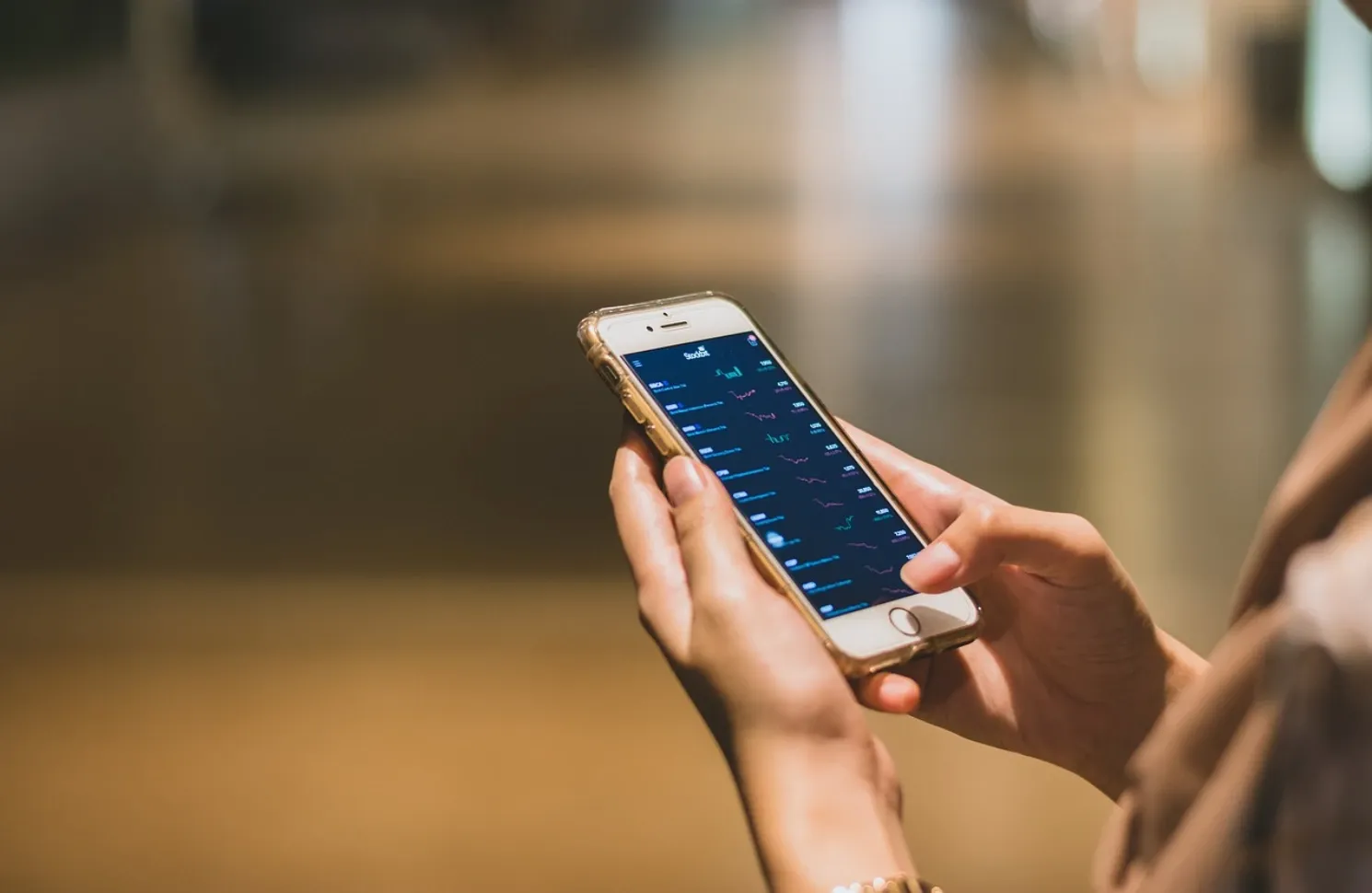
Robust safeguards for the real-time payment revolution
SEP. 16, 2024
2 Min Read
As real-time payments revolutionize the financial landscape with their speed and efficiency, financial institutions face the dual challenge of harnessing their potential while fortifying defenses against escalating fraud risks.
Payments and bank transfers have long been the tortoise of the financial world. This is changing with the increasing popularity of real-time payments (RTPs). No more waiting several business days for a transfer to settle; with RTPs, it happens instantaneously, 24/7. At the same time, international payments and instant cross-border payments are also gaining momentum.
