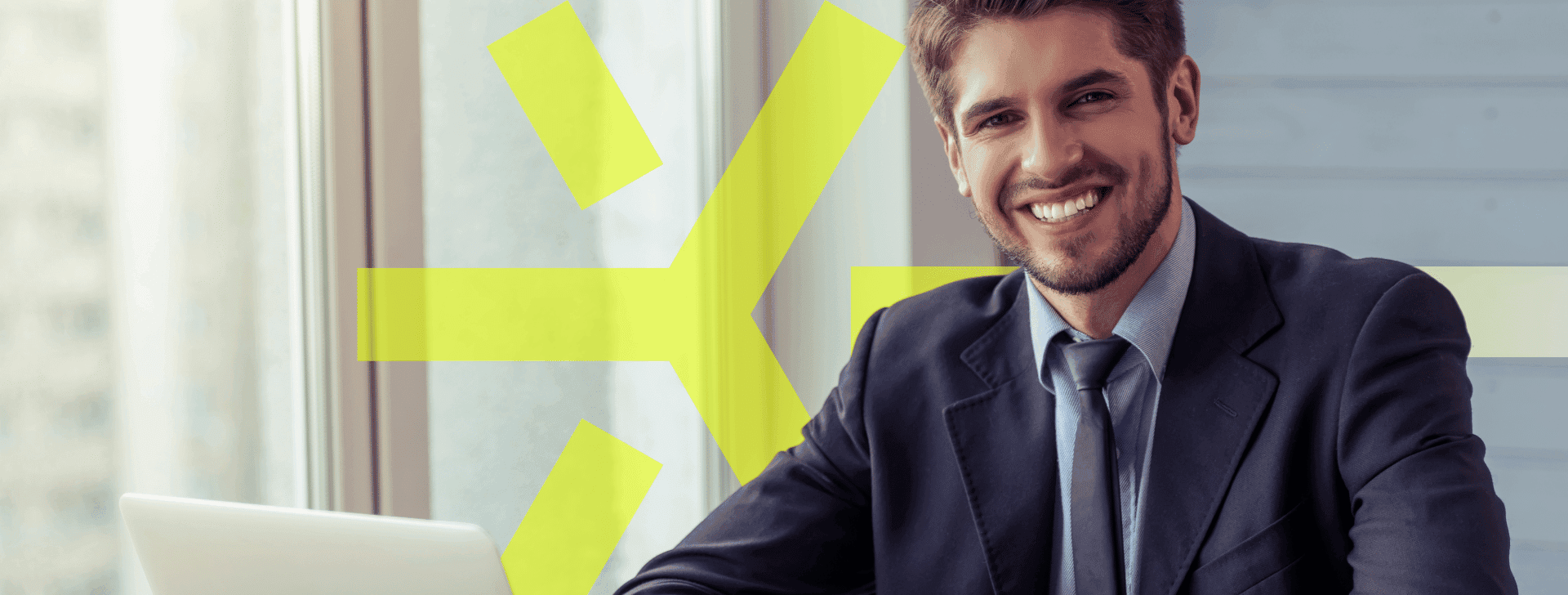
Fuel BFSI approvals and profitability through AI-powered transaction monitoring
MAY. 5, 2025
7 Min Read
Banks have traditionally viewed AI-powered transaction monitoring as a compliance necessity, essentially a cost center to meet regulations like AML and fraud prevention.
Forward-thinking institutions, however, are realizing that the same AI oversight can be a growth driver when implemented holistically. By shifting from passive monitoring to AI-driven approvals, banks can not only catch bad actors but also accelerate legitimate transactions, improving customer experience and revenue. The key is to embed AI monitoring into a broader digital transformation strategy, rather than treating it as an isolated tool.
Key takeaways
- 1. Real-time fraud detection: AI-powered transaction monitoring analyzes transactions in real time to catch suspicious activities that humans might miss, strengthening fraud prevention and AML compliance.
- 2. Fewer false alarms: By dramatically cutting false positives, AI allows banks to approve more legitimate transactions faster, improving customer experience and throughput.
- 3. Efficiency and cost savings: Automated AI monitoring reduces manual workloads, lowering compliance costs and freeing staff to focus on high-value investigations instead of reviewing false alerts.
- 4. Faster, smarter decisions: AI-driven analytics accelerate decision-making and ensure consistent, unbiased risk assessment—crucial benefits for digital transformation in banking.
- 5. Higher profitability: AI in BFSI prevents fraud losses, streamlines processes, and gives institutions a competitive edge, directly boosting the bottom line.
