Whether assessing data, evaluating risks, or forming hypotheses, selecting the right approach determines the accuracy and reliability of conclusions. Deductive, inductive, and abductive reasoning each serves unique functions, guiding professionals across industries such as law, research, finance, and healthcare. Understanding how these methods differ—and knowing when to apply each—improves accuracy in decision-making, strengthens analytical thinking, and enhances strategic outcomes.
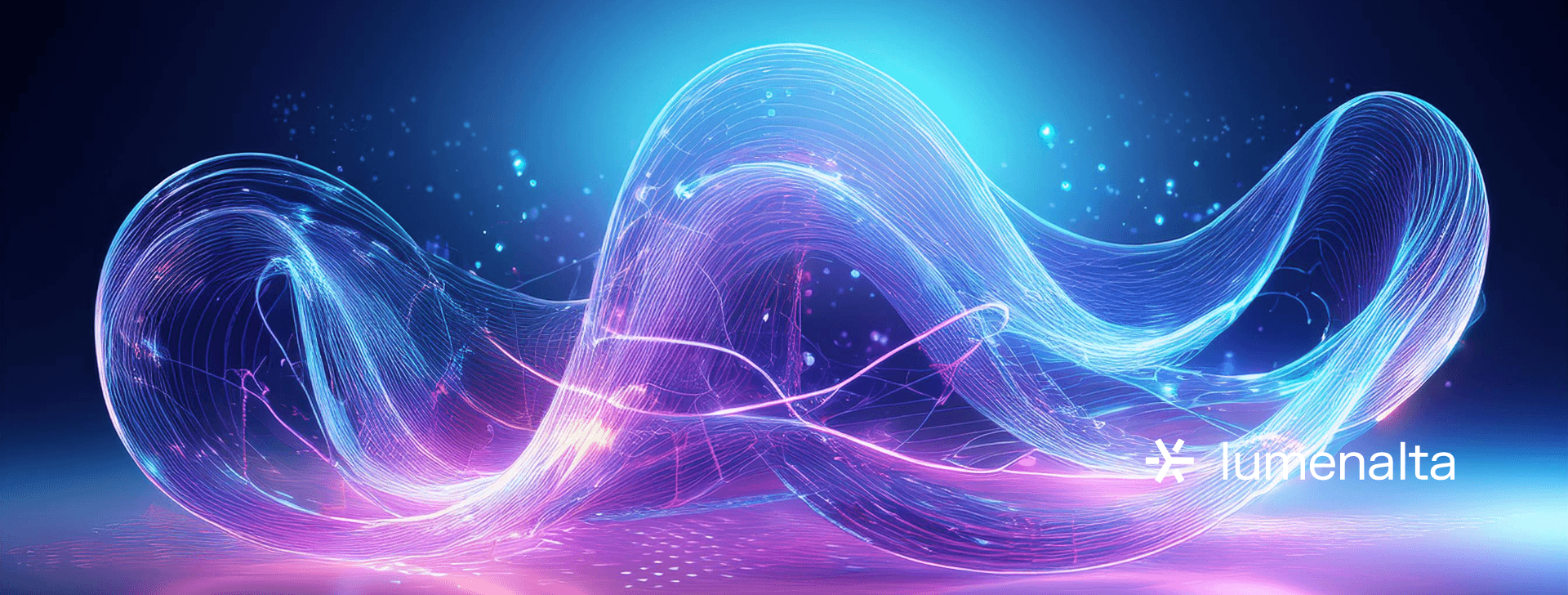
Deductive reasoning vs inductive reasoning
FEB. 21, 2025
5 Min Read
Logical reasoning is critical in shaping conclusions, analyzing information, and refining problem-solving strategies.
Key takeaways
- 1. Deductive reasoning applies general principles to specific cases, producing conclusions that are logically certain when premises are correct.
- 2. Inductive reasoning identifies patterns from specific observations to form generalizations, making it useful for research, forecasting, and trend analysis.