Real-time payments are a boon to convenience and efficiency and can improve cash flow and liquidity management, but this new frontier has exposed vulnerabilities in the financial system.
To realize the transformative potential of RTPs while continuing to battle fraud, financial institutions must embrace platforms and machine learning (ML) to keep pace with and win against ever-increasing fraud threats. With the introduction of FedNow in 2023, the U.S. now has two Clearing and Settlement Mechanism (CSM) providers, providing an alternative to the RTP network from The Clearing House (TCH).
Security challenges presented by real-time payments
The breakneck speed that makes RTP so popular is also its Achilles’ heel. Traditional security measures are ill-equipped to handle the near-instantaneous nature of these payments, leaving a gap that criminals are all too eager to exploit.
The irrevocable nature of RTPs further exacerbates the problem. Scammers are winning and fraud has reached a crisis level.
Although regulators and financial institutions are starting to address and collaborate more on fraud detection and prevention, much more needs to happen across the industry while balancing transparency and competition. Fraud schemes are constantly evolving and becoming more sophisticated, often requiring coordination across actors, channels, institutions, and law enforcement to properly investigate, if not prevent.
With Authorized Push Payment (APP) fraud leading the pack in terms of total dollar impact, banks need to ensure their RTP fraud prevention capabilities can operate better at scale compared to their proficiency with credit and debit card transactions across consumers and businesses alike.
These threats span a wide range of business transactions including account-to-account credit transfers, consumer-to-business bill pay, title insurance, mortgage closing, B2B invoicing, C2B ecommerce, and B2C payroll. In addition, fraud threats are becoming more prevalent, with scams spanning investments, purchases of goods, sweepstakes and lotteries, romance, advance fees, invoicing, and impersonation.
Standard security playbooks won’t suffice in this new landscape. So, financial institutions need a fresh approach that keeps up with the speed of real-time payments.
Transactional fraud models have been good at fighting account takeovers, but RTP transaction fraud requires more sophistication where transactions often look authentic. You need RTP-specific rules and data sets and real-time customer experience.
Balancing security and user experience in real-time payments
Financial institutions face a delicate balancing act: implementing robust security measures while maintaining a smooth user experience for real-time payments (RTPs). The goal should be to incorporate just enough “warranted friction” to enhance security without sacrificing convenience.
RTPs introduce a new payment channel that must be managed alongside traditional methods like ACH, credit, and debit cards. This complexity requires a nuanced approach to security and user experience.
