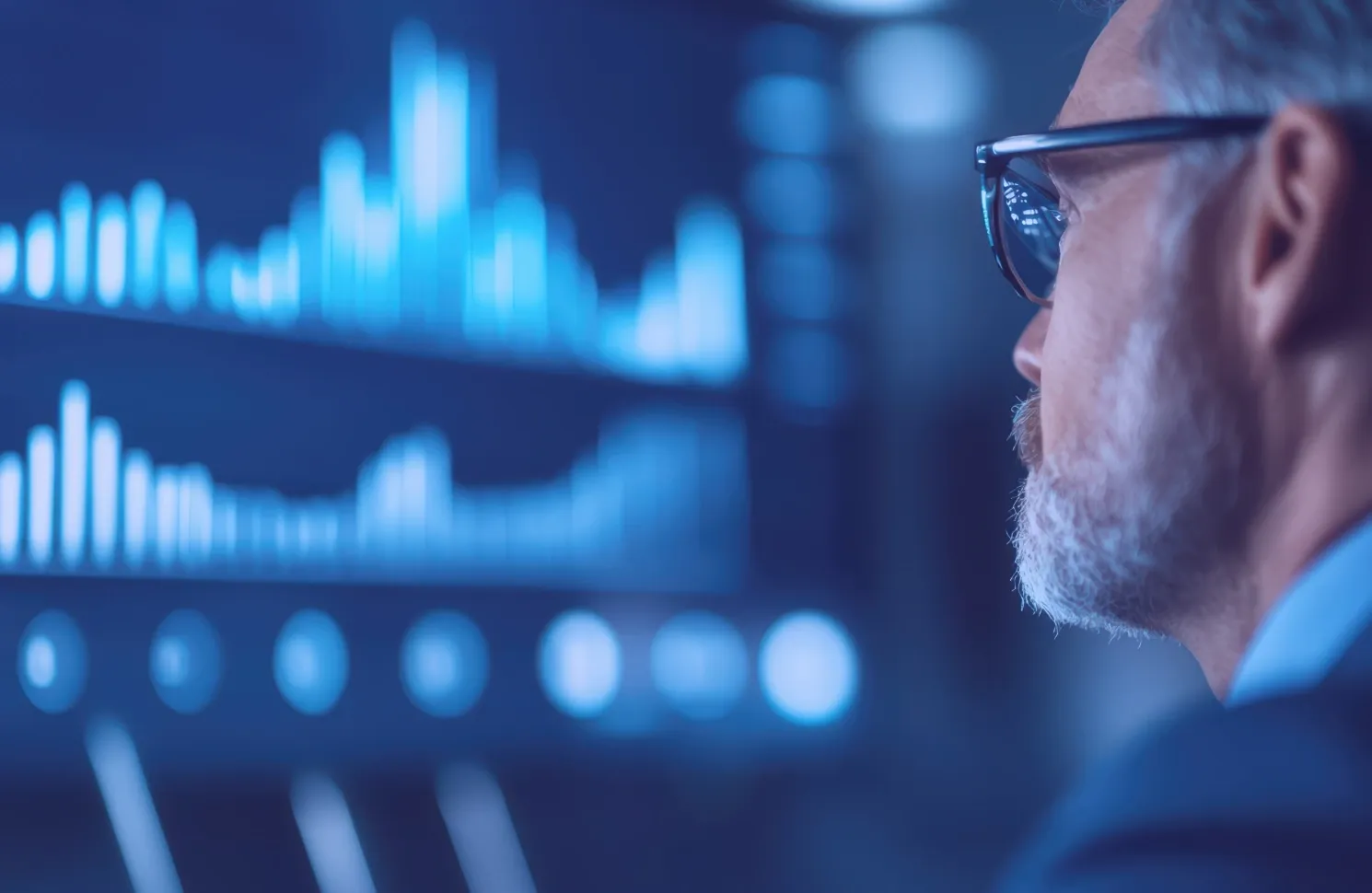
The CFO's data mandate: The end of unlimited data budgets
APR. 24, 2025
2 Min Read
AI is live. Budgets are tight. The era of “store everything” is over—data must prove its value.
For years, “just store it” was the default data strategy. Teams assumed every log line and every transaction might prove valuable someday, especially with AI (artificial intelligence) looming on the horizon. It was easier to over-collect than risk under-preparing. And for a long time, there was enough budgetary slack to make that behavior sustainable.
But now that AI is live in production and touching real parts of the business, that logic no longer holds up. Leaders don’t want to know what data you might need someday. They want to know what’s actually delivering value now.
What used to be “keep it all, just in case” has turned into “prove it’s worth it.” Every dollar spent on storage, compute, and data engineering is getting a closer look. The mandate from leadership is clear: keep only what matters, and make sure your infrastructure supports real outcomes.