- 3. Abductive reasoning determines the most plausible explanation based on incomplete data, commonly used in medical diagnosis, investigations, and troubleshooting.
- 4. Different reasoning methods apply to distinct industries, with deductive reasoning ideal for structured logic, inductive reasoning for predictive analysis, and abductive reasoning for best-guess assessments.
- 5. Choosing the right reasoning method strengthens accuracy, enhances strategic thinking, and improves problem-solving in fields that rely on data-led conclusions.
What is deductive reasoning?
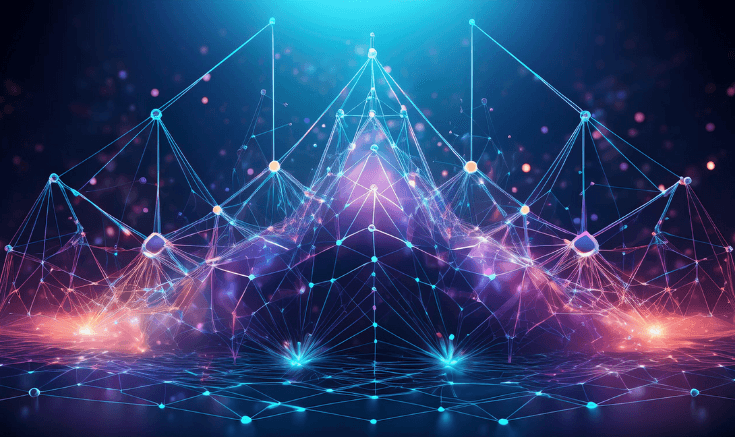
Logical consistency plays a central role in fields that require accuracy, making deductive reasoning a critical approach for professionals who rely on facts. This method follows a structured path, moving from general premises to specific conclusions. When applied correctly, it eliminates uncertainty and strengthens analytical outcomes. Legal experts, scientists, and analysts frequently use deductive reasoning to validate claims, test hypotheses, and assess financial models. Unlike methods based on observation or probabilities, this reasoning process depends entirely on factual statements, making it a dependable tool for those who require precise conclusions.
Why deductive reasoning is important
Logical accuracy plays a crucial role in industries that require objective conclusions. Professionals in law use deductive reasoning to apply legal statutes to specific cases, while researchers use it to test theories through controlled experiments. Analysts apply this method when evaluating financial models, identifying risks, and assessing profitability. This logical approach strengthens the accuracy of conclusions by eliminating reliance on speculation and assumption-based reasoning.
Many industries benefit from this structured reasoning method, allowing professionals to work with facts instead of probabilities. Stronger conclusions lead to more reliable projections, helping individuals and organizations reduce uncertainty when making assessments.
Key components of deductive reasoning
Logical reasoning follows a set structure that determines the accuracy of conclusions. Each component plays a critical role in supporting arguments and reinforcing credibility.
- Premises: Statements that establish a logical foundation. The accuracy of these statements determines the reliability of the conclusion.
- Logical structure: A reasoning pattern that follows a clear sequence to maintain logical consistency.
- Conclusion: The final result that follows from the premises, assuming logical accuracy is maintained.
- Validity and soundness: A valid argument maintains a correct logical structure, while a sound argument includes premises that are both factual and logical.
Strong conclusions depend on the accuracy of these components. Logical consistency supports clear reasoning, reinforcing conclusions in fields that require factual assessments.
Examples of deductive reasoning
Practical applications of deductive reasoning appear in industries where accuracy is critical. Each example demonstrates how this method leads to sound conclusions logically.
- Mathematics: Squares have four sides. A specific shape is identified as a square. That shape must have four sides.
- Law enforcement: Theft violates the law. An individual has taken property without permission. That individual has broken the law.
- Business analysis: Higher production costs without price adjustments lead to lower profit margins. An organization increases production costs but keeps prices the same. Profit margins decrease.
- Scientific research: Water boils at 100°C under standard atmospheric pressure. A pot of water is heated to 100°C at sea level. The water boils.