Forward-thinking institutions view payments analytics not just as a risk management tool, but as a revenue driver. Advanced machine learning fraud models offer precise behavioral analytics at scale, simultaneously thwarting malicious actors and unlocking personalization opportunities for legitimate customers.
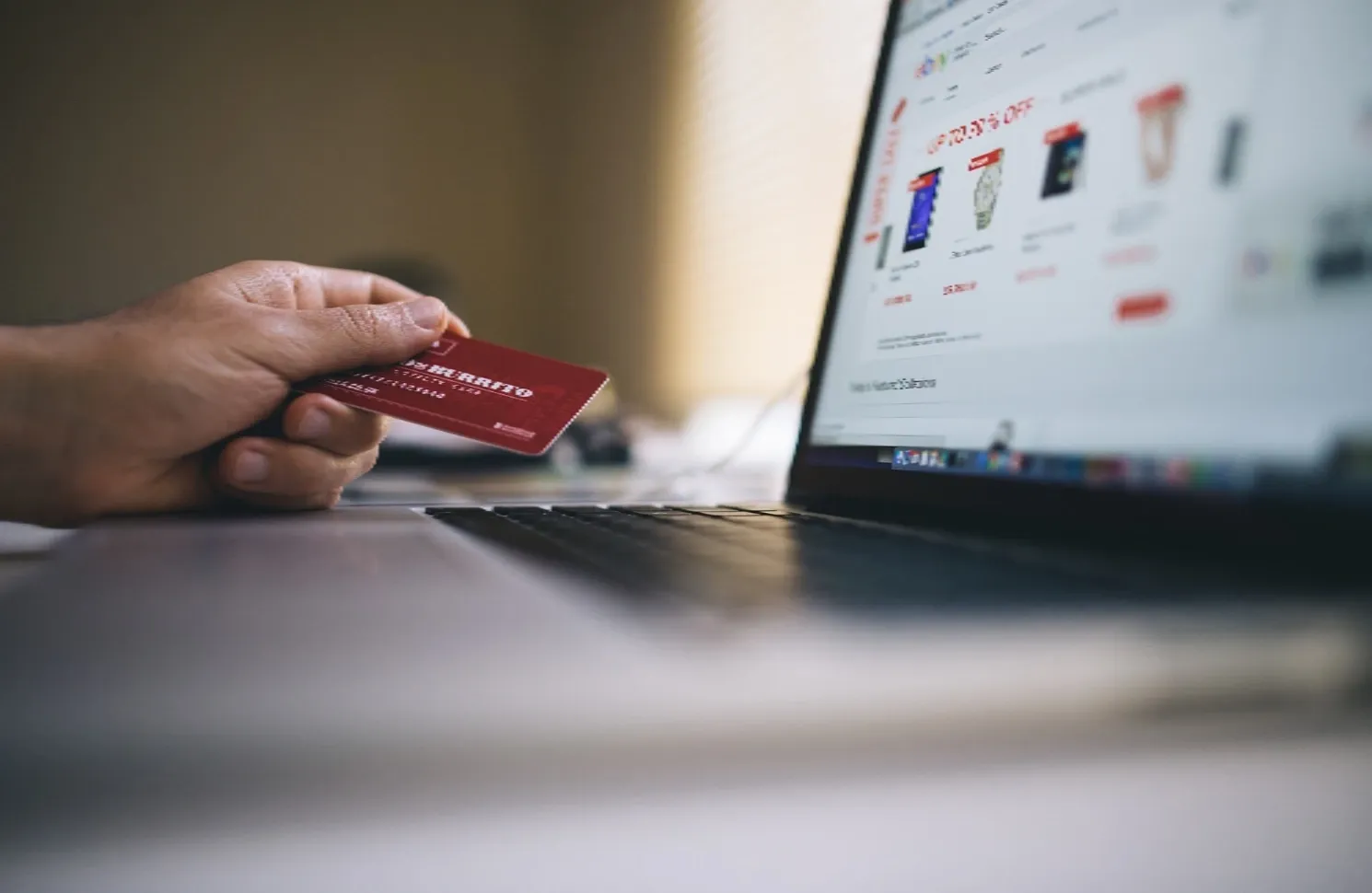
- Multi-factor authentication
- One-time passwords
- Biometric verification
- Multi-signature approvals for commercial payments
To further enhance security while building trust, institutions can empower customers to customize fraud alert notifications across their preferred channels (mobile, email, social media, or banking apps). This approach engages customers in the fraud detection process and helps protect them from emerging scams.
By striking the right balance between security and usability, financial institutions can deliver on the promise of RTP convenience while safeguarding their customers and systems.
Key security considerations
The unique risks presented by RTPs demand a multi-pronged approach that confronts vulnerabilities head-on. Let’s delve into the key areas that financial institutions and technology providers must address to ensure the safety and integrity of real-time transactions.
Preventing fraud in the first place is far more effective than picking up the pieces after it happens. That's why fraud monitoring tools are indispensable for ensuring your RTPs stay secure.
Emerging technologies and strategies
As real-time payments evolve, so must the tools and strategies we employ to secure them. Here are some of the technologies banks are using to stay one step ahead of fraudsters:
Biometrics and advanced authentication
Biometrics offers a solution that's both more secure and user-friendly than passwords. By harnessing the power of our unique biological signatures—think fingerprints, facial features, and even the way we type—proving one's identity is as simple as a touch or a glance.
MFA (multi-factor authentication) adds another security layer by requiring users to supply proof of their identity. And for those high-stakes transactions, risk-based adaptive authentication kicks in, dynamically adjusting security requirements based on the perceived level of risk.
These advanced authentication methods both thwart unauthorized access and streamline the payment process. It's a win-win for everyone involved.
Secure APIs and open banking framework
Interoperability, security, and transparency are some of the most notable benefits of an open banking framework and ISO 20022.
Secure APIs act as connective tissue—they allow financial institutions and third-party providers to seamlessly exchange data and services while upholding stringent security protocols and controlled data access. FedNow provides capabilities for fraud prevention at the network and participant level and via participant-defined negative lists.
Third parties can provide KYC information, aggregated transaction data, channel data, financial information data, and ratings. Financial institutions should seek to enrich their intelligence and be supported by a solution that provides connectivity to these data sources.