From compliance tool to customer-centric growth
For many banks, transaction monitoring has been about flagging suspicious transactions after the fact – a reactive, check-the-box compliance activity. This passive approach can frustrate customers (e.g. when their legitimate transactions get delayed or declined) and offers little business value. In contrast, AI-driven monitoring and approvals work in real time to analyze transactions, distinguish normal customer behavior from truly suspicious activity, and proactively approve legitimate transactions faster. This reduces friction for customers and keeps revenue flowing.
One striking example is in payment processing: false declines (legitimate transactions wrongly blocked by fraud controls) cost e-commerce merchants an estimated $443 billion in 2021, vastly more than the $40 billion lost to actual fraud. In other words, overly cautious systems are turning away good business – and banks, as card issuers and payment facilitators, share in that lost revenue. AI models that better discern fraud can minimize these false positives, boosting approval rates and sales. Fintechs using AI-driven transaction monitoring report up to 80% reductions in payment failures and significantly improved approval rates. By moving from a purely defensive stance to an enablement mindset, financial institutions turn compliance into an opportunity: fewer unnecessary blocks mean happier customers and more completed transactions.
“By drastically reducing false positives, AI-driven monitoring frees compliance teams to focus on real risks, enabling faster legitimate approvals and a healthier bottom line.”
High-value benefits beyond compliance
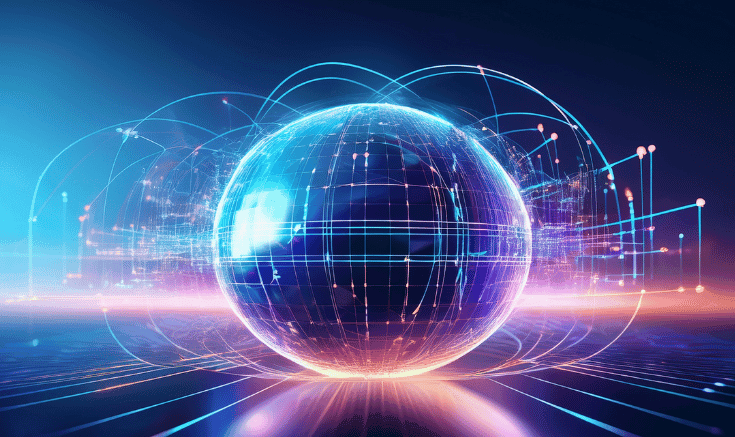
Integrating AI-powered monitoring into the core of BFSI operations yields tangible benefits across the board. Business and operational leaders should consider the following high-value gains:
- Cost reductions: Automating compliance and fraud checks with AI cuts down manual review workloads and errors. North American banks spent $56.7 billion on financial crime compliance in 2022 – a huge cost burden that AI can help trim through efficiency. Machine learning models can sift through vast transaction data faster than staff, reducing labor hours and expensive false alarms. For instance, AI-driven systems have reduced false positive alerts by over 90% in some cases, freeing compliance teams to focus on real risks.
- New revenue streams: Better monitoring insights can uncover customer behaviors and unmet needs. By breaking out of siloed rule-based systems, banks can analyze transaction patterns to personalize offers (within ethical guardrails) – turning data into cross-sell and up-sell opportunities. More immediately, higher approval rates on transactions mean more fee revenue. Every approved payment or loan that would have been erroneously flagged before is now a saved sale. In retail banking, improving customer experience correlates directly with growth – studies show enhanced customer experience can drive 27.5% revenue growth in three years. AI helps deliver that experience by making banking smoother and more responsive.
Reduced risk (with better customer experience): It may sound paradoxical, but tightening risk controls with AI actually reduces risk and improves CX at the same time. AI models can detect fraud and money laundering patterns far more accurately than legacy rules, catching illicit activity that might slip through and preventing costly regulatory fines or losses. At the same time, AI’s accuracy means fewer “false alarms” that inconvenience good customers with account freezes or extra documentation requests. The result is a safer institution and a more trusted, convenient service for clients. With real-time anomaly detection, banks can stop fraud in its tracks without halting legitimate transactions – a win-win for risk and relationship managers alike.
Breaking down silos for holistic AI impact
Achieving these benefits requires more than just plugging in an AI tool – it demands a holistic strategy and breaking down organizational silos. In many financial institutions, compliance, fraud, IT, and business units operate in isolation. Siloed systems and data not only create inefficiency, they also “block a holistic view” of both the institution and the customer. An AI-powered monitoring solution confined to the compliance department will never reach its full potential if its insights aren’t shared with product teams, customer service, and leadership.