Logical reasoning strengthens assessments by reinforcing conclusions based on factual premises. Those who apply this method eliminate unnecessary risks tied to assumption-based rationale, making it a valuable tool in research, law, and financial analysis.
"Data security focuses on safeguarding digital assets from unauthorized access, data privacy controls how information is collected and shared, and data protection enforces policies to maintain confidentiality, integrity, and availability."
What is inductive reasoning?
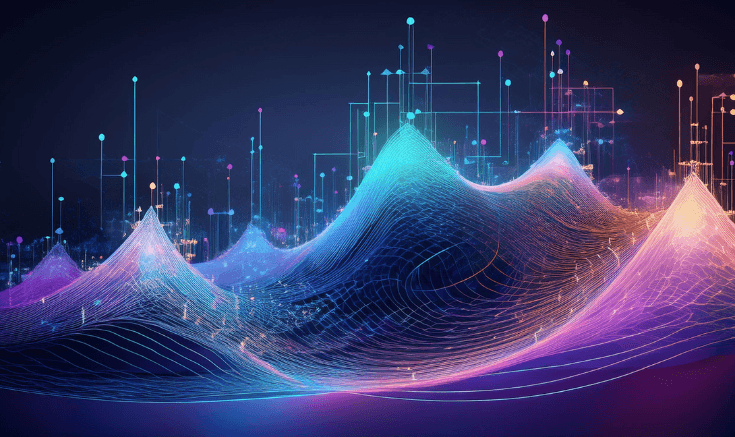
Inductive reasoning builds conclusions based on specific observations, identifying patterns that suggest broader generalizations. Unlike deductive reasoning, which guarantees a logically certain conclusion when premises are accurate, inductive reasoning deals with probability. Scientists, market analysts, and financial experts rely on this approach to form predictions, assess trends, and develop theories based on observed data. By analyzing repeated occurrences, professionals refine strategies and make informed assessments without relying on rigid formulas.
Since inductive reasoning draws insights from specific examples, conclusions remain flexible and adaptable as new information becomes available. This approach plays a significant role in forecasting, allowing industries to anticipate shifts in consumer behavior, financial markets, and operational performance. Applying inductive reasoning correctly strengthens the ability to recognize meaningful patterns, helping professionals adapt to emerging trends and refine strategies more confidently.
Why inductive reasoning is important
Recognizing trends from observed data allows industries to anticipate potential outcomes and refine their methods accordingly. Scientists use inductive reasoning to construct theories after analyzing repeated experimental results, making it a foundation of research-based fields. Market analysts track customer purchasing behaviors and identify emerging preferences by studying sales data, helping organizations refine their products and services. Financial experts assess investment trends by examining previous market fluctuations, allowing them to anticipate potential risks.
Since inductive reasoning generates insights rather than absolute conclusions, professionals gain the flexibility to adjust predictions as conditions shift. This method strengthens the ability to anticipate risks, optimize strategies, and refine operations based on developing information.
Key components of inductive reasoning
Drawing logical conclusions from observations requires several essential elements. The reliability of inductive reasoning depends on the consistency and accuracy of these components.
- Observations: Collected data that forms the basis for identifying recurring patterns and trends.
- Patterns and trends: Recognizable relationships within observed data that suggest possible outcomes.
- Generalization: Broad conclusions formed by analyzing multiple data points that exhibit similar behaviors.
- Probability: The likelihood that a generalization will hold true based on the observed patterns, recognizing that conclusions remain subject to new findings.
The accuracy of inductive reasoning depends on the strength of these components. When applied correctly, this approach provides valuable insights that support research, financial assessments, and operational strategies.
Examples of inductive reasoning
Patterns identified through repeated observations allow professionals to make informed predictions across multiple industries. These examples illustrate how inductive reasoning contributes to various fields.
- Scientific research: Multiple clinical trials show that a new drug consistently lowers blood pressure. Researchers conclude that the medication is effective for treating hypertension.
- Market analysis: Sales reports indicate that online promotions increase customer engagement. Analysts predict that additional discounts will generate higher sales.
