimillion-dollar company projects in many industries. During my tenure, I’ve developed an understanding of the many different features that are important when testing — voice recognition, virtual reality, machine learning — to plan for all aspects of the process.
Inadequate software QA can cost thousands
Not investing in QA might save you money in the upfront, but will cost you later on. As quality assurance analysts, my team is always looking to find efficiency for our clients.
The use of AI is growing in automated testing to cut down on ineffective software testing procedures and locate the issues that human eyes find it hard to pinpoint.
7 ways AI can reshape software quality assurance
With this in mind, here are a few insights on how my product team is experimenting with a few AI tools in quality assurance to add value and speed to the software testing process.
1. Generates edge test case scenarios instantly
One of the most time-consuming aspects of QA has been generating comprehensive test cases — it can take more than 80 hours to write test cases for a single project, depending on its complexity.
With AI in the picture, we can instantly generate edge cases by simply providing the tool with the application’s attributes and acceptance criteria. This not only saves valuable time, but significantly enhances test coverage, ensuring we catch potential issues before they manifest.
2. Accelerates test automation
Behavioral-Driven Development (BDD) test scenarios support non-technical team members in understanding the software project. Crafting these with AI has led to more efficient scenario-based testing.
The outcome is a QA automation framework that mirrors user expectations and ensures our software functions seamlessly.
3. Eliminates redundancies with AI precision
As a QA professional, I’m aware of how hard it can be to identify redundant test cases. AI’s precision in analyzing and eliminating redundancy has been a game-changer. It’s like having an additional set of meticulous eyes, ensuring that every test conducted contributes meaningfully to our software quality assurance efforts.
4. Reveals complex software connections
AI’s capacity for analysis goes beyond surface-level insights and can uncover complex connections between software issues that might have eluded our manual scrutiny. This depth enables us to address intricate interdependencies, fortifying the reliability and performance of our software.
5. Automates test execution scheduling
Managing test execution schedules used to be a logistical challenge. AI’s intervention automates this process, delegating tasks and setting timelines for each test case. This not only optimizes our resource utilization but ensures a systematic and timely testing cycle.
6. Detects issues earlier in the process
Detecting issues in the beginning stages of the project’s life cycle has always been a high-priority goal — and AI’s efficient algorithms achieve just that. Proactive issue detection from the outset saves us valuable time and resources, preventing potential roadblocks down the development road.
7. Strengthens strategic focus on software QA
With AI seamlessly handling automatable components, our QA team can redirect its focus strategically. We’ve been able to devote more attention to creating a streamlined QA strategy that aligns with organizational goals. This strategic approach ensures a comprehensive and efficient testing process.
AI isn’t merely about adopting new technology; it’s about embracing a mindset that propels testing towards unprecedented efficiency, speed, and precision.
In the journey of testing, the convergence of AI and innovation in QA promises a future where testing excellence becomes the norm.