Cross-functional collaboration is essential. Forward-looking banks are creating unified data environments where transaction monitoring AI feeds into customer profiles, and vice versa. For example, if the AI flags unusual, but not illicit, customer behavior (say an overseas transaction pattern), the compliance team can inform customer service to proactively reach out and verify, rather than simply shutting down the account. Conversely, customer onboarding data and profile updates should flow into the AI models to continuously refine what “normal” behavior looks like for each individual. Breaking barriers between departments allows the organization to leverage AI insights enterprise-wide – delivering both stronger risk controls and more personalized customer experiences. Leading FIs find that dissolving these silos “opens new ways of understanding and engaging with customers” and helps deliver the superior experiences modern consumers expect
Technology integration is part of this equation as well. Banks often juggle multiple legacy platforms; moving to a unified, cloud-based, or API-integrated architecture ensures your AI monitoring system can pull data from all relevant sources (core banking, mobile apps, CRM, etc.) and push out alerts or approvals to all the right channels in real time. An enterprise-wide decisioning layer that centralizes rules and AI models can coordinate decisions across product lines – so a fraud alert in one division informs others, or a customer’s risk score is consistently applied whether they’re applying for a credit card or transferring funds. In short, make AI a shared capability across the institution, not an isolated project.
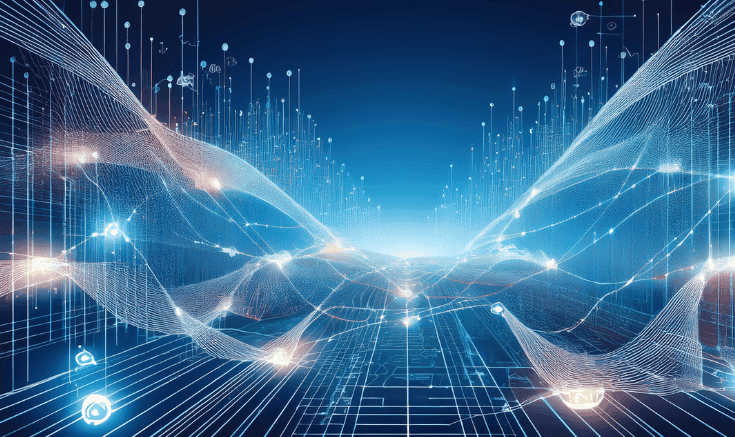
Tracking gains and adapting strategies
To truly fuel profitability, AI-powered transaction monitoring must be approached as an ongoing program with measurable outcomes. This means establishing clear metrics and feedback loops from the start. Banks should define KPIs that capture both compliance efficiency and customer experience improvements. Examples include:
- Alert accuracy: Track reduction in false positives (e.g. % decrease in alerts that are cleared as non-issues). A dropping false positive rate directly translates to saved investigation costs and less customer friction.
- Approval/Conversion rate: Measure the percentage of legitimate transactions or applications approved immediately. As AI models refine, you should see a higher share of transactions processed straight-through without manual review, boosting revenue.
- Case handling time: Monitor how quickly flagged issues are resolved. If AI effectively prioritizes truly risky alerts, investigators can close cases faster. Quicker resolutions mean less operational drag and a smoother experience for customers who may be waiting.
- Customer impact: Keep an eye on customer experience indicators – complaint volumes related to “blocked transactions” or account lockouts, customer satisfaction scores, churn rates, etc. Improvement here signals that AI oversight is enhancing the customer journey, not just tightening control.
Regularly review these metrics and be ready to adapt your strategy. AI models learn over time, and so should your processes. For instance, if certain rules are still causing too many false alerts, feed that feedback to your data science team to retune the model. If new fraud patterns emerge (as they inevitably will), update your algorithms and training data promptly. Some banks hold quarterly cross-department meetings to assess the AI program’s results – discussing what’s working, what needs adjustment, and where to invest next. This iterative approach ensures the impact is not only achieved but sustained. The institutions that get the most value from AI in transaction monitoring treat it as a living system, continually improving detection rates and alignment with business goals.
“AI-powered transaction monitoring transforms compliance from a regulatory burden into a strategic asset—fueling faster approvals, lower costs, and greater profitability in BFSI.”
Sustaining momentum: Training, governance, and flexibility
Implementing AI-powered monitoring is not a one-time project – it’s a transformational journey. To maintain momentum and long-term impact, banks should focus on a few actionable strategies:
- Invest in training and culture: The best AI tools won’t deliver value if teams don’t trust or know how to use them. Train your compliance analysts, risk officers, and even front-line staff on the new AI system’s capabilities and outputs. Help them understand why the AI flags something or approves something (providing explainability where possible). This builds trust in the AI and encourages teams to embrace new workflows rather than revert to old habits. Moreover, cultivate a culture where compliance and business teams work together – for example, relationship managers should understand compliance pain points, and compliance staff should appreciate the customer impact of their decisions. A shared vision of “compliance + customer experience” will keep everyone invested in the AI’s success.