Artificial intelligence and machine learning
Artificial intelligence (AI) and machine learning (ML) are the brains behind a new generation of fraud analytics and predictive modeling in financial services. These technologies provide multiple layers of protection against threats, reducing losses and improving transaction approval rates by continuously analyzing data to flag suspicious patterns and anomalies.
- Machine learning algorithms, particularly supervised learning models like decision trees and neural networks, are used extensively to identify fraudulent transactions by learning from historical data. These models can distinguish between legitimate and fraudulent transactions by recognizing subtle patterns that might be missed by traditional rule-based systems.Decision trees can be used to classify transactions as fraudulent or nonfraudulent by evaluating various attributes, such as transaction amount, location, and time.
- Unsupervised learning methods, including clustering and anomaly detection, are used to detect novel fraud schemes by identifying outliers in transaction data that do not conform to expected behavior.Anomaly detection algorithms are designed to identify rare or unusual patterns that deviate from the norm. These algorithms are particularly effective in fraud detection, as fraudulent transactions often exhibit anomalous behavior compared to regular transactions. Techniques such as k-means clustering, Isolation Forest, and OneClassSVM are commonly used for anomaly detection.
- Deep learning is a subset of machine learning that uses neural networks with many layers to model complex patterns in data. Deep learning techniques have shown great success in various applications, including fraud detection.
Convolutional Neural Networks (CNNs) are primarily used for image and spatial data analysis but can also be used for fraud detection by treating transaction data as multi-dimensional. CNNs use convolutional layers to automatically extract relevant features from the input data.
In fraud detection, CNNs can be used to analyze transaction sequences and patterns over time. By capturing spatial relationships within the data, CNNs can detect subtle and complex fraud patterns that may not be apparent using traditional methods.
Recurrent Neural Networks (RNNs) are designed to handle sequential data and time-series analysis. They can retain information from previous inputs, making them suitable for temporal dependencies.
RNNs are useful in fraud detection for analyzing transaction histories and identifying suspicious patterns over time. For example, they can detect fraudulent behaviors that involve a series of transactions across different time periods, which may be indicative of money laundering or other sophisticated schemes.
- Natural language processing can help detect fraud in written communication by analyzing textual data such as emails and transaction descriptions to identify suspicious language and patterns. For example, certain terms and language commonly used in phishing emails can be flagged as potential fraud indicators.
These models aren’t just good at finding clues—they're also quick learners. And they become more effective over time by learning signals typically associated with fraud with high degrees of granularity and precision.
Balancing act: Reducing false positives
A key challenge in fraud prevention is minimizing false positives while maximizing fraud detection. AI and ML solutions help strike this balance by:
- Refining detection rules based on learned patterns
- Adapting to new fraud techniques in real-time
- Providing more accurate risk assessments for individual transactions
Customized real-time ML techniques
To enhance fraud prevention, financial institutions should:
- Build features that confirm the sender's identity and intentions
- Establish behavioral baselines for customers
- Implement actions for when abnormalities arise
In addition, specialized behavior-sorted lists (B-lists) can be particularly effective. These lists monitor attributes of a customer’s payment history to differentiate normal behavior from suspicious anomalies, improving the accuracy of fraud detection while reducing friction for legitimate users.
Collaboration between banks, Fintechs, and regulators
Building a secure RTP ecosystem is a team effort. Each player brings a unique perspective and expertise to the table—banks provide stability, Fintechs drive innovation, and regulators demand accountability.
While they may be pulling in different directions, these stakeholders can work together to create a cohesive security framework that benefits everyone. This means sharing threat intelligence, developing industry standards, and fostering an environment where innovation can thrive responsibly.
Network and counter-party collaboration is critical for sharing data characteristics of fraudulent transactions to combat fraud.
Continuous improvement and adaptation
In the high-stakes cat-and-mouse game between financial institutions and scammers, keeping up with the evolving threat landscape requires a proactive and adaptive approach.
With their ability to identify fraudulent transaction patterns and iterate accordingly, AI and ML are game changers. They give financial institutions the power to detect and neutralize fraudulent activity before it wreaks havoc.
These technologies are a crucial component of financial fraud detection software for banks — the kind that leading firms are adopting to stay one step ahead of cybercriminals.
But when it comes to implementing next-generation threat detection systems, it’s best to leave it to the pros. That way, you can focus on what you do best—keeping your customers satisfied.
The experts at Lumenalta bring decades of experience in the finance world and are ready to help you craft real-time payment solutions that meet your unique needs and goals. Contact us to start customizing your financial operations.