- Risk assessment: Data from previous economic downturns show increased loan defaults during recessions. Financial institutions anticipate similar trends in future downturns.
- Operational strategy: Production logs reveal that machinery failures increase when maintenance schedules are delayed. Leadership introduces more frequent inspections to prevent disruptions.
Inductive reasoning strengthens the ability to identify patterns, refine predictions, and improve strategic planning. While conclusions may expand with new data, this approach enhances long-term forecasting, allowing professionals to make informed adjustments based on observed trends.
Deductive reasoning vs inductive reasoning
The main difference between deductive reasoning and inductive reasoning lies in the approach used to reach conclusions. Deductive reasoning starts with a general premise and applies it to specific cases, leading to conclusions that are logically certain if the premises hold true. Inductive reasoning, on the other hand, begins with specific observations and identifies patterns to form general conclusions, making it a probability-based approach rather than one grounded in absolute certainty.
Aspect | Deductive Reasoning | Inductive Reasoning |
---|---|---|
Approach | Applies general premises to specific cases | Forms general conclusions from specific observations |
Certainty | Guarantees a true conclusion if premises are correct | Provides a probable conclusion based on observed patterns |
Common Uses | Mathematics, law, formal logic, software verification | Scientific research, market analysis, financial forecasting |
Example | All mammals have lungs. A dog is a mammal. Therefore, a dog has lungs. | A researcher observes that multiple patients benefit from a new drug. The conclusion is that the drug is effective for treating the condition. |
Flexibility | Fixed conclusions that remain true if logic is valid | Adaptable conclusions that change with new data |
Reliability | High when premises are correct | Dependent on the quality and consistency of observed patterns |
Both methods serve distinct purposes across industries that require logical assessments. Deductive reasoning provides structured conclusions that remain valid when premises hold, making it useful for legal analysis, mathematical proofs, and software development. Inductive reasoning allows professionals to refine strategies based on observed trends, helping scientists develop theories, businesses anticipate consumer behavior, and financial analysts assess investment risks. Applying the correct method depends on the need for certainty or adaptability in concluding.
Abductive reasoning vs deductive reasoning
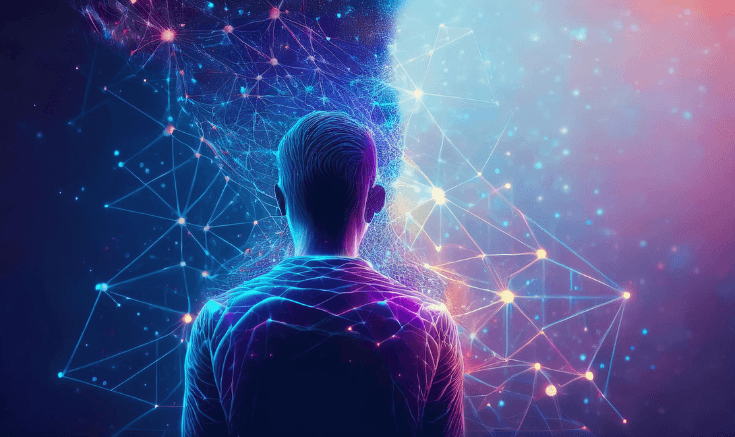
Logical reasoning follows different paths depending on the certainty of available information. Deductive reasoning applies general premises to specific cases, guaranteeing a true conclusion when the initial premises are accurate. Abductive reasoning takes a different approach by forming the most likely explanation based on limited information. While deductive reasoning focuses on logical certainty, abductive reasoning is used when data is incomplete, making it essential for diagnosing medical conditions, conducting investigations, and developing scientific hypotheses. Professionals in different fields rely on these reasoning methods to analyze information, draw conclusions, and refine strategies based on available evidence.