- Establish strong governance: With great power (AI automation) comes great responsibility. Create a governance framework to oversee the AI’s performance, fairness, and alignment with regulations. This might include an AI ethics committee or regular audits of the model’s decisions to ensure no unintentional bias or compliance gaps. Governance ensures that even as AI takes on more decision-making, human oversight remains in place to catch errors and continuously tune the system. It also helps in documenting decisions and changes – critical for regulator confidence. Remember, regulators are increasingly interested in AI explainability and controls, so proactive governance not only manages risk but also reassures examiners that your bank’s AI is under control.
- Adopt flexible implementation: Lastly, avoid rigid, monolithic deployments. Aim for modular AI solutions that can plug into various systems and be updated or expanded easily. Financial crimes and customer behaviors evolve rapidly; your AI platform should allow quick integration of new data sources, tweaking of models, or addition of new use cases (such as extending from AML monitoring into anti-fraud, credit risk, or even marketing analytics). Cloud-based AI services or microservices architectures can provide this flexibility. The goal is to future-proof your investment – ensuring the AI can adapt as business needs change, new regulations come in, or as you scale to new markets. Flexibility also means measuring and scaling: start with a pilot in one area, prove the ROI, then expand to other lines of business with lessons learned.
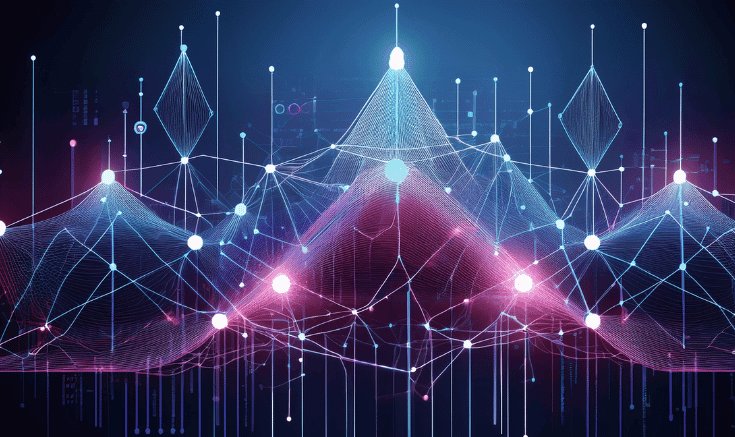
AI-powered monitoring thrives when integrated into a bank’s entire ecosystem. Breaking down silos – both technical and organizational – is crucial. The illustration above symbolizes collaborative digital transformation: multiple hands working in unison on a connected platform. By unifying efforts across compliance, IT, and business units, banks create a support system for AI to deliver its full value. This kind of teamwork and integration is what turns an AI tool into a catalyst for enterprise-wide growth.
In the BFSI sector, effective oversight and customer experience have often been seen as opposing forces – tighten controls and you inevitably slow things down for customers. AI-powered transaction monitoring is turning that old assumption on its head. When implemented as part of a strategic digital transformation (not just a standalone compliance module), AI monitoring can fuel approvals, streamline operations, and elevate the customer experience simultaneously. The result is not only fewer losses and compliance violations but also tangible upticks in revenue and efficiency. Banks that embrace this approach are essentially monetizing compliance: using cutting-edge AI to satisfy regulators and delight customers in one stroke.
The message for decision-makers is clear. Don’t relegate AI to the back office for checkbox compliance. Bring it front and center into your products and customer journeys. Tear down the walls between risk and business teams so that insights flow freely. Track your successes, learn from the data, and refine continuously. And above all, keep your people engaged and accountable through training and governance, so the technology remains aligned with your goals. By doing so, your institution can turn transaction monitoring from a cost of doing business into a driver of new business – achieving oversight and growth, hand in hand. The banks and insurers that get this right will not only avoid the pitfalls of the past but also set new benchmarks for what proactive, AI-enabled financial services can achieve in profitability and customer loyalty.
At Lumenalta, we specialize in transforming cutting-edge technologies like AI-powered transaction monitoring into practical, bottom-line results for BFSI organizations. Our approach to digital transformation is rooted in measurable outcomes and business pragmatism—focusing on solutions that accelerate approvals, strengthen compliance, and drive profitability.
Common questions about big data
What is AI-powered transaction monitoring in banking?
How does AI-powered transaction monitoring boost profitability in BFSI?
How does AI in BFSI reduce false positives in fraud detection?
What role does AI play in digital transformation in banking?
How can financial institutions implement AI-powered transaction monitoring effectively?
Want to learn how AI in BFSI can bring more transparency and trust to your operations?