How enterprise data platforms got bloated in the first place
This problem didn’t appear overnight. It accumulated slowly over time due to the fear of deleting the wrong dataset or missing out on a future use case. What started as caution quickly calcified into clutter.
At the same time, it became easier than ever to add new tools, spin up environments, and layer on services. The cost of saying “yes” to more data was hidden behind a layer of abstraction, and no one was responsible for the full picture. Platform teams managed pipelines. Finance managed budgets. But cleanup? That belonged to no one.
The result is what most companies are dealing with now: a tangled ecosystem of overlapping tools, idle workloads, and data that hasn’t been touched in months but still costs money to store.
Where costs hide and how to surface them
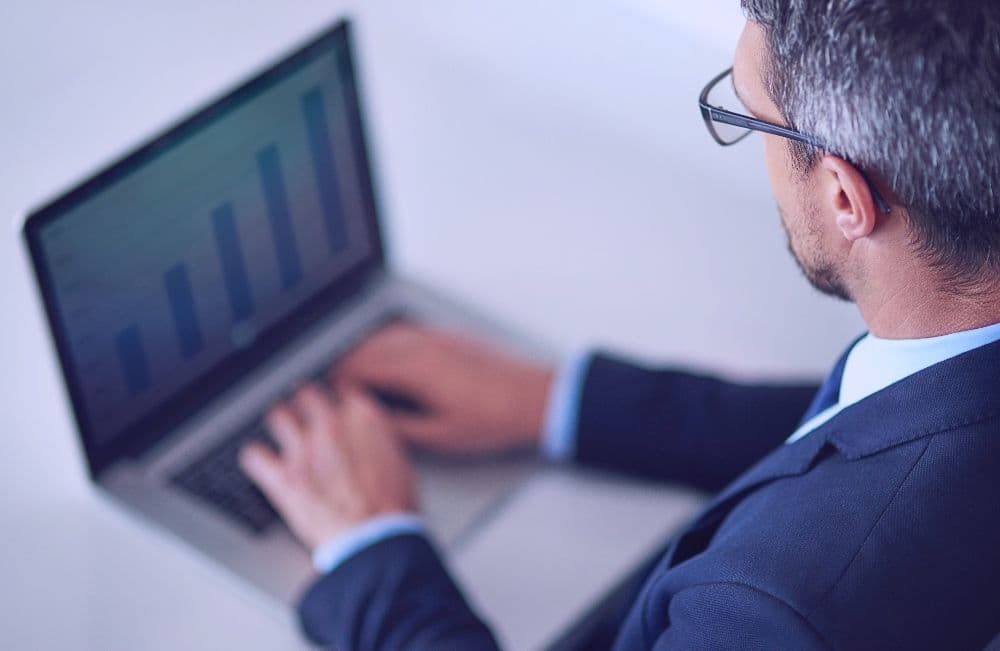
- Pipelines run each night against stale data
- BI dashboards auto-refreshing whether or not anyone checks them
- Tables are getting copied and rebuilt across environments because no one wants to break what’s already working
All of it quietly burns through compute and engineering hours, and most of it goes unnoticed.
Redundancy is part of the problem. A table gets rebuilt in two different pipelines. Two teams ship similar dashboards using the same inputs. Everyone’s solving the same problem in slightly different ways, but no one’s connecting the dots.
Without a shared understanding of who’s responsible for what—and whether it still matters—systems grow more expensive by the week.
You don’t need to rip out infrastructure to fix this. You need visibility. Start by mapping usage: who’s actually using which assets, how often, and to what end. When teams can trace cost to outcome, decisions get sharper. Dead jobs get retired. Duplicates get merged.
Value becomes easier to prove, and waste becomes easier to cut.
How leading teams are responding
The shift from “store it all” to “prove it’s worth it” doesn’t start with a new tool or framework. It starts with a simple question from finance: “What are we actually getting for all this?”
That question changes how data teams think about value. It’s no longer just about performance benchmarks or how many assets you’ve built. It’s about whether those assets are being used and what they’re enabling. A fast dashboard that no one opens doesn’t matter. The new focus is on usage, not volume.
Rather than simply cutting costs, teams that are adapting well are measuring them with more granularity: cost per query, cost per workflow, cost per insight. It’s a way to tie infrastructure back to outcomes instead of just tracking it as overhead.
And that’s changing how platforms are built. Instead of adding more layers, teams are tightening what’s already there. They’re improving metadata, adding cost attribution, and enforcing policies that help data stay useful, not just available.
The days of infinite data budgets are over, and that’s a good thing for all involved. It means less clutter, more clarity, and a stronger connection between investment and impact.
Audit your data to cut the clutter and keep the value.