Deductive reasoning provides a structured approach where conclusions follow a step-by-step logical framework. This method plays a critical role in mathematics, legal analysis, and technical problem-solving, where the accuracy of premises determines the conclusion's validity. Abductive reasoning, on the other hand, focuses on probability rather than certainty, making it valuable in scenarios where limited evidence requires an educated assumption. A doctor diagnosing an illness based on symptoms, a detective identifying a suspect based on scattered clues, or a scientist forming an initial hypothesis based on partial data all rely on abductive reasoning to reach the most plausible conclusion. Since this reasoning method involves inference, conclusions may require adjustments as additional information becomes available.
Logical certainty in deductive reasoning makes it a reliable tool in disciplines that require definitive outcomes. Once a deductive conclusion is reached, no further evidence is needed to verify its accuracy, provided the premises hold. Abductive reasoning, however, requires a degree of flexibility, allowing professionals to refine their conclusions as new information is gathered. While these two reasoning methods differ in approach, both play essential roles in analysis, problem-solving, and critical thinking. Deductive reasoning eliminates ambiguity through structured logic, while abductive reasoning helps professionals make timely assessments based on the best available evidence.
Inductive reasoning vs abductive reasoning
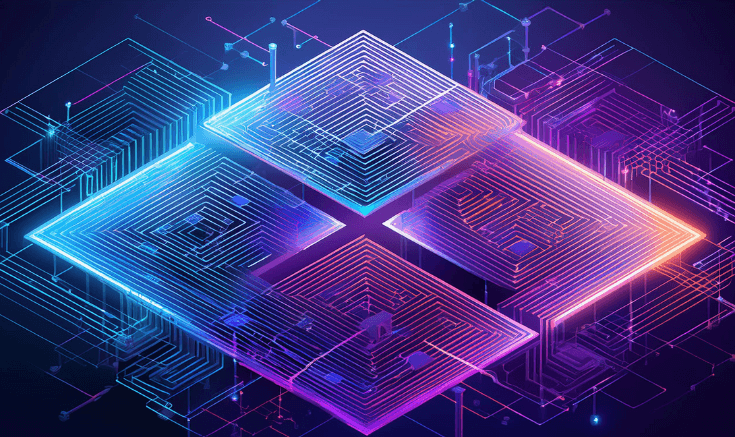
Logical reasoning plays a critical role in forming conclusions, but different approaches serve distinct purposes based on the available information and the desired outcome. Inductive reasoning builds general principles by analyzing repeated observations, making it valuable for trend analysis, predictive modeling, and statistical assessments. Abductive reasoning, on the other hand, focuses on forming the most plausible explanation given incomplete information. This method plays a key role in fields that require immediate assumptions based on limited data, such as medical diagnosis, investigative work, and troubleshooting technical issues. While both reasoning methods help professionals interpret information, their applications and reliability differ based on how conclusions are formed.
Inductive reasoning relies on pattern recognition and statistical probability to develop broader generalizations. When researchers observe a consistent outcome across multiple studies, conclusions are drawn based on the assumption that the pattern will continue under similar conditions. Market analysts track consumer behaviors over time to predict future trends, while risk assessors evaluate historical data to anticipate potential financial fluctuations. Since this reasoning method depends on probability rather than absolute certainty, conclusions may shift as additional data becomes available. This flexibility allows professionals to refine insights over time, making inductive reasoning an essential tool in industries that depend on forecasting and trend analysis.
Abductive reasoning focuses on inference and hypothesis formation when evidence is incomplete. Medical professionals diagnose conditions based on symptoms before test results confirm the cause, engineers troubleshoot system failures based on observable malfunctions, and detectives build cases around the most logical suspect given the available clues. Unlike inductive reasoning, which looks at repeated patterns, abductive reasoning forms conclusions based on the best possible explanation for a single event. While this method allows for faster decision-making in situations where immediate findings are necessary, the accuracy of the outcome depends on the quality of the available data.
Both reasoning methods provide valuable insights, but they serve different objectives. Inductive reasoning builds conclusions by analyzing patterns over time, making it useful for predictions and strategic planning. Abductive reasoning focuses on forming an immediate, probable explanation when data is incomplete, making it ideal for quick assessments. Selecting the appropriate reasoning method depends on the problem's nature, the available information's reliability, and the level of certainty required for making an informed conclusion.
Inductive vs deductive vs abductive reasoning
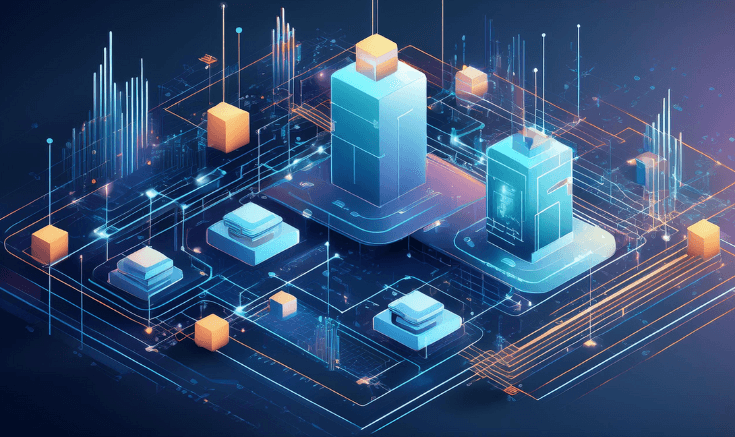
The main difference between inductive, deductive, and abductive reasoning lies in how conclusions are formed and the level of certainty each method provides. Deductive reasoning starts with a general premise and applies it to specific cases, leading to findings that hold true if the premises are accurate. Inductive reasoning begins with specific observations and identifies patterns to form general conclusions, relying on probability rather than certainty. Abductive reasoning seeks the most plausible explanation based on incomplete information, making it useful when all necessary facts are unavailable.
Aspect | Deductive Reasoning | Inductive Reasoning | Abductive Reasoning |
---|---|---|---|
Approach | Applies general premises to specific cases | Forms general conclusions from specific observations | Determines the most plausible explanation based on incomplete information |
Certainty | Guarantees a true conclusion if premises are correct | Provides a probable conclusion based on observed patterns | Offers a likely explanation but lacks guaranteed certainty |
Common Uses | Mathematics, legal analysis, computer programming | Scientific research, market analysis, financial forecasting | Medical diagnosis, criminal investigations, hypothesis formation |
Example | All mammals have lungs. A cat is a mammal. Therefore, a cat has lungs. | Multiple patients benefit from a new medication. The conclusion is that the medication is effective for treating the condition. | A doctor observes fever and a persistent cough. The most likely explanation is a respiratory infection. |
Flexibility | Fixed conclusions based on logical structure | Adaptable conclusions that change with new data | Conclusions may shift as additional evidence emerges |
Reliability | High when premises are correct | Dependent on the consistency and quality of observations | Accuracy depends on the strength of available evidence |
Each reasoning method plays a distinct role in logical analysis. Deductive reasoning guarantees logical accuracy when applied correctly, making it ideal for fields that require definitive conclusions. Inductive reasoning allows professionals to refine predictions and strategic models by analyzing patterns and trends. Abductive reasoning provides a way to make informed assessments when limited data is available, helping experts form the best possible explanations under uncertain conditions. Selecting the appropriate approach depends on the information at hand, the required level of certainty, and the specific objectives of the analysis.
Logical reasoning supports stronger conclusions, better analysis, and more effective problem-solving across industries. Choosing the right reasoning method allows professionals to make confident assessments and informed strategic choices. At Lumenalta, we deliver solutions that align with business objectives, equipping organizations with the insights needed to lead. Let’s chart a brighter path together.
table-of-contents
Common questions about deductive reasoning vs inductive reasoning
What is the difference between inductive and deductive reasoning?
How does abductive reasoning differ from deductive reasoning?
Why is inductive reasoning important in research and forecasting?
What are some examples of abductive reasoning in real-world applications?
Which type of reasoning is the most reliable?
Want to learn how digital transformation can bring more transparency and trust to your operations?